Transformer Based Semantic Relation Typing for Knowledge Graph Integration
The Semantic Web(2023)
摘要
More and more knowledge graphs (KGs) are generated in various domains. Applications using more than one KG require an integrated view of those KGs, which, in the first place, requires a common schema or ontology. Merging schemas requires not only equivalence mappings between classes but also other semantic relations, like subclass, superclass, etc. In this paper, we introduce TaSeR, a Transformer based model for Semantic Relation Typing, which is able to decide which type of relation holds between two given classes. The approach can differentiate between equivalent class, sub-/superclass, part of/has part, cohyponym, and no relation at all. With the latter outcome, it is not only possible to refine given class alignments, but also filter incorrect correspondences. The models are trained based on examples from general knowledge graphs as well as fine-tuned on the test case at hand. The former models can be directly used to predict a relation without further training. We show that those models are able to outperform other approaches which solve a similar task. For the evaluation, a new measure is introduced which credits for proximal matches.
更多查看译文
关键词
knowledge graph integration,relation,transformer
AI 理解论文
溯源树
样例
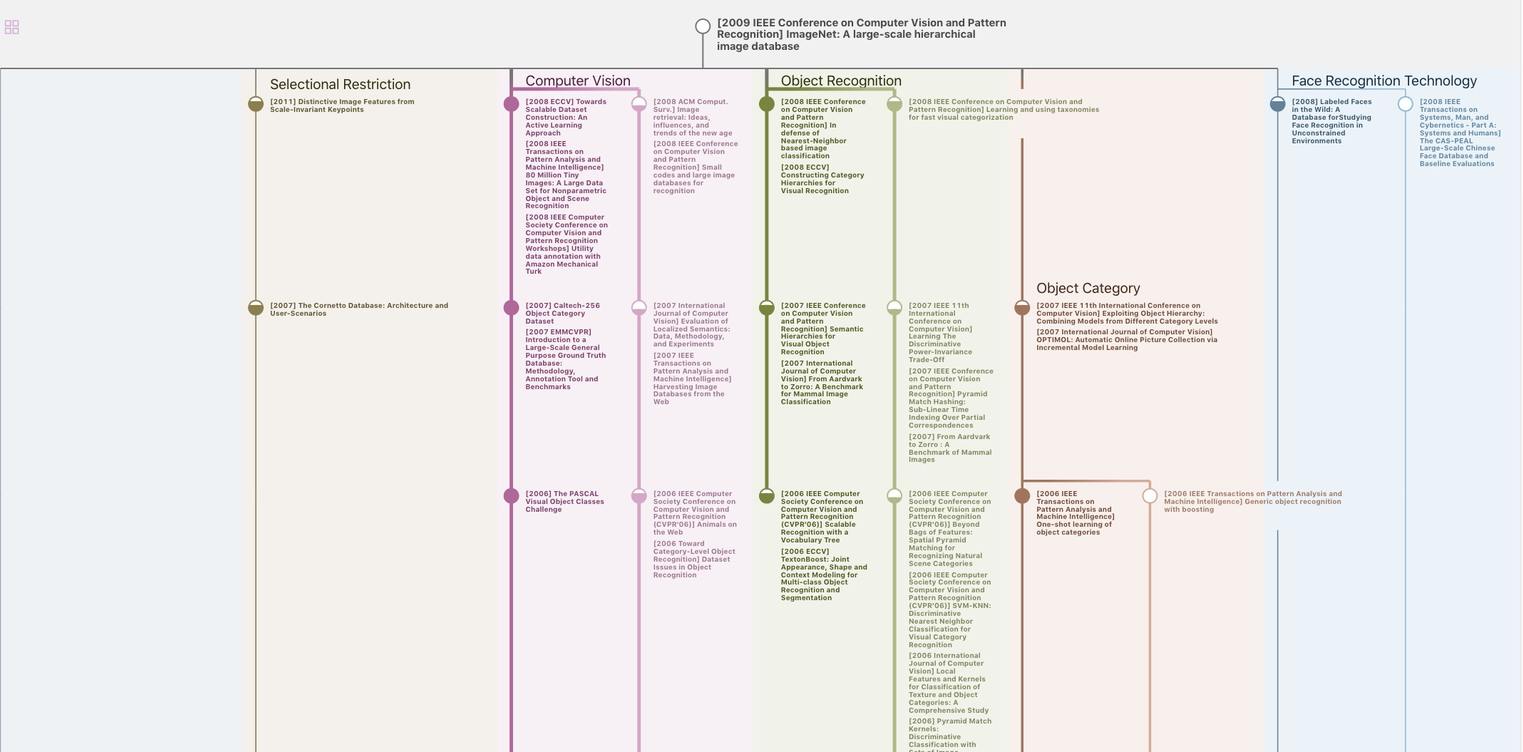
生成溯源树,研究论文发展脉络
Chat Paper
正在生成论文摘要