Learning Preconditioner for Conjugate Gradient PDE Solvers
CoRR(2023)
摘要
Efficient numerical solvers for partial differential equations empower science and engineering. One of the commonly employed numerical solvers is the preconditioned conjugate gradient (PCG) algorithm which can solve large systems to a given precision level. One challenge in PCG solvers is the selection of preconditioners, as different problem-dependent systems can benefit from different preconditioners. We present a new method to introduce \emph{inductive bias} in preconditioning conjugate gradient algorithm. Given a system matrix and a set of solution vectors arise from an underlying distribution, we train a graph neural network to obtain an approximate decomposition to the system matrix to be used as a preconditioner in the context of PCG solvers. We conduct extensive experiments to demonstrate the efficacy and generalizability of our proposed approach in solving various 2D and 3D linear second-order PDEs.
更多查看译文
关键词
preconditioner,learning,gradient
AI 理解论文
溯源树
样例
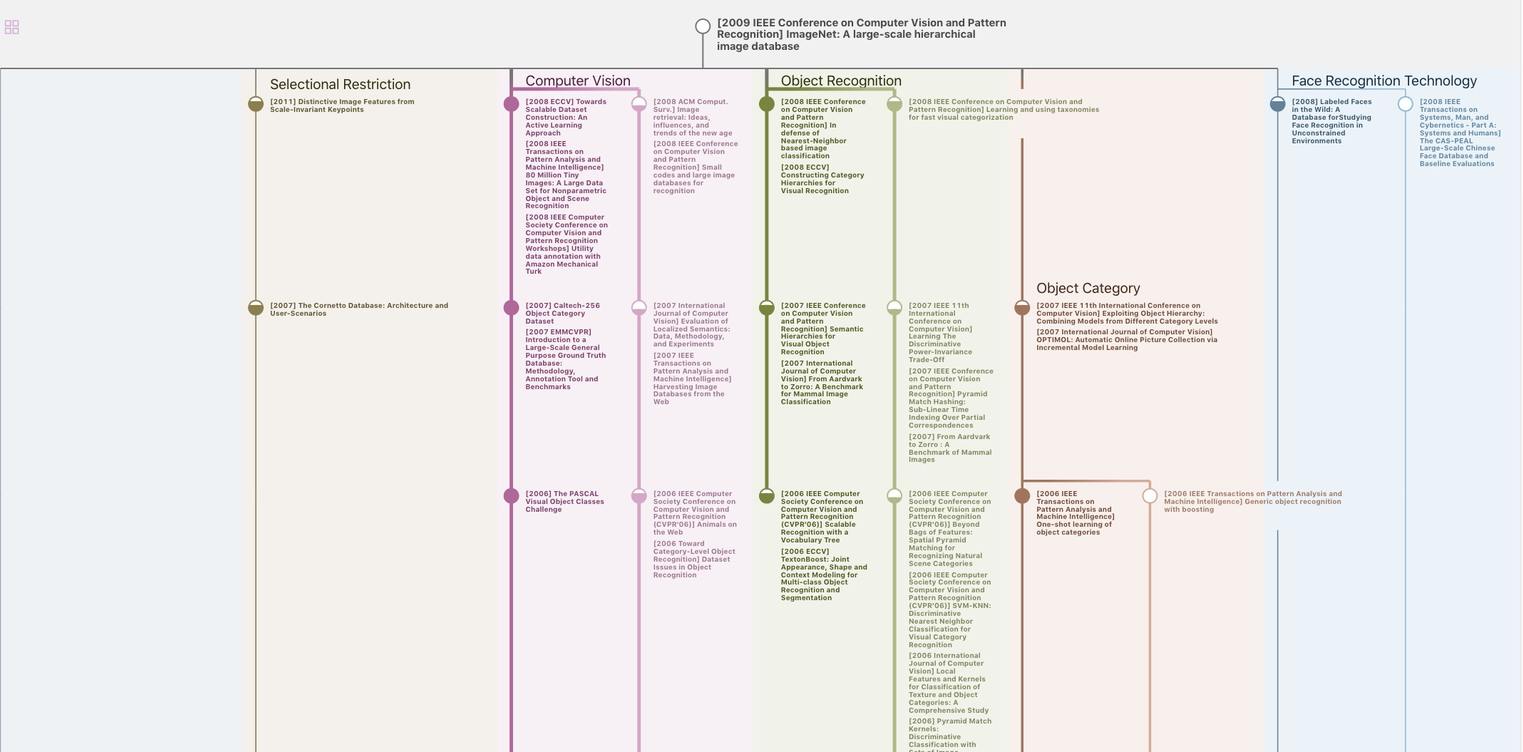
生成溯源树,研究论文发展脉络
Chat Paper
正在生成论文摘要