Graph Attention for Automated Audio Captioning
IEEE Signal Processing Letters(2023)
摘要
State-of-the-art audio captioning methods typically use the encoder-decoder structure with pretrained audio neural networks (PANNs) as encoders for feature extraction. However, the convolution operation used in PANNs is limited in capturing the long-time dependencies within an audio signal, thereby leading to potential performance degradation in audio captioning. This letter presents a novel method using graph attention (GraphAC) for encoder-decoder based audio captioning. In the encoder, a graph attention module is introduced after the PANNs to learn contextual association (i.e. the dependency among the audio features over different time frames) through an adjacency graph, and a top-k mask is used to mitigate the interference from noisy nodes. The learnt contextual association leads to a more effective feature representation with feature node aggregation. As a result, the decoder can predict important semantic information about the acoustic scene and events based on the contextual associations learned from the audio signal. Experimental results show that GraphAC outperforms the state-of-the-art methods with PANNs as the encoders, thanks to the incorporation of the graph attention module into the encoder for capturing the long-time dependencies within the audio signal.
更多查看译文
关键词
Feature extraction,Decoding,Transformers,Semantics,Acoustics,Noise measurement,Matrix converters,Audio modelling,automated audio captioning,graph attention network,temporal information
AI 理解论文
溯源树
样例
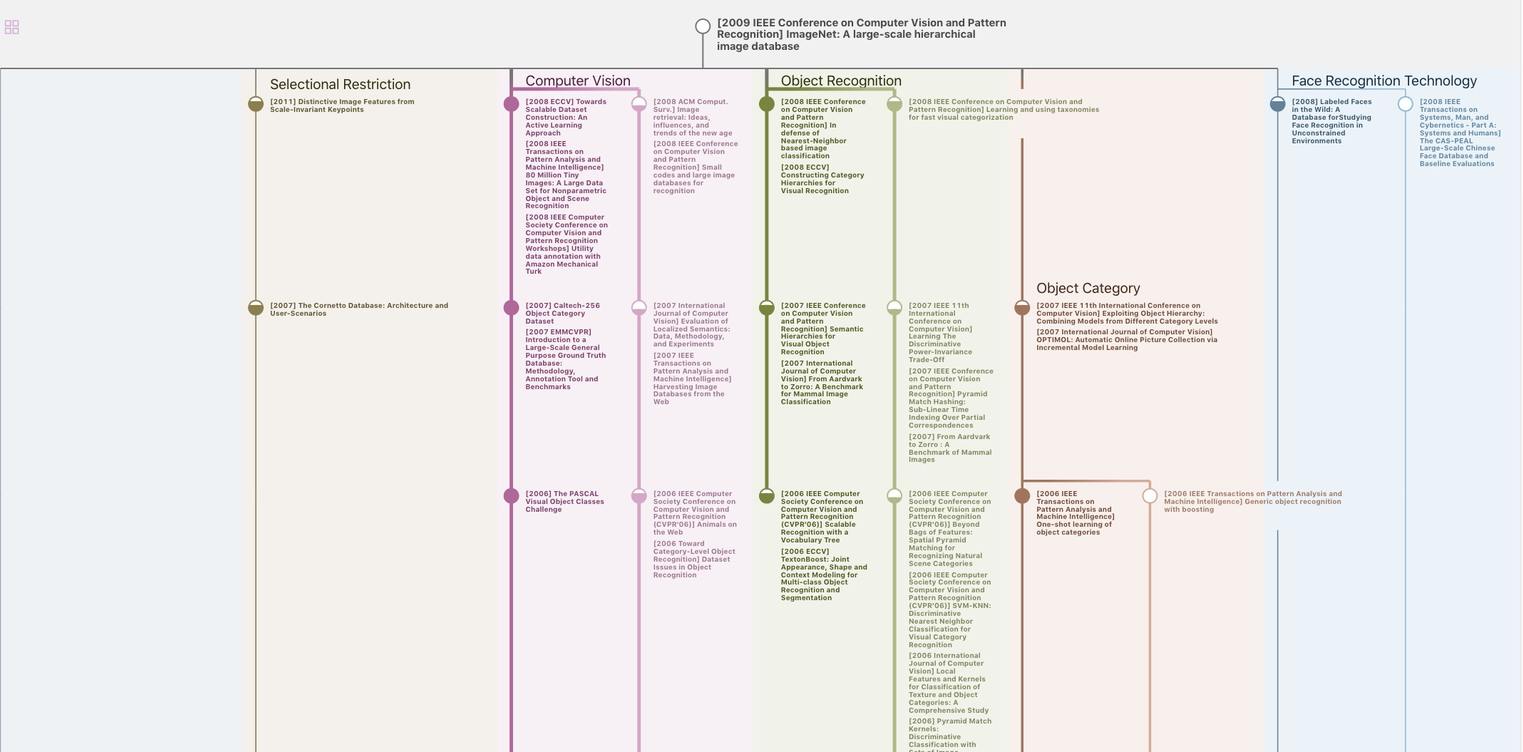
生成溯源树,研究论文发展脉络
Chat Paper
正在生成论文摘要