Deep Manifold Graph Auto-Encoder for Attributed Graph Embedding
ICASSP 2023 - 2023 IEEE International Conference on Acoustics, Speech and Signal Processing (ICASSP)(2024)
摘要
Representing graph data in a low-dimensional space for subsequent tasks is the purpose of attributed graph embedding. Most existing neural network approaches learn latent representations by minimizing reconstruction errors. Rare work considers the data distribution and the topological structure of latent codes simultaneously, which often results in inferior embeddings in real-world graph data. This paper proposes a novel Deep Manifold (Variational) Graph Auto-Encoder (DMVGAE/DMGAE) method for attributed graph data to improve the stability and quality of learned representations to tackle the crowding problem. The node-to-node geodesic similarity is preserved between the original and latent space under a pre-defined distribution. The proposed method surpasses state-of-the-art baseline algorithms by a significant margin on different downstream tasks across popular datasets, which validates our solutions. We promise to release the code after acceptance.
更多查看译文
关键词
Manifold learning,structure information,graph embedding,crowding problem
AI 理解论文
溯源树
样例
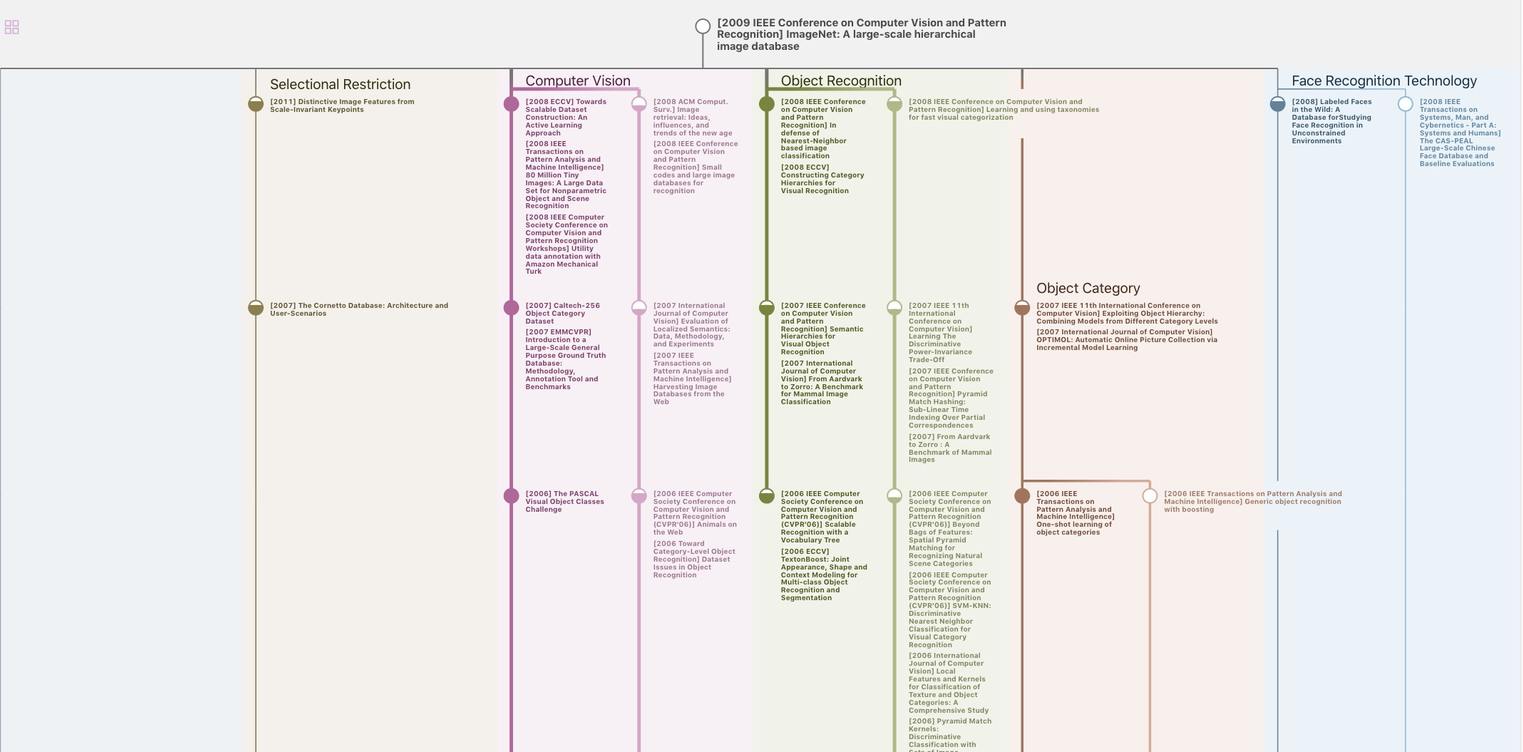
生成溯源树,研究论文发展脉络
Chat Paper
正在生成论文摘要