Token Merging for Fast Stable Diffusion
CoRR(2023)
摘要
The landscape of image generation has been forever changed by open vocabulary diffusion models. However, at their core these models use transformers, which makes generation slow. Better implementations to increase the throughput of these transformers have emerged, but they still evaluate the entire model. In this paper, we instead speed up diffusion models by exploiting natural redundancy in generated images by merging redundant tokens. After making some diffusion-specific improvements to Token Merging (ToMe), our ToMe for Stable Diffusion can reduce the number of tokens in an existing Stable Diffusion model by up to 60% while still producing high quality images with-out any extra training. In the process, we speed up image generation by up to 2× and reduce memory consumption by up to 5.6×. Furthermore, this speed-up stacks with efficient implementations such as xFormers, minimally impacting quality while being up to 5.4× faster for large images. Code is available at https://github.com/dbolya/tomesd.
更多查看译文
关键词
diffusion-specific improvements,high quality images,image generation,natural redundancy,open vocabulary diffusion models,redundant tokens,speed-up stacks,stable diffusion model,token merging,ToMe,transformers,xFormers
AI 理解论文
溯源树
样例
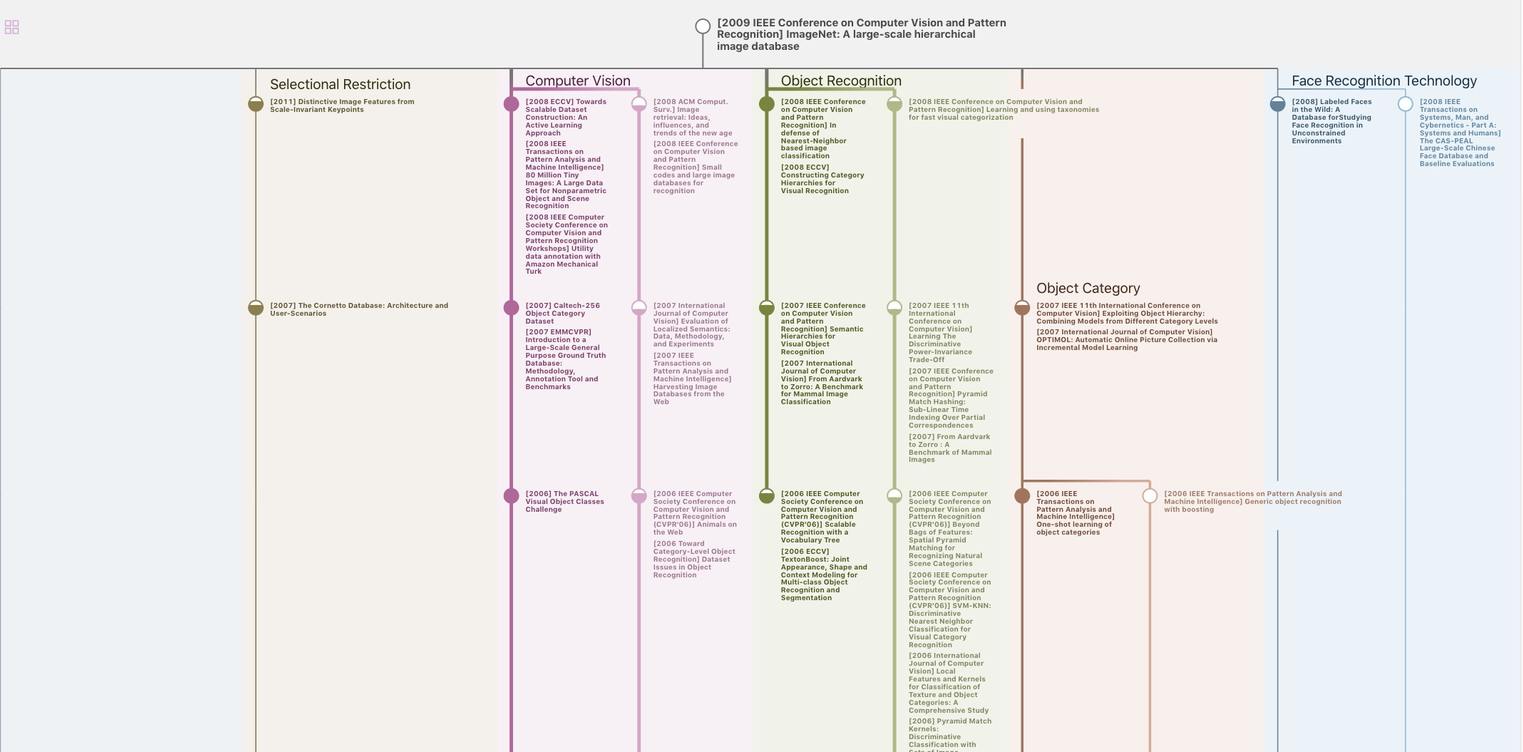
生成溯源树,研究论文发展脉络
Chat Paper
正在生成论文摘要