Elpis: Graph-Based Similarity Search for Scalable Data Science.
Proc. VLDB Endow.(2023)
摘要
The recent popularity of learned embeddings has fueled the growth of massive collections of high-dimensional (high-d) vectors that model complex data. Finding similar vectors in these collections is at the core of many important and practical data science applications. The data series community has developed tree-based similarity search techniques that outperform state-of-the-art methods on large collections of both data series and generic high-d vectors, on all scenarios except for no-guarantees ng-approximate search, where graph-based approaches designed by the high-d vector community achieve the best performance. However, building graph-based indexes is extremely expensive both in time and space. In this paper, we bring these two worlds together, study the corresponding solutions and their performance behavior, and propose ELPIS, a new strong baseline that takes advantage of the best features of both to achieve a superior performance in terms of indexing and ng-approximate search in-memory. ELPIS builds the index 3x-8x faster than competitors, using 40% less memory. It also achieves a high recall of 0.99, up to 2x faster than the state-of-the-art methods, and answers 1-NN queries up to one order of magnitude faster.
更多查看译文
关键词
scalable data science,similarity search,graph-based
AI 理解论文
溯源树
样例
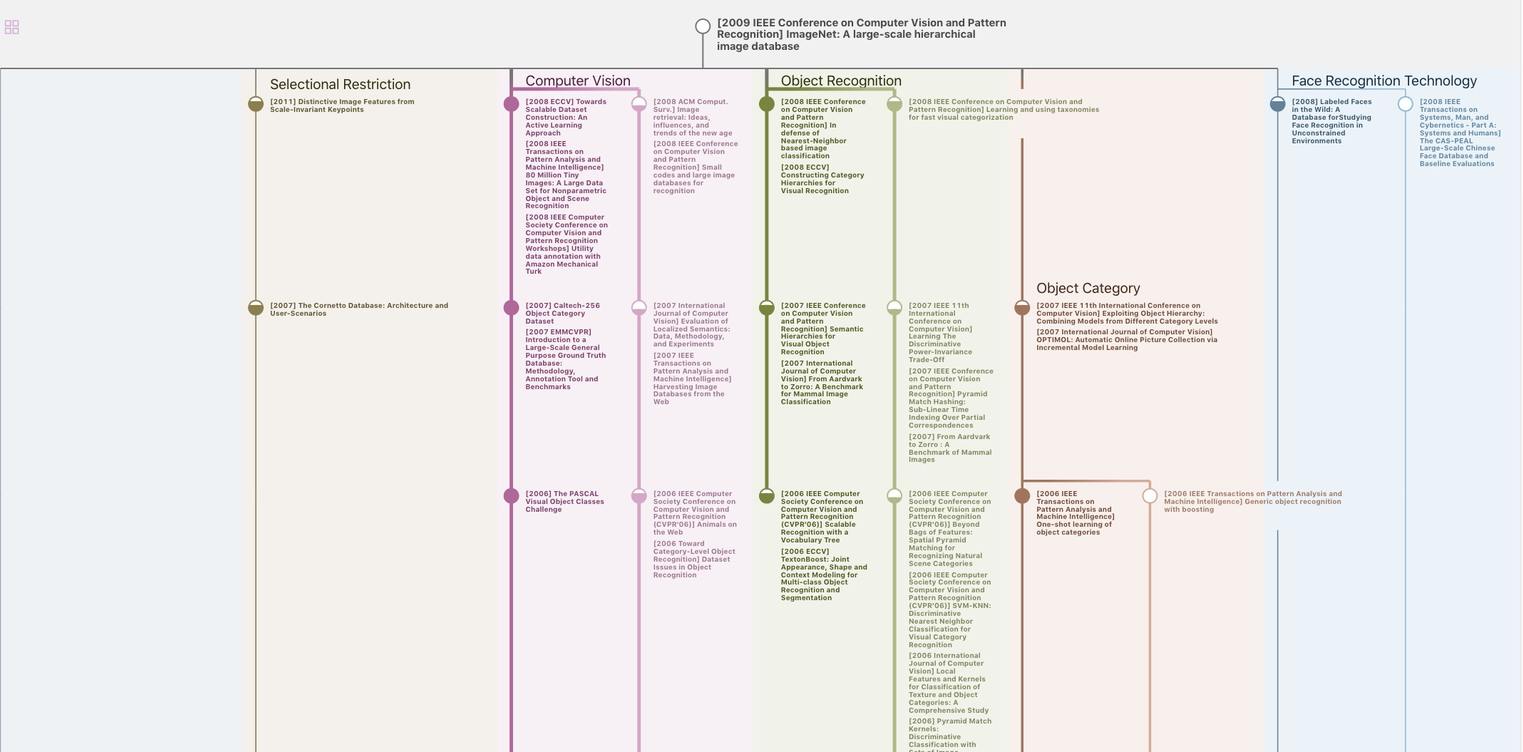
生成溯源树,研究论文发展脉络
Chat Paper
正在生成论文摘要