Batch Size Reconstruction–Distribution Trade–Off in Kernel Based Generative Autoencoders
2022 IEEE International Conference on Image Processing (ICIP)(2022)
摘要
Most autoencoder-based generative machine learning models use a two-factor cost function composed of reconstruction error and prior distribution distance. The latter is often evaluated with kernel–based methods. We notice that the impact of the batch size is different on each of the factors: kernel distribution profits from larger batches, while the reconstruction term achieves peak performance on smaller ones. Thus, we define a batch size reconstruction-distribution trade-off. Instead of searching for an optimum global size of the batch, we propose to use small batches for the sake of reconstruction together with a vector enhanced with previously computed latent data for the sake of prior distribution optimization. We evaluate our method on standard benchmarks and illustrate that it can improve the model’s generative scores.
更多查看译文
关键词
deep learning,autoencoder,generative model,kernel methods
AI 理解论文
溯源树
样例
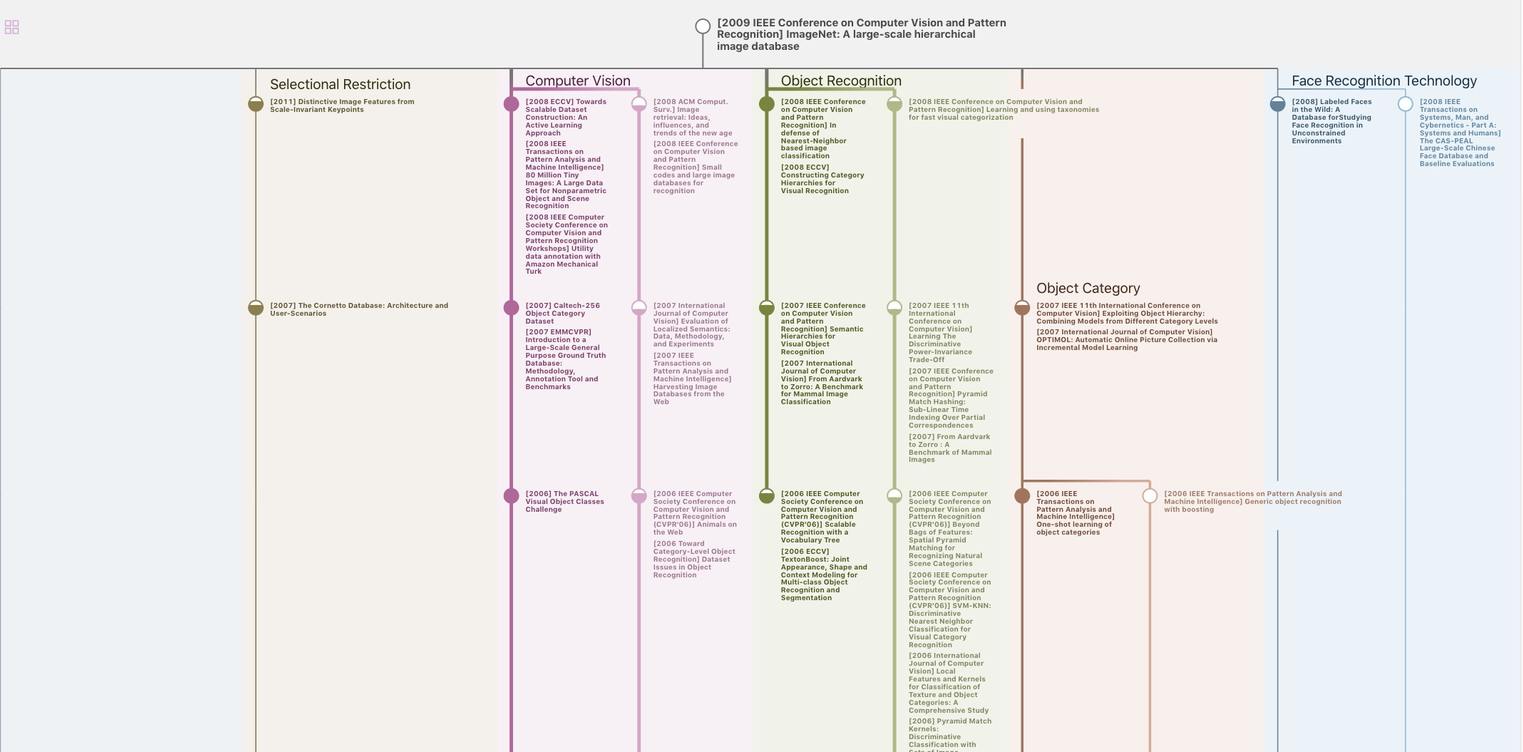
生成溯源树,研究论文发展脉络
Chat Paper
正在生成论文摘要