Identifying Malignant Breast Ultrasound Images Using ViT-Patch
APPLIED SCIENCES-BASEL(2023)
摘要
Recently, the Vision Transformer (ViT) model has been used for various computer vision tasks, due to its advantages to extracting long-range features. To better integrate the long-range features useful for classification, the standard ViT adds a class token, in addition to patch tokens. Despite state-of-the-art results on some traditional vision tasks, the ViT model typically requires large datasets for supervised training, and thus, it still face challenges in areas where it is difficult to build large datasets, such as medical image analysis. In the ViT model, only the output corresponding to the class token is fed to a Multi-Layer Perceptron (MLP) head for classification, and the outputs corresponding to the patch tokens are exposed. In this paper, we propose an improved ViT architecture (called ViT-Patch), which adds a shared MLP head to the output of each patch token to balance the feature learning on the class and patch tokens. In addition to the primary task, which uses the output of the class token to discriminate whether the image is malignant, a secondary task is introduced, which uses the output of each patch token to determine whether the patch overlaps with the tumor area. More interestingly, due to the correlation between the primary and secondary tasks, the supervisory information added to the patch tokens help with improving the performance of the primary task on the class token. The introduction of secondary supervision information also improves the attention interaction among the class and patch tokens. And by this way, ViT reduces the demand on dataset size. The proposed ViT-Patch is validated on a publicly available dataset, and the experimental results show its effectiveness for both malignant identification and tumor localization.
更多查看译文
关键词
ViT,ultrasound,classification,detection,attention map,multi-task learning,auxiliary learning
AI 理解论文
溯源树
样例
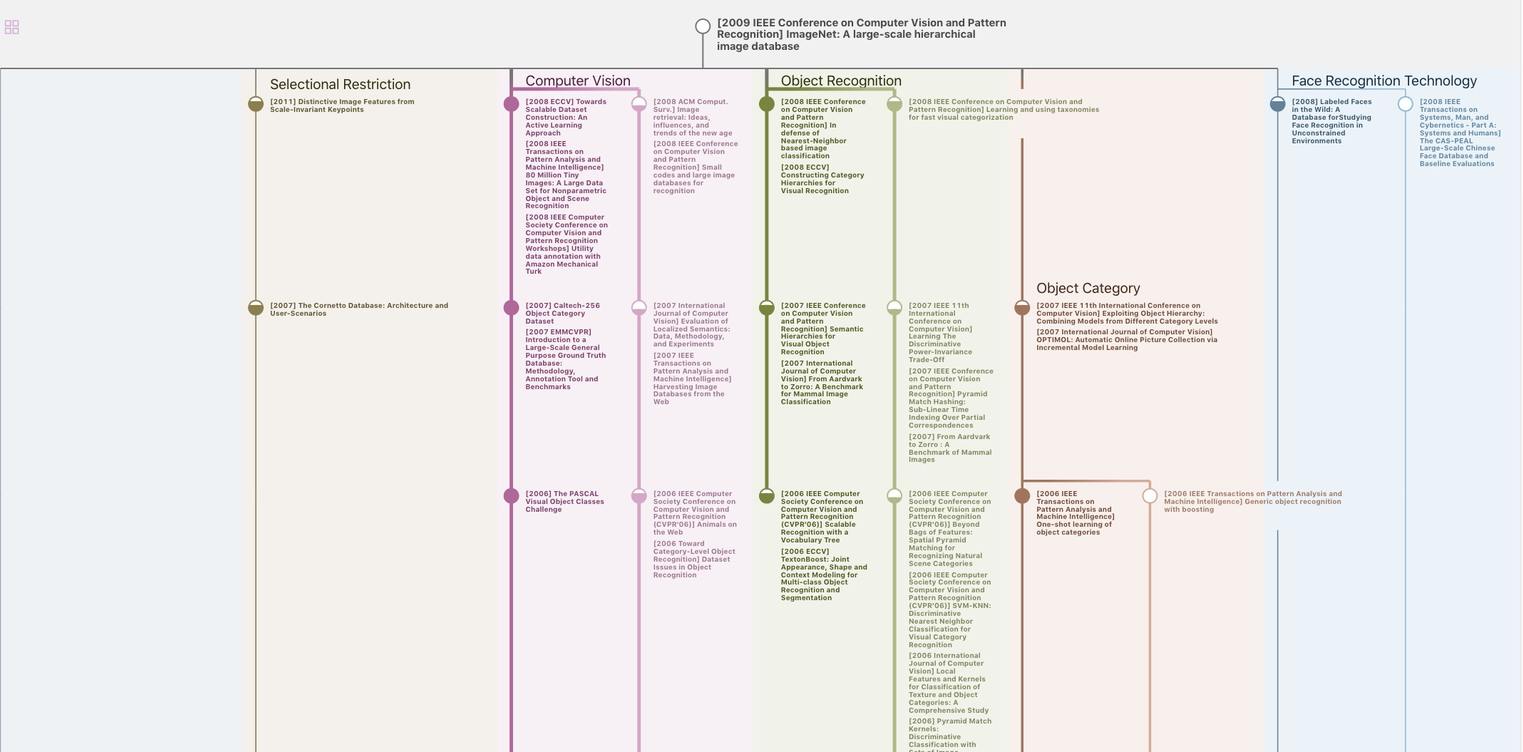
生成溯源树,研究论文发展脉络
Chat Paper
正在生成论文摘要