Concept-Oriented Transformers for Visual Sentiment Analysis.
WSDM(2023)
摘要
In the richly multimedia Web, detecting sentiment signals expressed in images would support multiple applications, e.g., measuring customer satisfaction from online reviews, analyzing trends and opinions from social media. Given an image, visual sentiment analysis aims at recognizing positive or negative sentiment, and occasionally neutral sentiment as well. A nascent yet promising direction is Transformer-based models applied to image data, whereby Vision Transformer (ViT) establishes remarkable performance on large-scale vision benchmarks. In addition to investigating the fitness of ViT for visual sentiment analysis, we further incorporate concept orientation into the self-attention mechanism, which is the core component of Transformer. The proposed model captures the relationships between image features and specific concepts. We conduct extensive experiments on Visual Sentiment Ontology (VSO) and Yelp.com online review datasets, showing that not only does the proposed model significantly improve upon the base model ViT in detecting visual sentiment but it also outperforms previous visual sentiment analysis models with narrowly-defined orientations. Additional analyses yield insightful results and better understanding of the concept-oriented self-attention mechanism.
更多查看译文
AI 理解论文
溯源树
样例
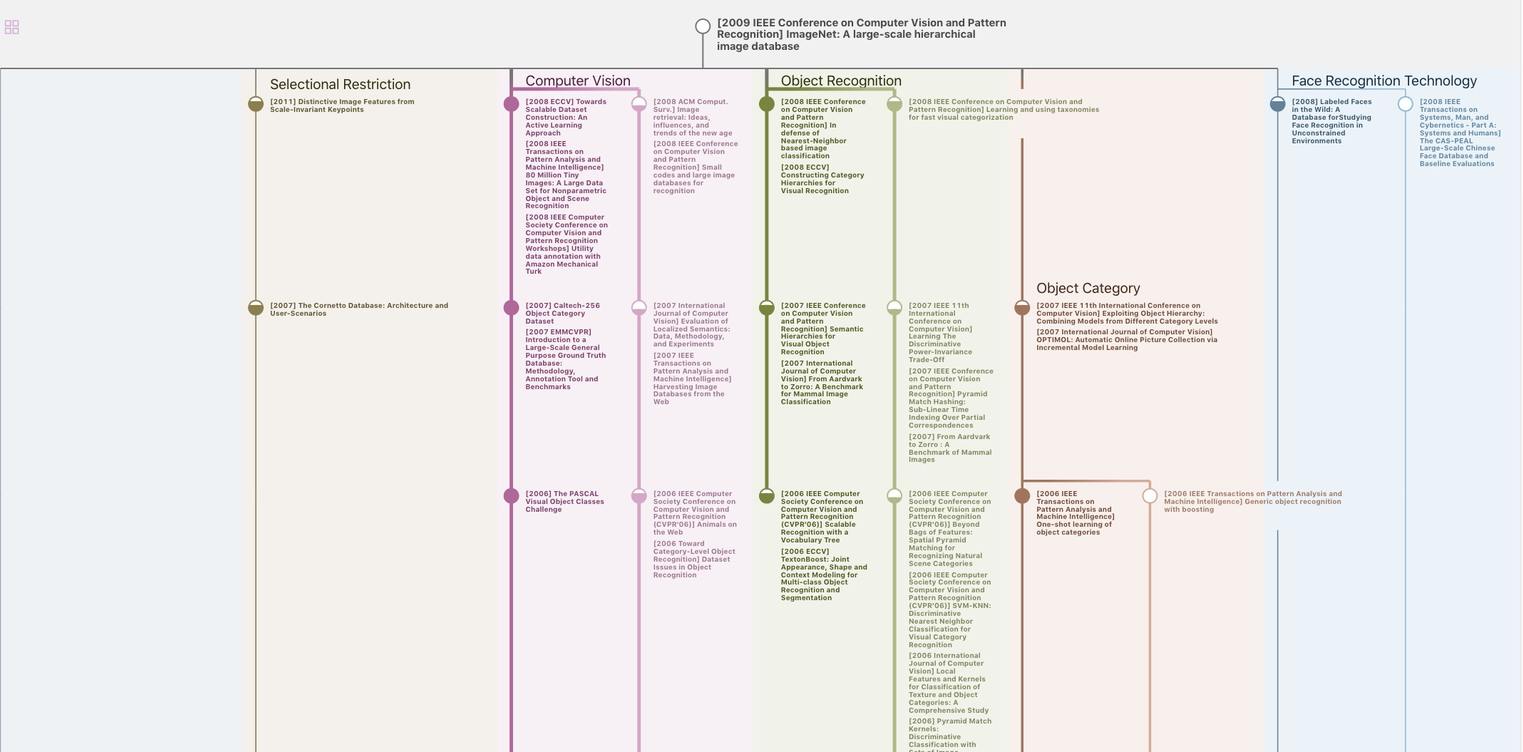
生成溯源树,研究论文发展脉络
Chat Paper
正在生成论文摘要