Vertical Federated Learning Over Cloud-RAN: Convergence Analysis and System Optimization
IEEE TRANSACTIONS ON WIRELESS COMMUNICATIONS(2024)
摘要
Vertical federated learning (FL) is a collaborative machine learning framework that enables devices to learn a global model from the feature-partition datasets without sharing local raw data. However, as the number of the local intermediate outputs is proportional to the training samples, it is critical to develop communication-efficient techniques for wireless vertical FL to support high-dimensional model aggregation with full device participation. In this paper, we propose a novel cloud radio access network (Cloud-RAN) based vertical FL system to enable fast and accurate model aggregation by leveraging over-the-air computation (AirComp) and alleviating communication straggler issue with cooperative model aggregation among geographically distributed edge servers. However, the model aggregation error caused by AirComp and quantization errors caused by the limited fronthaul capacity degrade the learning performance for vertical FL. To address these issues, we characterize the convergence behavior of the vertical FL algorithm considering both uplink and downlink transmissions. To improve the learning performance, we establish a system optimization framework by joint transceiver and fronthaul quantization design, for which successive convex approximation and alternate convex search based system optimization algorithms are developed. We conduct extensive simulations to demonstrate the effectiveness of the proposed system architecture and optimization framework for vertical FL.
更多查看译文
关键词
Servers,Wireless communication,Atmospheric modeling,Training,Data models,Optimization,Computational modeling,Vertical federated learning,cloud radio access network,over-the-air computation
AI 理解论文
溯源树
样例
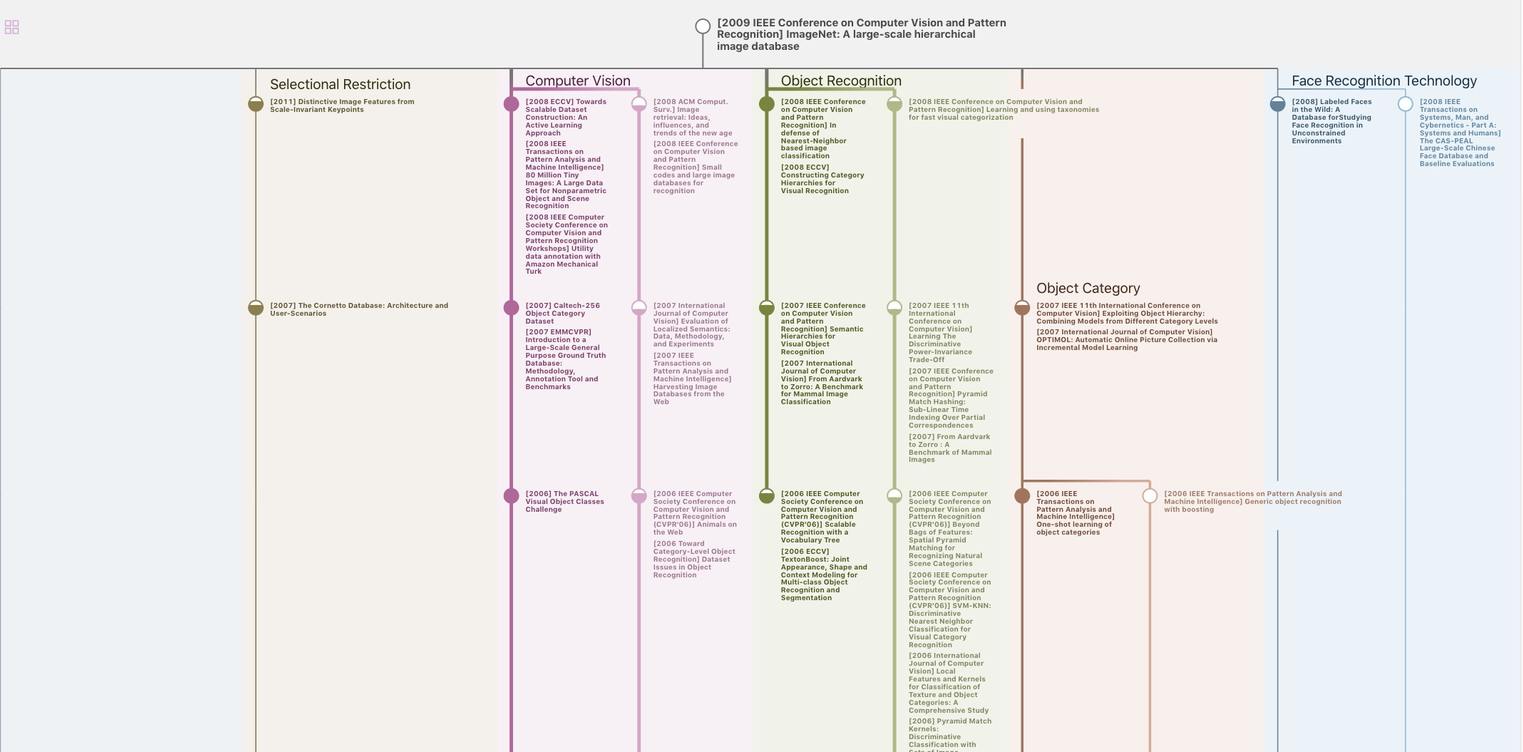
生成溯源树,研究论文发展脉络
Chat Paper
正在生成论文摘要