DAC: Detector-Agnostic Spatial Covariances for Deep Local Features
CoRR(2023)
摘要
Current deep visual local feature detectors do not model the spatial uncertainty of detected features, producing suboptimal results in downstream applications. In this work, we propose two post-hoc covariance estimates that can be plugged into any pretrained deep feature detector: a simple, isotropic covariance estimate that uses the predicted score at a given pixel location, and a full covariance estimate via the local structure tensor of the learned score maps. Both methods are easy to implement and can be applied to any deep feature detector. We show that these covariances are directly related to errors in feature matching, leading to improvements in downstream tasks, including triangulation, solving the perspective-n-point problem and motion-only bundle adjustment. Code is available at https://github.com/javrtg/DAC
更多查看译文
关键词
deep local features,detector-agnostic
AI 理解论文
溯源树
样例
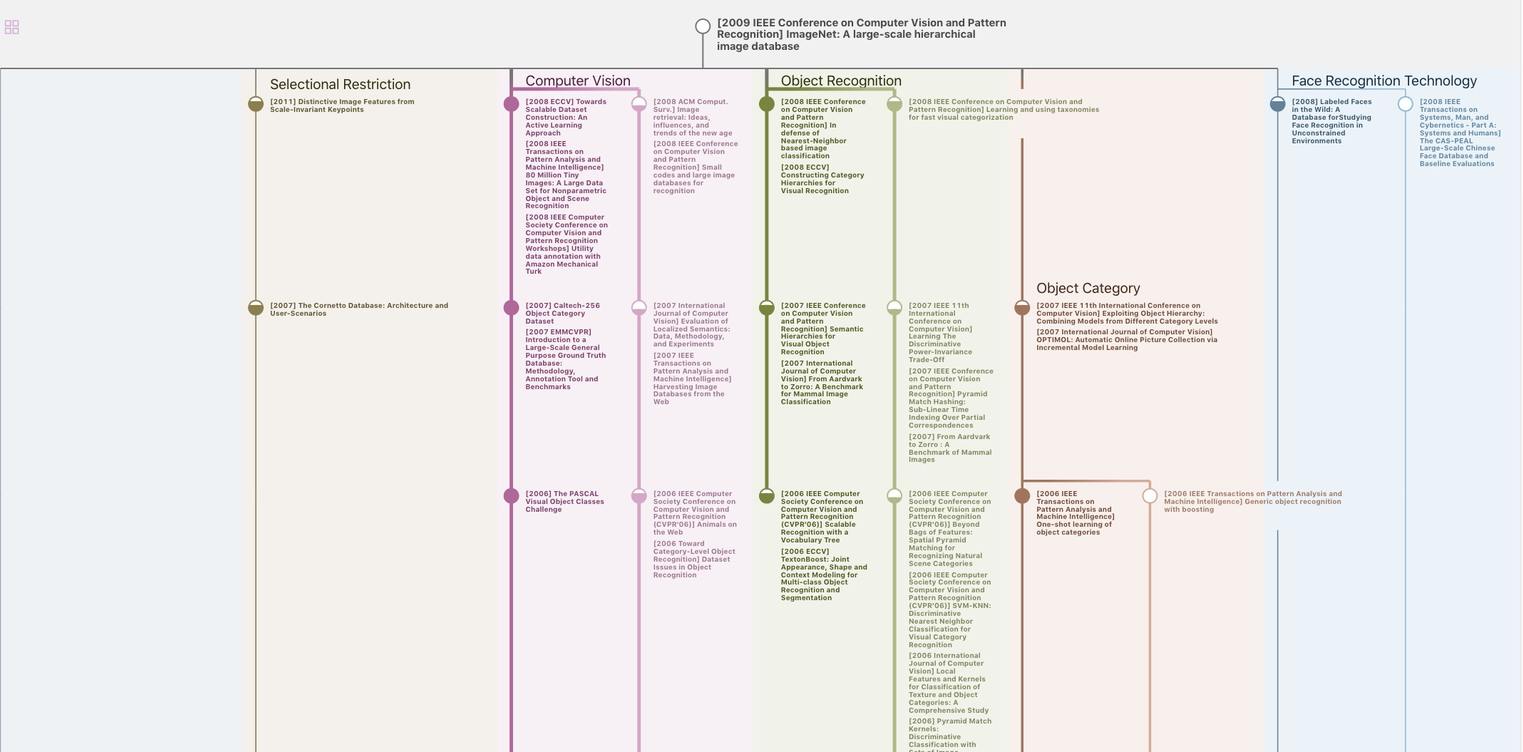
生成溯源树,研究论文发展脉络
Chat Paper
正在生成论文摘要