Sharp Deviations Bounds for Dirichlet Weighted Sums with Application to analysis of Bayesian algorithms
arXiv (Cornell University)(2023)
摘要
In this work, we derive sharp non-asymptotic deviation bounds for weighted sums of Dirichlet random variables. These bounds are based on a novel integral representation of the density of a weighted Dirichlet sum. This representation allows us to obtain a Gaussian-like approximation for the sum distribution using geometry and complex analysis methods. Our results generalize similar bounds for the Beta distribution obtained in the seminal paper Alfers and Dinges [1984]. Additionally, our results can be considered a sharp non-asymptotic version of the inverse of Sanov's theorem studied by Ganesh and O'Connell [1999] in the Bayesian setting. Based on these results, we derive new deviation bounds for the Dirichlet process posterior means with application to Bayesian bootstrap. Finally, we apply our estimates to the analysis of the Multinomial Thompson Sampling (TS) algorithm in multi-armed bandits and significantly sharpen the existing regret bounds by making them independent of the size of the arms distribution support.
更多查看译文
关键词
sharp deviations bounds,dirichlet,sums,algorithms
AI 理解论文
溯源树
样例
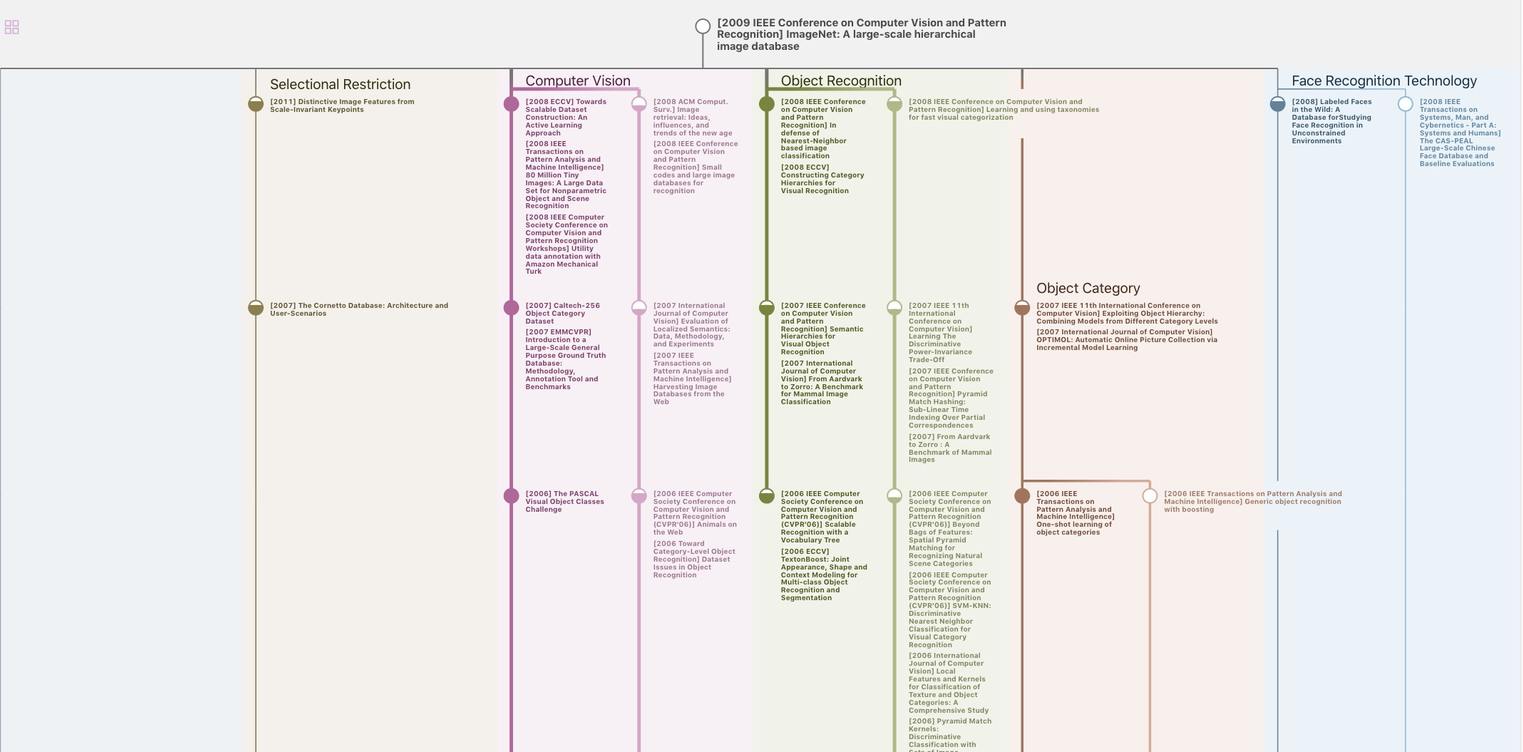
生成溯源树,研究论文发展脉络
Chat Paper
正在生成论文摘要