Few-Shot Link Prediction with Domain-Agnostic Graph Embedding.
ICMLA(2022)
摘要
Real-world link prediction problems often deal with data from multiple domains, where data may be highly skewed and imbalanced. Computer vision research has studied similar issues under the Few-Shot Learning (FSL) umbrella. However, this problem has rarely been addressed and explored in the graph domain, specifically for link prediction. In this work, we propose an adversarial training-based framework that aims at improving link prediction for highly skewed and imbalanced graphs from different domains by generating domain agnostic embedding. We introduce a domain discriminator on pairs of graph-level embedding. We then use the discriminator to improve the model in an adversarial way, such that the graph embedding generated by the model are domain agnostic. We test our ideas on one large real-world user-business-review dataset and three benchmark datasets. Our results demonstrate that when domain differences exist, our method creates better graph embedding that are more evenly distributed across domains and generate better prediction outcomes. In the absence of domain differences, our method is on par with state-of-the-art.
更多查看译文
关键词
neural networks, link prediction, few-shot learning, graph neural networks, domain adaptation
AI 理解论文
溯源树
样例
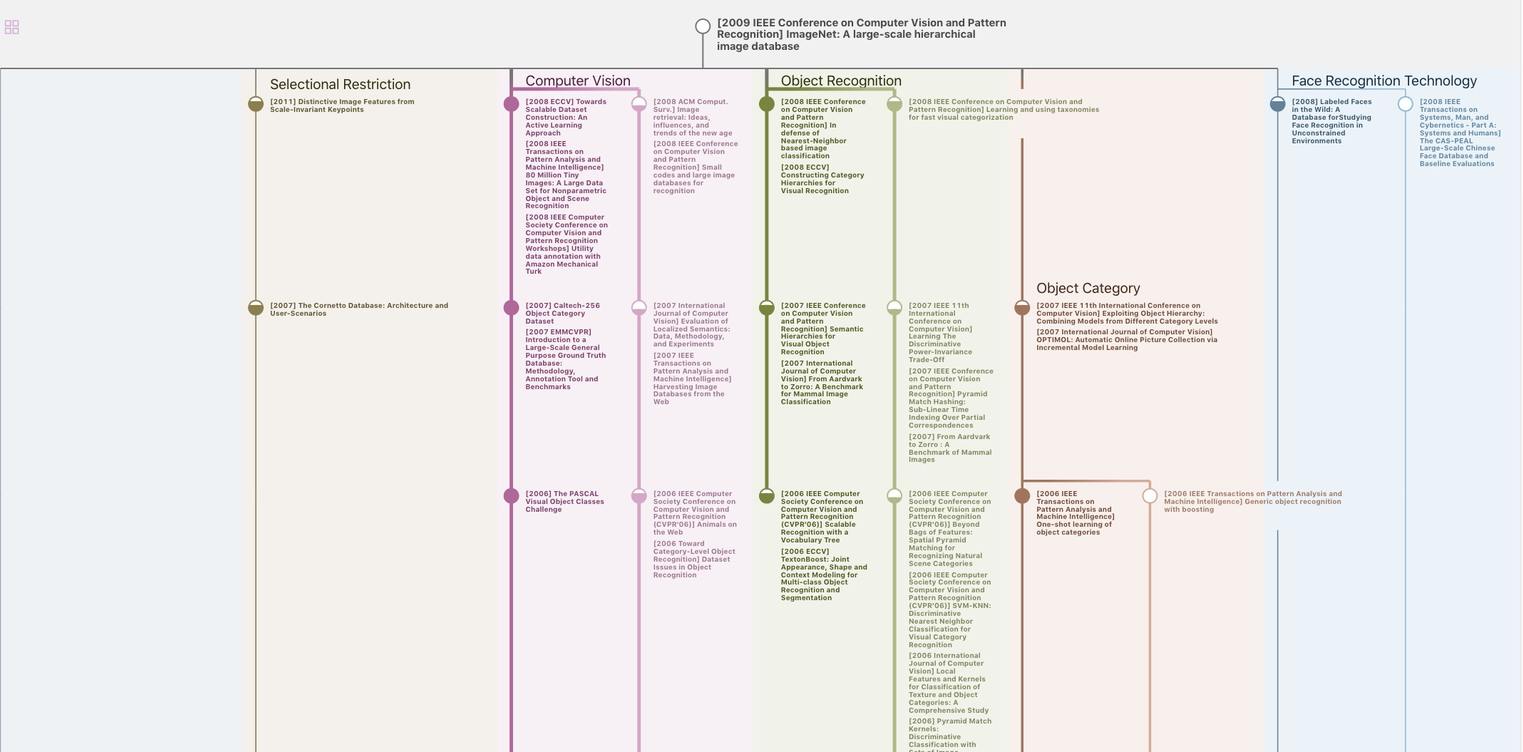
生成溯源树,研究论文发展脉络
Chat Paper
正在生成论文摘要