Enhancing Continual Relation Extraction via Classifier Decomposition
conf_acl(2023)
摘要
Continual relation extraction (CRE) models aim at handling emerging new relations while avoiding catastrophically forgetting old ones in the streaming data. Though improvements have been shown by previous CRE studies, most of them only adopt a vanilla strategy when models first learn representations of new relations. In this work, we point out that there exist two typical biases after training of this vanilla strategy: classifier bias and representation bias, which causes the previous knowledge that the model learned to be shaded. To alleviate those biases, we propose a simple yet effective classifier decomposition framework that splits the last FFN layer into separated previous and current classifiers, so as to maintain previous knowledge and encourage the model to learn more robust representations at this training stage. Experimental results on two standard benchmarks show that our proposed framework consistently outperforms the state-of-the-art CRE models, which indicates that the importance of the first training stage to CRE models may be underestimated. Our code is available at https://github.com/hemingkx/CDec.
更多查看译文
关键词
continual relation extraction,classifier decomposition
AI 理解论文
溯源树
样例
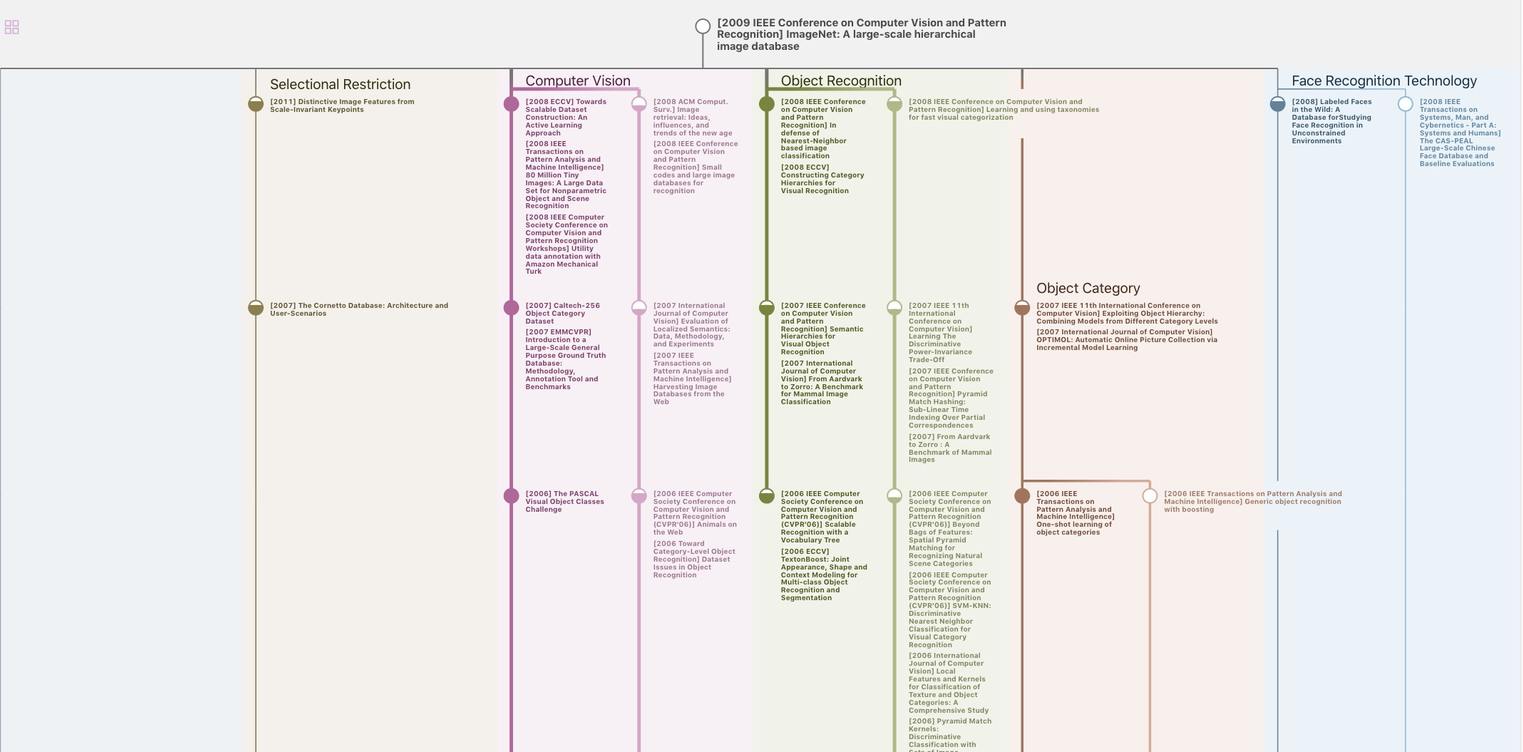
生成溯源树,研究论文发展脉络
Chat Paper
正在生成论文摘要