Cross-Modulated Few-Shot Image Generation for Colorectal Tissue Classification
MEDICAL IMAGE COMPUTING AND COMPUTER ASSISTED INTERVENTION, MICCAI 2023, PT III(2023)
摘要
In this work, we propose a few-shot colorectal tissue image generation method for addressing the scarcity of histopathological training data for rare cancer tissues. Our few-shot generation method, named XM-GAN, takes one base and a pair of reference tissue images as input and generates high-quality yet diverse images. Within our XM-GAN, a novel controllable fusion block densely aggregates local regions of reference images based on their similarity to those in the base image, resulting in locally consistent features. To the best of our knowledge, we are the first to investigate few-shot generation in colorectal tissue images. We evaluate our few-shot colorectral tissue image generation by performing extensive qualitative, quantitative and subject specialist (pathologist) based evaluations. Specifically, in specialist-based evaluation, pathologists could differentiate between our XM-GAN generated tissue images and real images only 55% time. Moreover, we utilize these generated images as data augmentation to address the few-shot tissue image classification task, achieving a gain of 4.4% in terms ofmean accuracy over the vanilla few-shot classifier. Code: https://github.com/VIROBO-15/XM-GAN.
更多查看译文
关键词
Few-shot Image generation,Cross Modulation
AI 理解论文
溯源树
样例
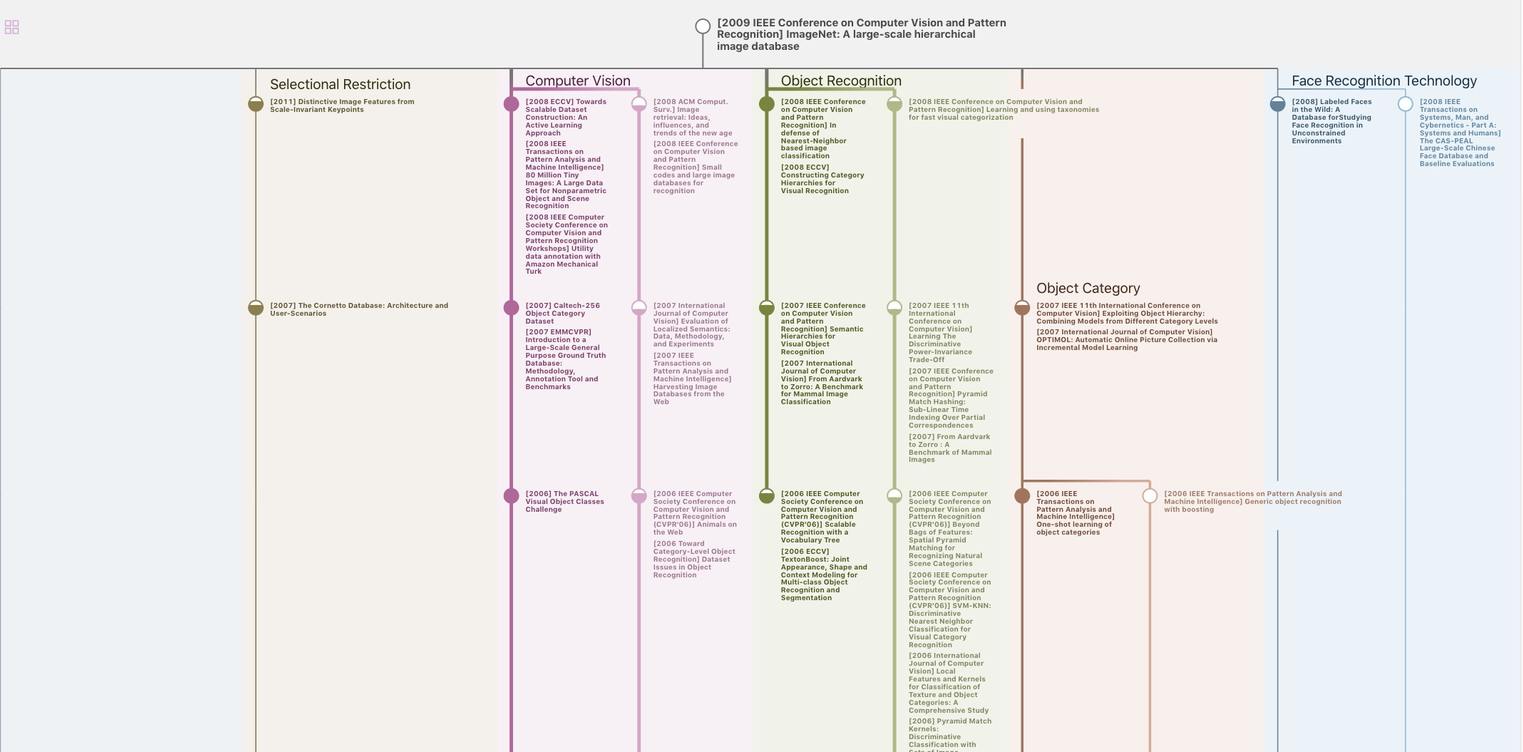
生成溯源树,研究论文发展脉络
Chat Paper
正在生成论文摘要