Risk-aware Analysis for Interpretations of Probabilistic Achievement and Maintenance Commitments
Artificial intelligence(2023)
Abstract
Probabilistic commitments provide a computational framework for multi-agent coordination, where one autonomous agent (the commitment provider), commits to a future course of action that probabilistically influences the local state of another agent (the commitment recipient) in ways that the recipient desires. Conventionally, a probabilistic commitment is specified abstractly so as to give the provider latitude at run time about how to achieve it. Unfortunately, as we analyze in this article, this abstraction incurs a risk of suboptimal performance for the recipient. For (achievement) commitments by the provider to achieve conditions that the recipient prefers but that do not initially hold, we prove that the recipient can make modeling choices that bound its risk of suboptimality. Somewhat surprisingly, however, for (maintenance) commitments by the provider to maintain conditions whose initial values are already ones the recipient prefers, we prove that no such bounds on suboptimality risk are possible. We study the two types of commitments empirically to measure the suboptimality they incur under different conditions, and based on our theoretical and empirical results suggest that adding selective details when specifying probabilistic maintenance commitments can be beneficial.
MoreTranslated text
Key words
Probabilistic commitment,Cooperative multiagent planning,Planning under uncertainty,Achievement commitment,Maintenance commitment
AI Read Science
Must-Reading Tree
Example
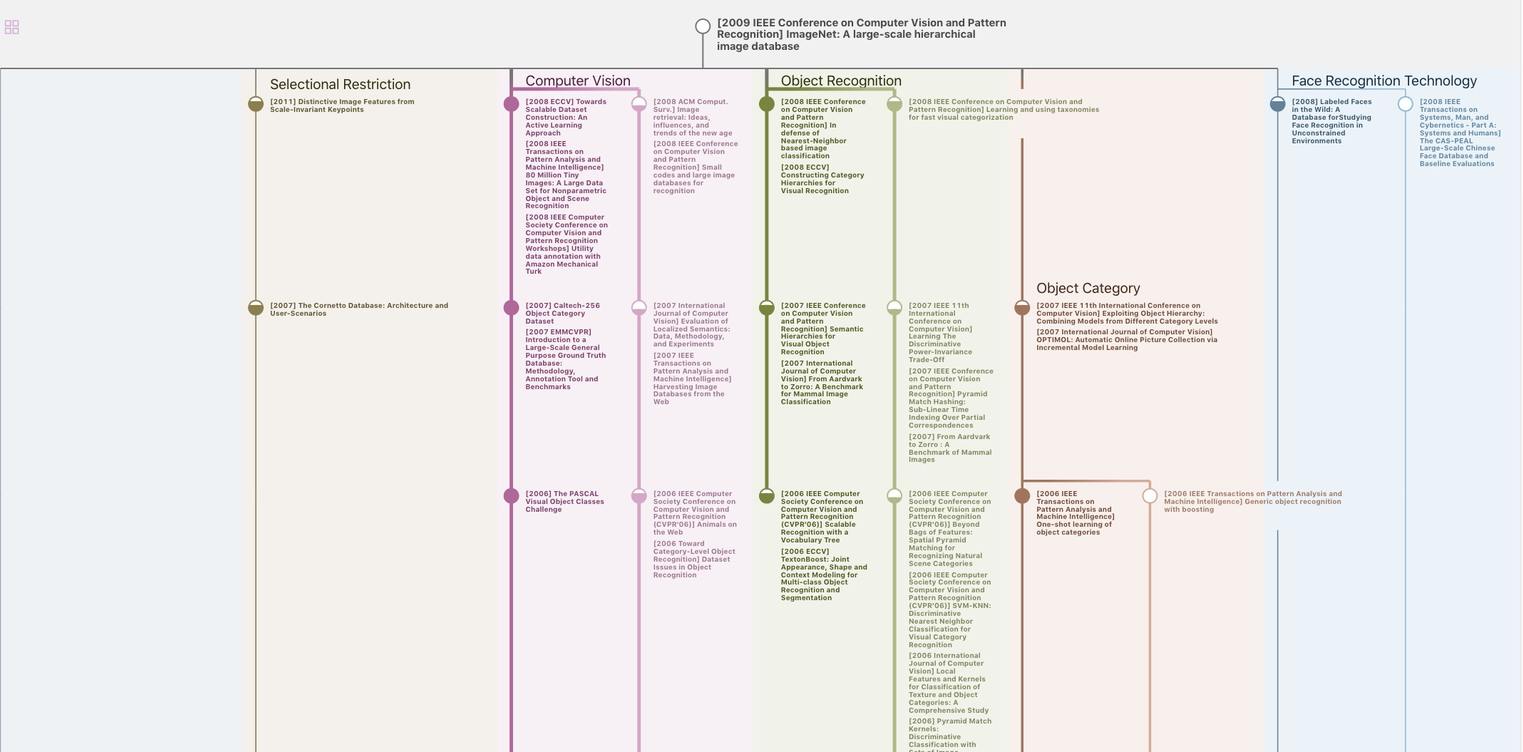
Generate MRT to find the research sequence of this paper
Chat Paper
Summary is being generated by the instructions you defined