Radio Galaxy Zoo: Building a multi-purpose foundation model for radio astronomy with self-supervised learning
arxiv(2023)
摘要
In this work, we apply self-supervised learning with instance differentiation to learn a robust, multi-purpose representation for use in radio astronomy. We exceed baseline supervised classification performance by a statistically significant margin for most label volumes in the in-distribution classification case and for all label volumes in the out-of-distribution case, with our model reducing the test set error by up to 5% depending on label volume: from 5% to 2%. Our model is also able to maintain high classification accuracy with very few labels, with only 7.79% error when only using 145 labels. We further demonstrate that by using our foundation model, users can efficiently trade off compute, human labelling cost and test set accuracy according to their respective budgets, allowing for efficient classification in a wide variety of scenarios. Visualizations of our labelled and un-labelled data show that our model's representation space is structured with respect to physical properties of the sources, such as angular source extent. We show that the learned representation is scientifically useful even if no labels are available by performing a similarity search, finding hybrid sources in the RGZ DR1 data-set without any labels. We show that good augmentation design and hyper-parameter choice can help achieve peak performance, while emphasising that optimal hyper-parameters are not required to obtain benefits from self-supervised pre-training.
更多查看译文
AI 理解论文
溯源树
样例
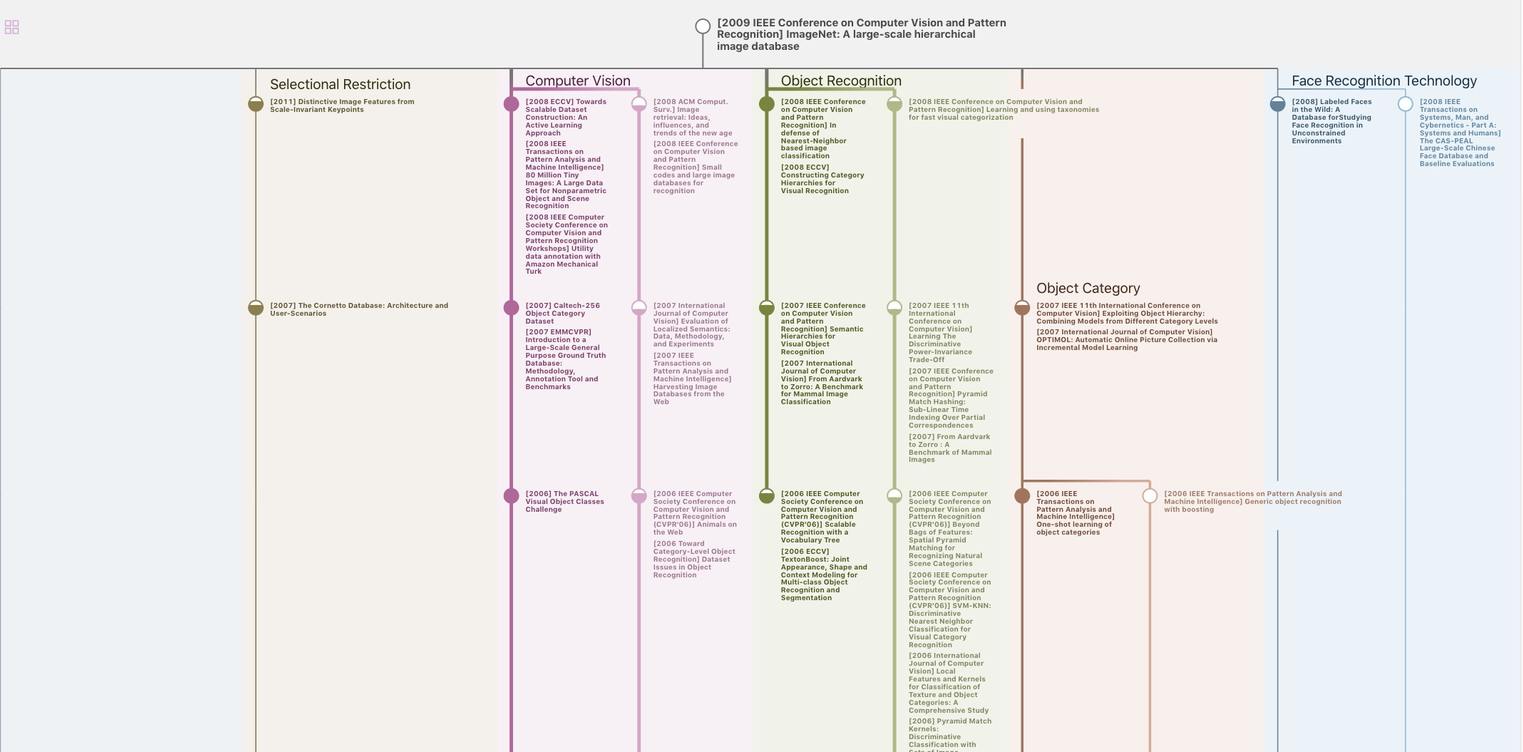
生成溯源树,研究论文发展脉络
Chat Paper
正在生成论文摘要