LiDAR-only 3D object detection based on spatial context
JOURNAL OF VISUAL COMMUNICATION AND IMAGE REPRESENTATION(2023)
摘要
LiDAR-based 3D Object detection is one of the popular topics in recent years, and it is widely used in the fields of autonomous driving and robot controlling. However, due to the scanning pattern of LiDAR, the point clouds of objects at far distance are sparse and more difficult to be detected. To solve this problem, we propose a two-stage network based on spatial context information, named SC-RCNN (Spatial Context RCNN), for object detection in 3D point cloud scenes. SC-RCNN first uses a backbone with sparse convolutions and submanifold sparse convolutions to extract the voxel features of point scenes and generate a series of candidate boxes. For the sparsity of far-distance point clouds, we design the local grid point pooling (LGP Pooling) to extract features and spatial context information around candidate regions for subsequent box refinement. In addition, we propose the pyramid candidate box augmentation (PCB Augmentation) to expand the candidate boxes with a multi-scale style, enriching the feature encoding. The experimental results show that SC-RCNN significantly outperforms previous methods on KITTI dataset and Waymo dataset, and is particularly robust to the sparsity of point clouds.
更多查看译文
关键词
3D object detection,Convolutional neural network,LiDAR,Computer vision,Deep learning
AI 理解论文
溯源树
样例
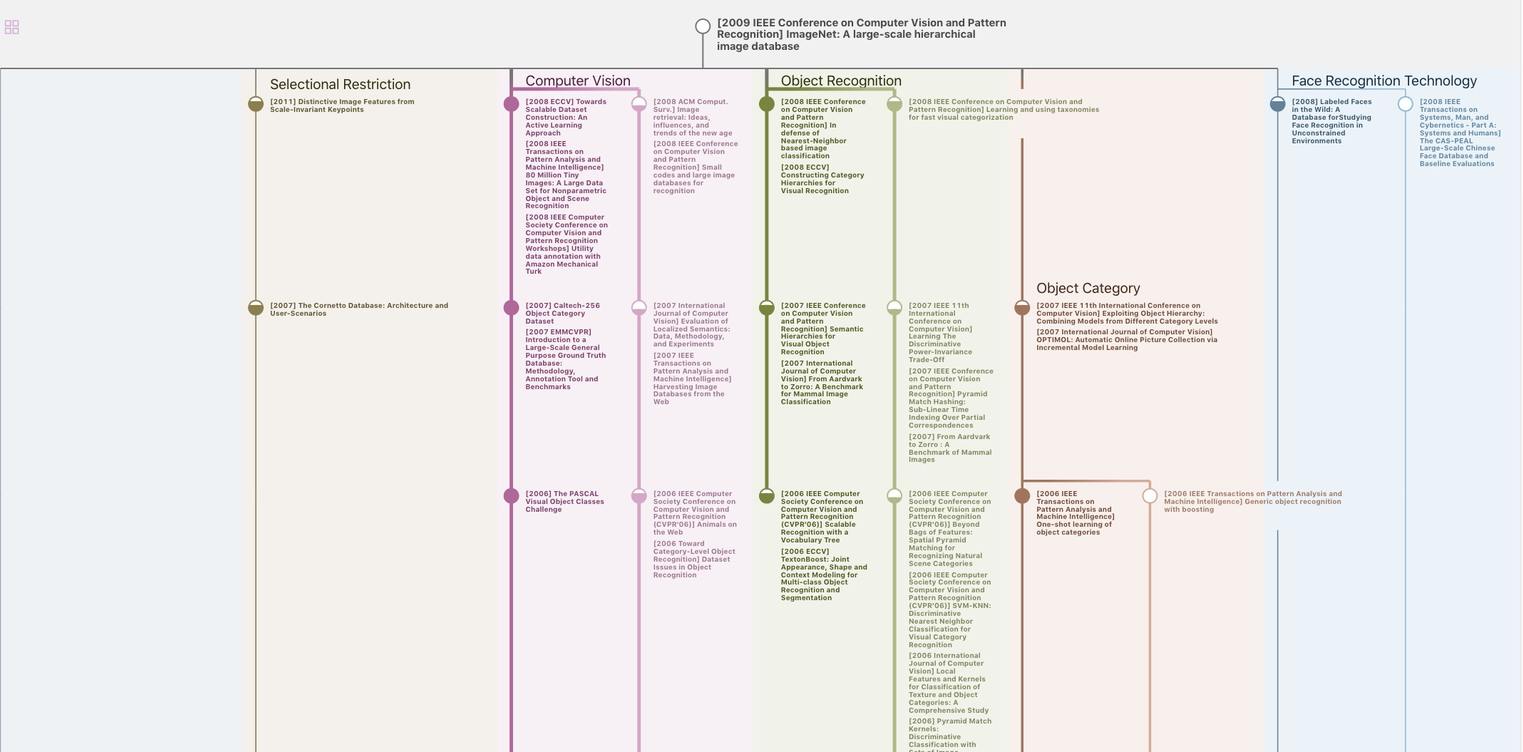
生成溯源树,研究论文发展脉络
Chat Paper
正在生成论文摘要