Zero-Shot Generative Model Adaptation Via Image-Specific Prompt Learning
CVPR 2023(2023)
摘要
Recently, CLIP-guided image synthesis has shown appealing performance on adapting a pre-trained source-domain generator to an unseen target domain. It does not require any target-domain samples but only the textual domain labels. The training is highly efficient, e.g., a few minutes. However, existing methods still have some limitations in the quality of generated images and may suffer from the mode collapse issue. A key reason is that a fixed adaptation direction is applied for all cross-domain image pairs, which leads to identical supervision signals. To address this issue, we propose an Image-specific Prompt Learning (IPL) method, which learns specific prompt vectors for each source-domain image. This produces a more precise adaptation direction for every cross-domain image pair, endowing the target-domain generator with greatly enhanced flexibility. Qualitative and quantitative evaluations on various domains demonstrate that IPL effectively improves the quality and diversity of synthesized images and alleviates the mode collapse. Moreover, IPL is independent of the structure of the generative model, such as generative adversarial networks or diffusion models. Code is available at https://github.com/Picsart-AI-Research/IPL-Zero-Shot-Generative-Model-Adaptation.
更多查看译文
关键词
Image Inpainting,Image Synthesis,Unsupervised Learning,Autofocusing,Representation Learning
AI 理解论文
溯源树
样例
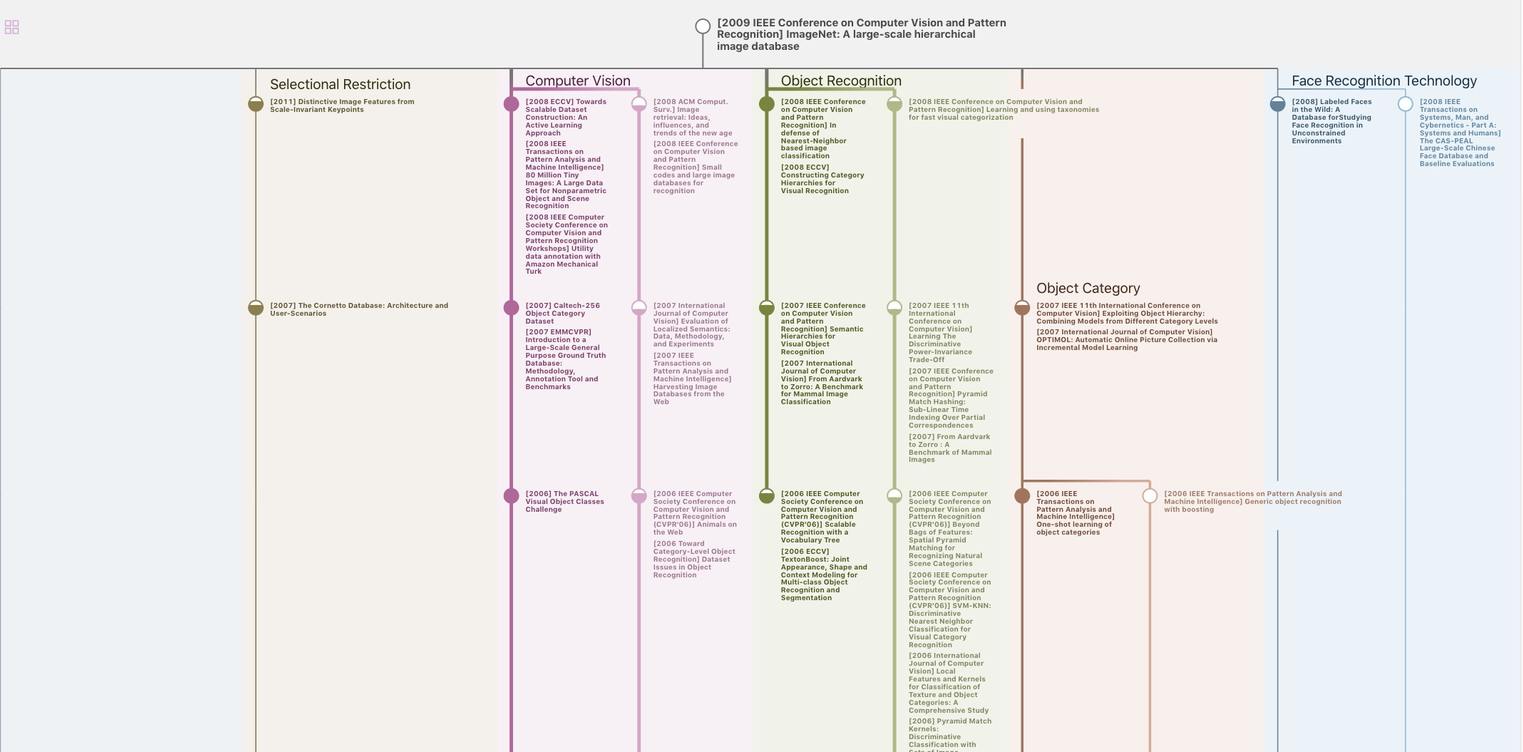
生成溯源树,研究论文发展脉络
Chat Paper
正在生成论文摘要