Learning Disentangled Representation via Domain Adaptation for Dialogue Summarization
WWW 2023(2023)
摘要
Dialogue summarization, which aims to generate a summary for an input dialogue, plays a vital role in intelligent dialogue systems. The end-to-end models have achieved satisfactory performance in summarization, but the success is built upon enough annotated data, which is costly to obtain, especially in the dialogue summarization. To leverage the rich external data, previous works first pre-train the model on the other domain data (e.g., the news domain), and then fine-tune it directly on the dialogue domain. The data from different domains are equally treated during the training process, while the vast differences between dialogues (usually informal, repetitive, and with multiple speakers) and conventional articles (usually formal and concise) are neglected. In this work, we propose to use a disentangled representation method to reduce the deviation between data in different domains, where the input data is disentangled into domain-invariant and domain-specific representations. The domain-invariant representation carries context information that is supposed to be the same across domains (e.g., news, dialogue) and the domain-specific representation indicates the input data belongs to a particular domain. We use adversarial learning and contrastive learning to constrain the disentangled representations to the target space. Furthermore, we propose two novel reconstruction strategies, namely backtracked and cross-track reconstructions, which aim to reduce the domain characteristics of out-of-domain data and mitigate the domain bias of the model. Experimental results on three public datasets show that our model significantly outperforms the strong baselines.
更多查看译文
AI 理解论文
溯源树
样例
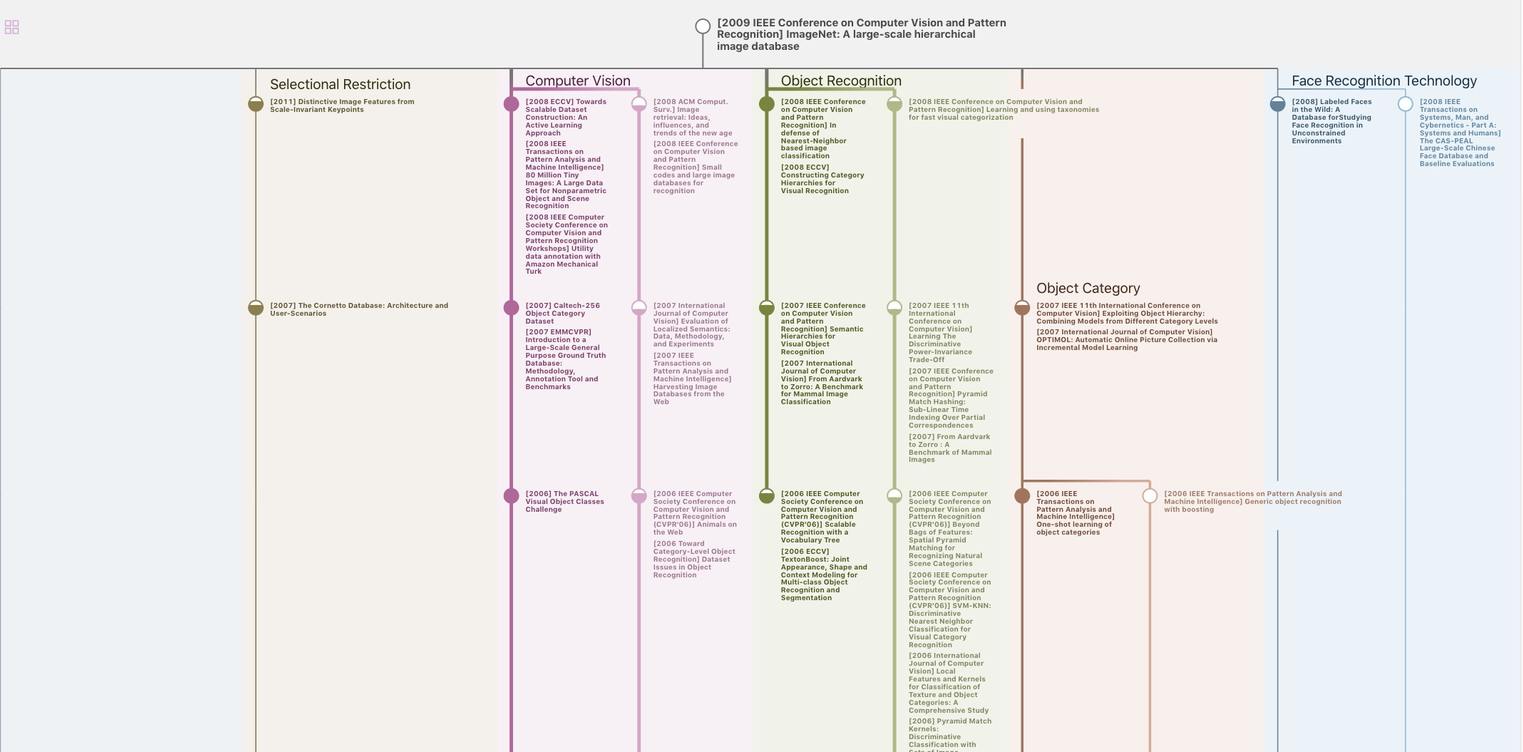
生成溯源树,研究论文发展脉络
Chat Paper
正在生成论文摘要