Uncovering the Missing Pattern: Unified Framework Towards Trajectory Imputation and Prediction.
CVPR 2023(2023)
摘要
Trajectory prediction is a crucial undertaking in understanding entity movement or human behavior from observed sequences. However, current methods often assume that the observed sequences are complete while ignoring the potential for missing values caused by object occlusion, scope limitation, sensor failure, etc. This limitation inevitably hinders the accuracy of trajectory prediction. To address this issue, our paper presents a unified framework, the Graph-based Conditional Variational Recurrent Neural Network (GC-VRNN), which can perform trajectory imputation and prediction simultaneously. Specifically, we introduce a novel Multi-Space Graph Neural Network (MS-GNN) that can extract spatial features from incomplete observations and leverage missing patterns. Additionally, we employ a Conditional VRNN with a specifically designed Temporal Decay (TD) module to capture temporal dependencies and temporal missing patterns in incomplete trajectories. The inclusion of the TD module allows for valuable information to be conveyed through the temporal flow. We also curate and benchmark three practical datasets for the joint problem of trajectory imputation and prediction. Extensive experiments verify the exceptional performance of our proposed method. As far as we know, this is the first work to address the lack of benchmarks and techniques for trajectory imputation and prediction in a unified manner.
更多查看译文
关键词
Video: Low-level analysis,motion,and tracking
AI 理解论文
溯源树
样例
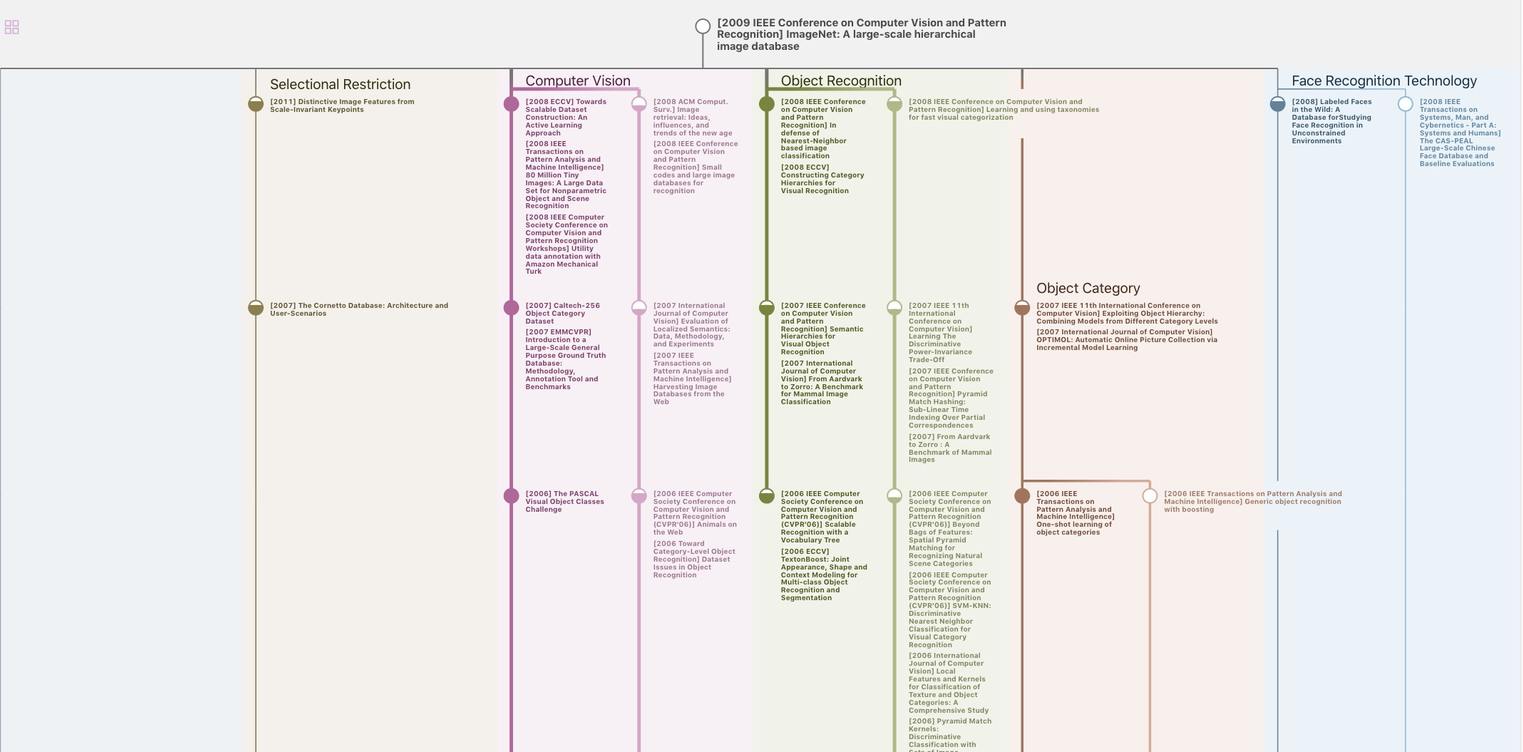
生成溯源树,研究论文发展脉络
Chat Paper
正在生成论文摘要