ProsodyBERT: Self-Supervised Prosody Representation for Style-Controllable TTS
ICLR 2023(2023)
Abstract
We propose ProsodyBERT, a self-supervised approach to learning prosody representations from raw audio. Different from most previous works, which use information bottlenecks to disentangle prosody features from speech content and speaker information, we perform an offline clustering of speaker-normalized prosody-related features (energy, pitch, their dynamics, etc.) and use the cluster labels as targets for HuBERT-like masked unit prediction. A span boundary loss is also introduced to capture long-range prosodic information. We demonstrate the effectiveness of ProsodyBERT on a multi-speaker style-controllable text-to-speech (TTS) system. Experiments show that the TTS system trained with ProsodyBERT features can generate natural and expressive speech samples, surpassing the model supervised by energy and pitch on subjective human evaluation. Also, the style and expressiveness of synthesized audio can be controlled by manipulating the prosody features. In addition, We achieve new state-of-the-art results on the IEMOCAP emotion recognition task by combining our prosody features with HuBERT features, showing that ProsodyBERT is complementary to popular pretrained speech self-supervised models.
MoreTranslated text
Key words
prosody,self-supervised learning,text-to-speech,speech processing,emotion recognition,speech synthesis
AI Read Science
Must-Reading Tree
Example
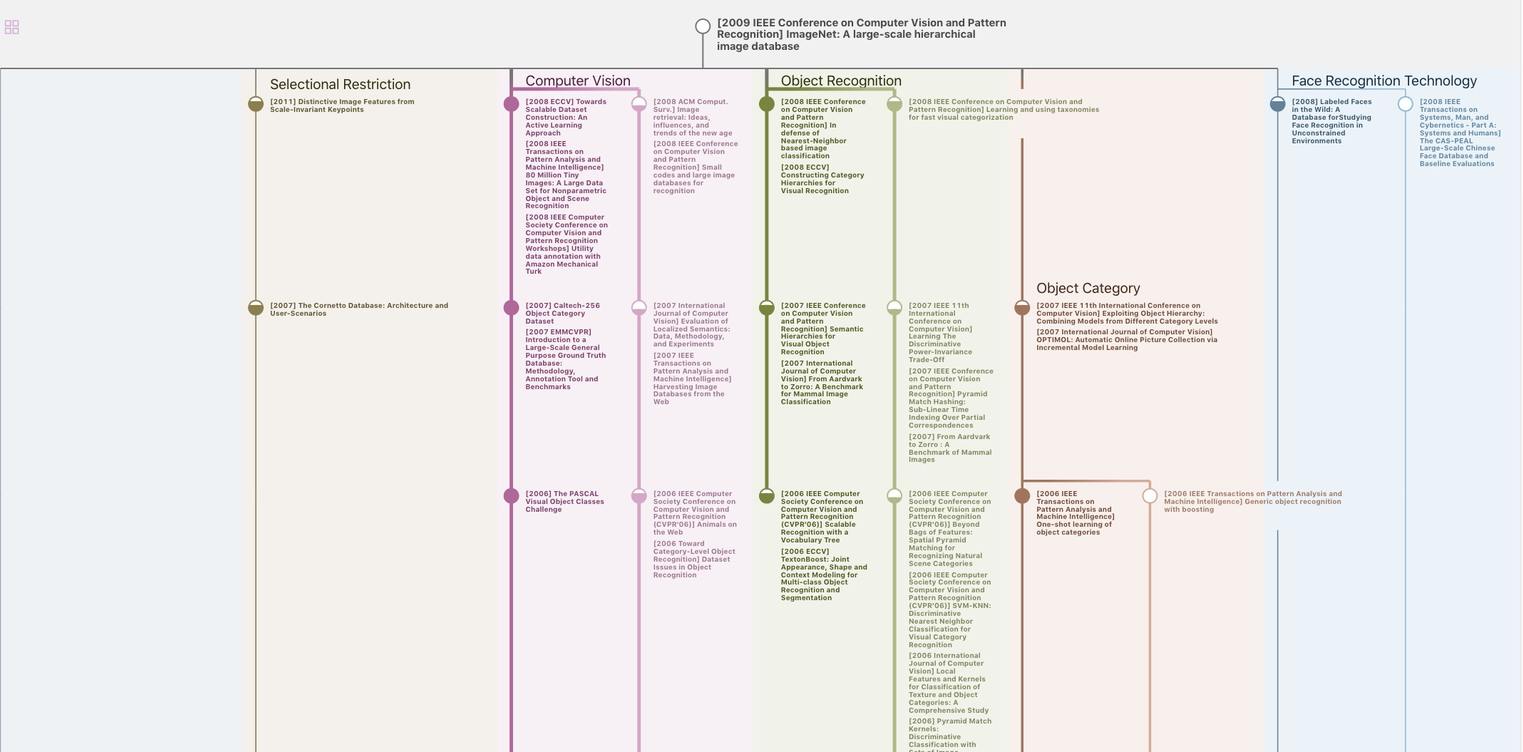
Generate MRT to find the research sequence of this paper
Chat Paper
Summary is being generated by the instructions you defined