Deep multi-view subspace clustering with anchor graph
IJCAI 2023(2023)
摘要
Deep multi-view subspace clustering (DMVSC) has recently attracted increasing attention due to its promising performance. However, existing DMVSC methods still have two issues: (1) they mainly focus on using autoencoders to nonlinearly embed the data, while the embedding may be sub-optimal for clustering because the clustering objective is rarely considered in autoencoders, and (2) they typically have a quadratic or even cubic complexity, which makes it challenging to deal with large-scale data. To address these issues, in this paper we propose a novel deep multi-view subspace clustering method with anchor graph (DMCAG). To be specific, DMCAG firstly learns the embedded features for each view independently, which are used to obtain the subspace representations. To significantly reduce the complexity, we construct an anchor graph with small size for each view. Then, spectral clustering is performed on an integrated anchor graph to obtain pseudo-labels. To overcome the negative impact caused by suboptimal embedded features, we use pseudo-labels to refine the embedding process to make it more suitable for the clustering task. Pseudo-labels and embedded features are updated alternately. Furthermore, we design a strategy to keep the consistency of the labels based on contrastive learning to enhance the clustering performance. Empirical studies on real-world datasets show that our method achieves superior clustering performance over other state-of-the-art methods.
更多查看译文
关键词
multi-view
AI 理解论文
溯源树
样例
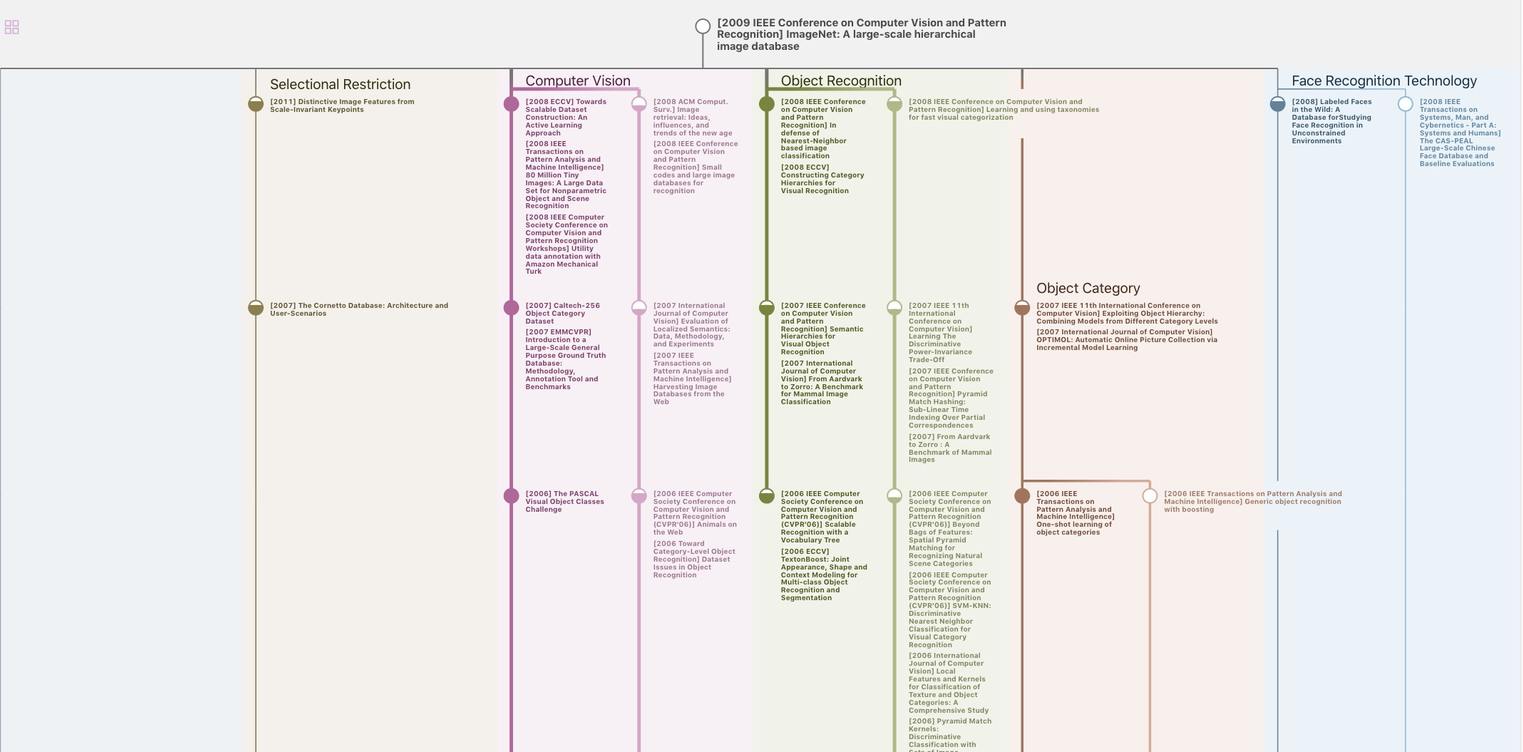
生成溯源树,研究论文发展脉络
Chat Paper
正在生成论文摘要