Reckoning with the Disagreement Problem: Explanation Consensus as a Training Objective
PROCEEDINGS OF THE 2023 AAAI/ACM CONFERENCE ON AI, ETHICS, AND SOCIETY, AIES 2023(2023)
摘要
As neural networks increasingly make critical decisions in high-stakes settings, monitoring and explaining their behavior in an understandable and trustworthy manner is a necessity. One commonly used type of explainer is post hoc feature attribution, a family of methods for giving each feature in an input a score corresponding to its influence on a model's output. A major limitation of this family of explainers in practice is that they can disagree on which features are more important than others. Our contribution in this paper is a method of training models with this disagreement problem in mind. We do this by introducing a Post hoc Explainer Agreement Regularization (PEAR) loss term alongside the standard term corresponding to accuracy, an additional term that measures the difference in feature attribution between a pair of explainers. We observe on three datasets that we can train a model with this loss term to improve explanation consensus on unseen data, and see improved consensus between explainers other than those used in the loss term. We examine the trade-off between improved consensus and model performance. And finally, we study the influence our method has on feature attribution explanations.
更多查看译文
关键词
explanation consensus,disagreement problem,objective,training
AI 理解论文
溯源树
样例
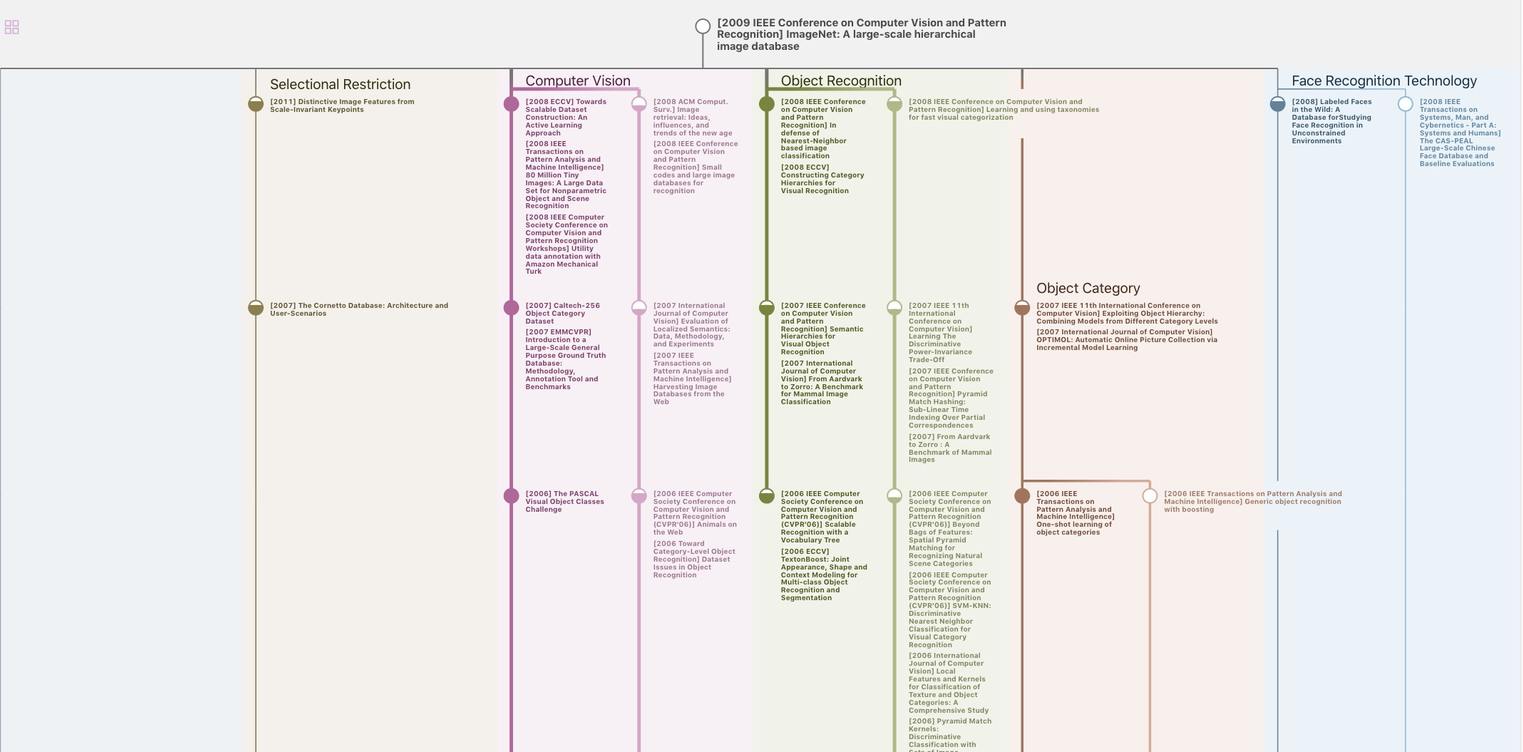
生成溯源树,研究论文发展脉络
Chat Paper
正在生成论文摘要