Get Back Here: Robust Imitation by Return-to-Distribution Planning
CoRR(2023)
摘要
We consider the Imitation Learning (IL) setup where expert data are not collected on the actual deployment environment but on a different version. To address the resulting distribution shift, we combine behavior cloning (BC) with a planner that is tasked to bring the agent back to states visited by the expert whenever the agent deviates from the demonstration distribution. The resulting algorithm, POIR, can be trained offline, and leverages online interactions to efficiently fine-tune its planner to improve performance over time. We test POIR on a variety of human-generated manipulation demonstrations in a realistic robotic manipulation simulator and show robustness of the learned policy to different initial state distributions and noisy dynamics.
更多查看译文
关键词
robust imitation,planning,return-to-distribution
AI 理解论文
溯源树
样例
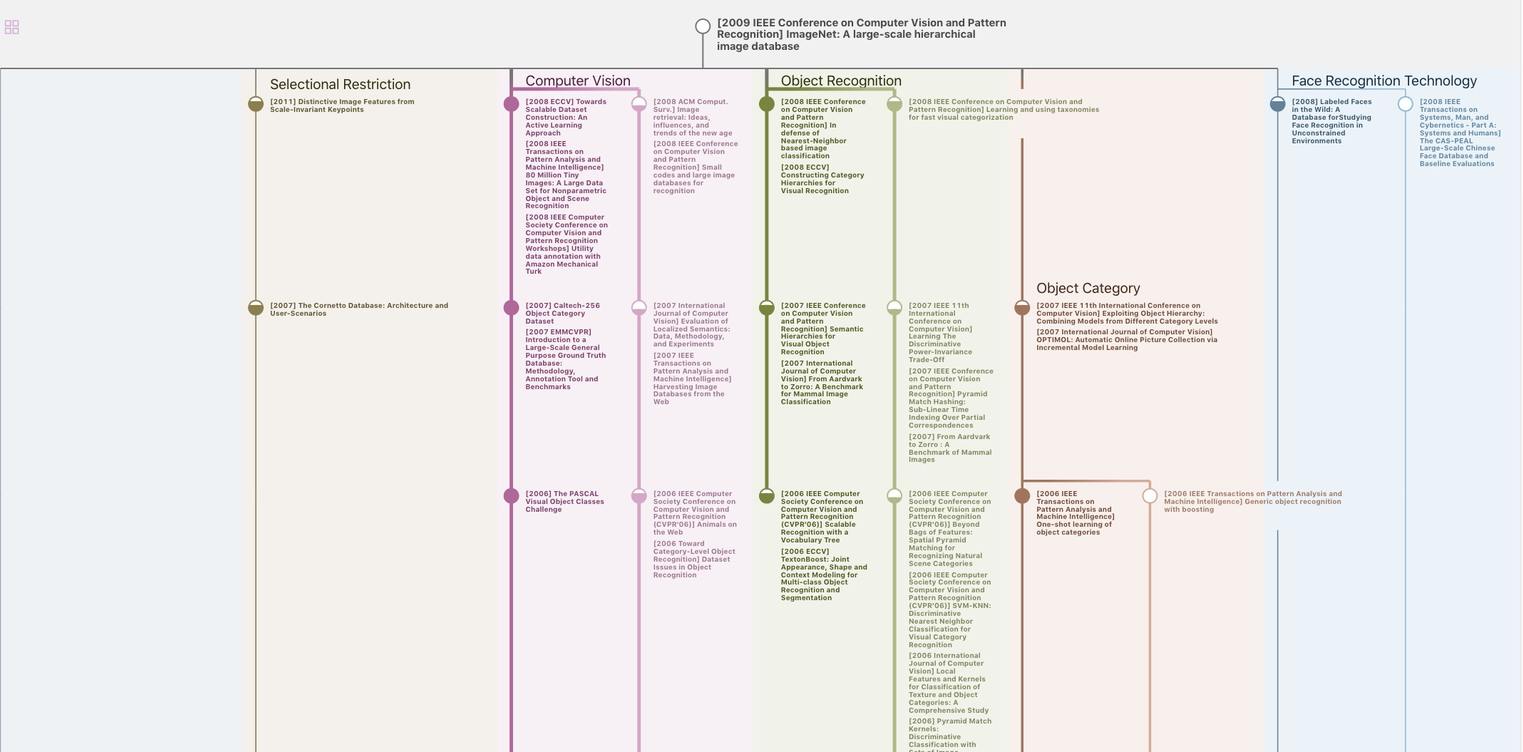
生成溯源树,研究论文发展脉络
Chat Paper
正在生成论文摘要