NeRC: Rendering Planar Caustics by Learning Implicit Neural Representations.
IEEE transactions on visualization and computer graphics(2023)
摘要
Caustics are challenging light transport effects for photo-realistic rendering. Photon mapping techniques play a fundamental role in rendering caustics. However, photon mapping methods render single caustics under the stationary light source in a fixed scene view. They require significant storage and computing resources to produce high-quality results. In this paper, we propose efficiently rendering more diverse caustics of a scene with the camera and the light source moving. We present a novel learning-based volume rendering approach with implicit representations for our proposed task. Considering the variety of materials and textures of planar caustic receivers, we decompose the output appearance into two components: the diffuse and specular parts with a probabilistic module. Unlike NeRF, we construct weights for rendering each component from the implicit signed distance function (SDF). Moreover, we introduce the centering calibration and the sine activation function to improve the performance of the color prediction network. Extensive experiments on the synthetic and real-world datasets illustrate that our method achieves much better performance than baselines in the quantitative and qualitative comparison, for rendering caustics in novel views with the dynamic light source. Especially, our method outperforms the baseline on the temporal consistency across frames. Code will be available at https://github.com/JiaxiongQ/NeRC.
更多查看译文
关键词
Caustics,photon mapping,volume rendering,centering calibration,sine activation
AI 理解论文
溯源树
样例
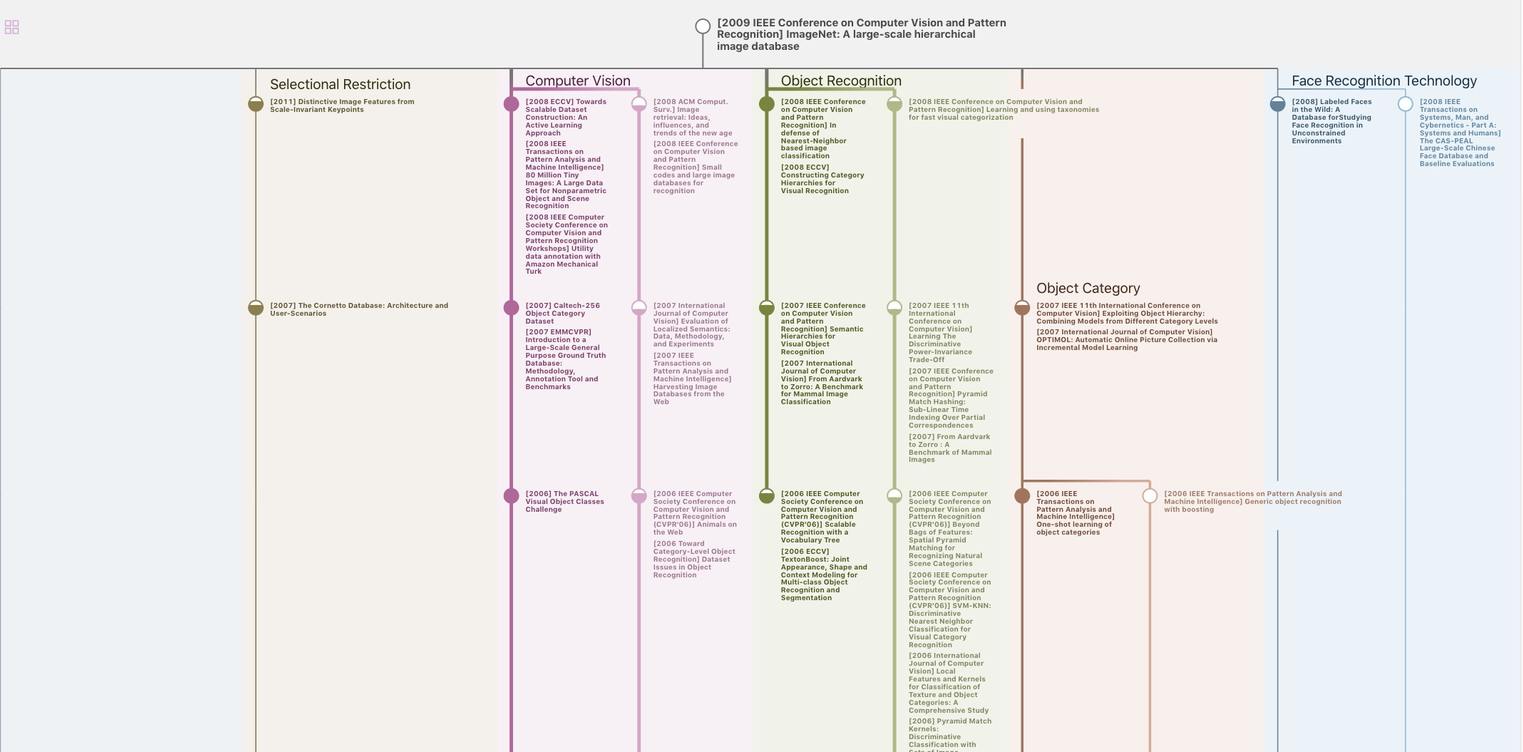
生成溯源树,研究论文发展脉络
Chat Paper
正在生成论文摘要