CARD: Channel Aligned Robust Blend Transformer for Time Series Forecasting
ICLR 2024(2023)
摘要
Recent studies have demonstrated the great power of Transformer models for
time series forecasting. One of the key elements that lead to the transformer's
success is the channel-independent (CI) strategy to improve the training
robustness. However, the ignorance of the correlation among different channels
in CI would limit the model's forecasting capacity. In this work, we design a
special Transformer, i.e., Channel Aligned Robust Blend
Transformer (CARD for short), that addresses key shortcomings of CI type
Transformer in time series forecasting. First, CARD introduces a
channel-aligned attention structure that allows it to capture both temporal
correlations among signals and dynamical dependence among multiple variables
over time. Second, in order to efficiently utilize the multi-scale knowledge,
we design a token blend module to generate tokens with different resolutions.
Third, we introduce a robust loss function for time series forecasting to
alleviate the potential overfitting issue. This new loss function weights the
importance of forecasting over a finite horizon based on prediction
uncertainties. Our evaluation of multiple long-term and short-term forecasting
datasets demonstrates that CARD significantly outperforms state-of-the-art time
series forecasting methods. The code is available at the following anonymous
repository:
更多查看译文
关键词
time series forecasting,transformer,robust learning,token mixing
AI 理解论文
溯源树
样例
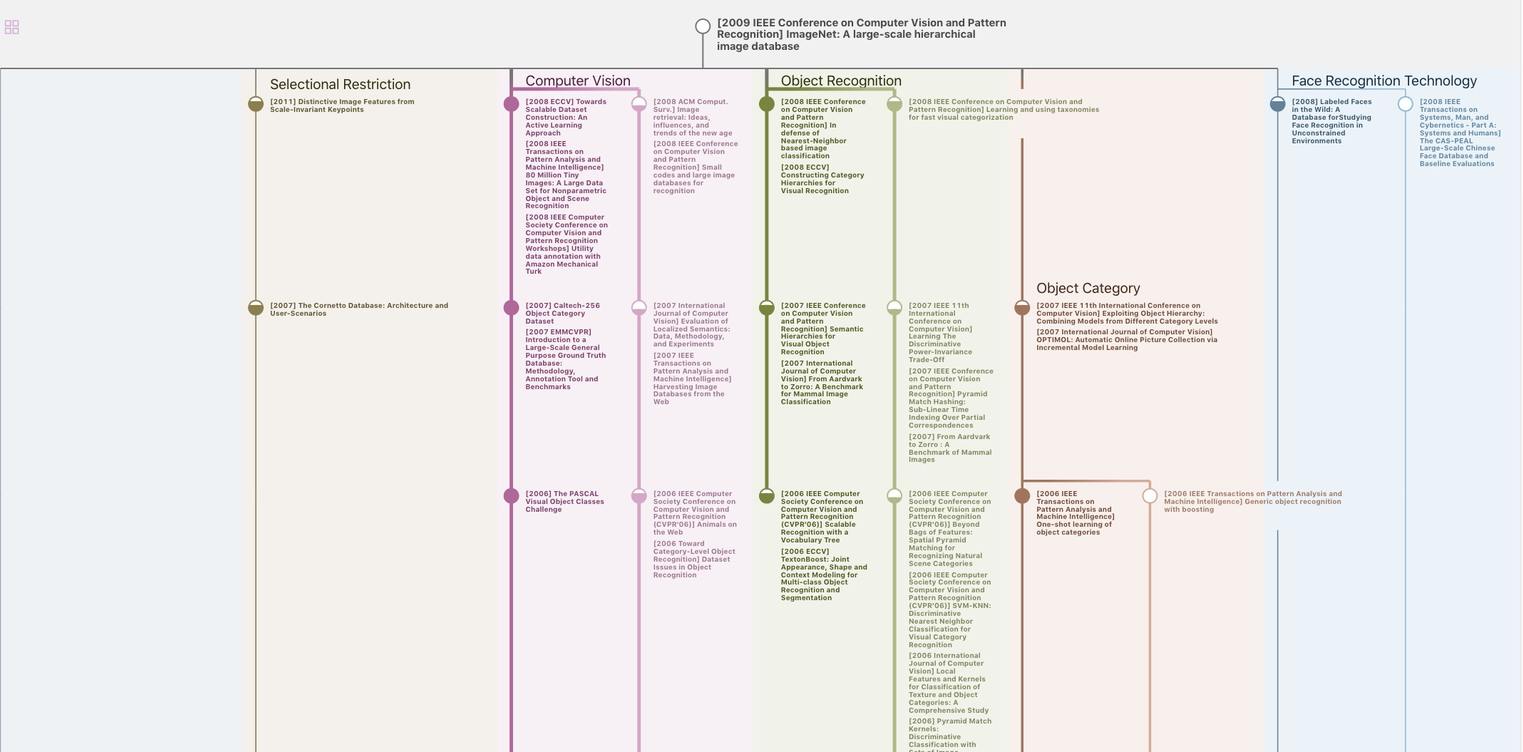
生成溯源树,研究论文发展脉络
Chat Paper
正在生成论文摘要