Approximate Conditional Coverage via Neural Model Approximations
ICLR 2023(2023)
摘要
We propose a new approach for constructing prediction sets for Transformer networks via the strong signals for prediction reliability from KNN-based approximations. This enables a data-driven partitioning of the high-dimensional feature space and a new Inductive Venn Predictor for calibration, the Venn-ADMIT Predictor. Our approach more closely obtains approximate conditional coverage than recent work proposing adaptive and localized conformal score functions for deep networks. We analyze coverage on several representative natural language processing classification tasks, including class-imbalanced and distribution-shifted settings.
更多查看译文
关键词
distribution-free uncertainty quantification,split-conformal prediction sets,Venn Predictors
AI 理解论文
溯源树
样例
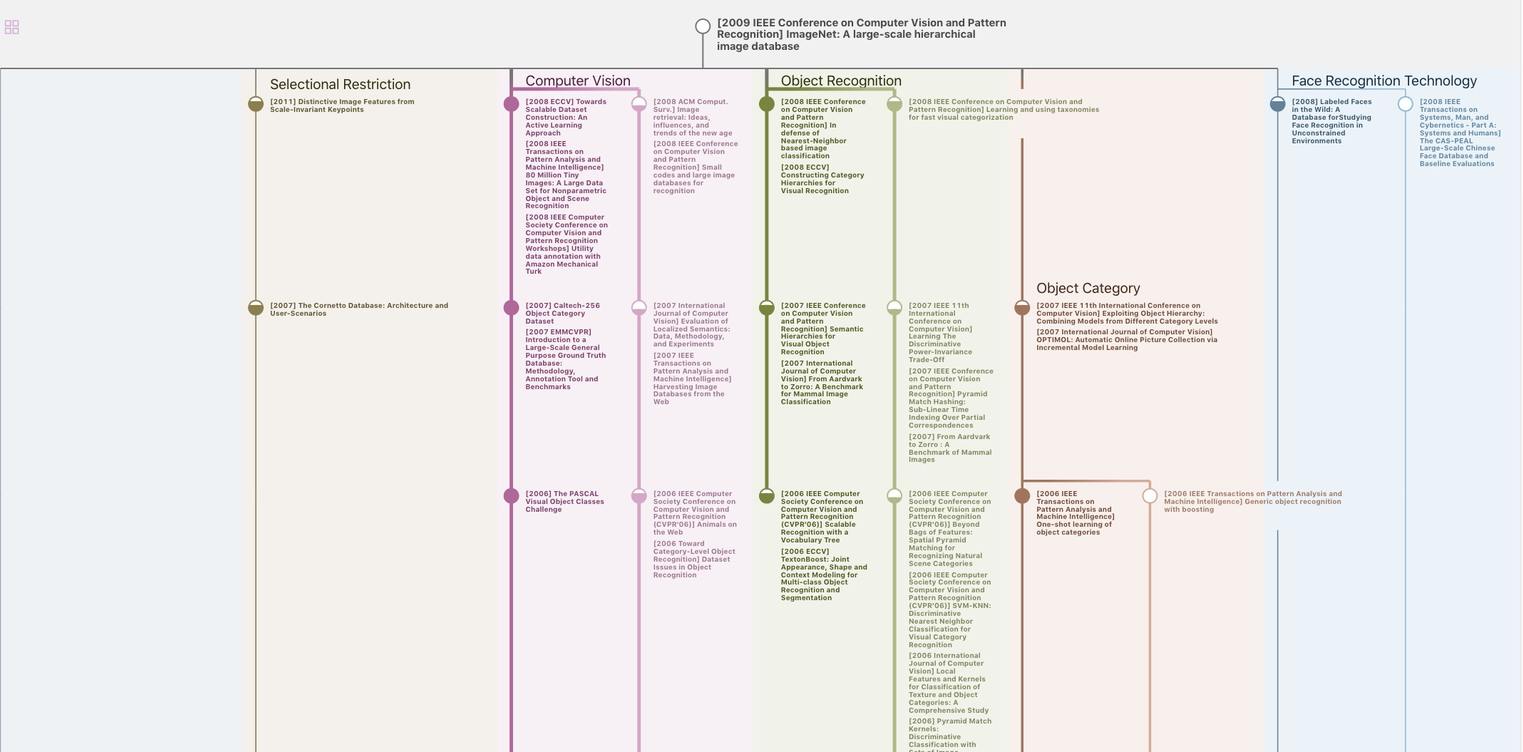
生成溯源树,研究论文发展脉络
Chat Paper
正在生成论文摘要