Markov Aggregation for Speeding Up Agent-Based Movement Simulations
AAMAS '23: Proceedings of the 2023 International Conference on Autonomous Agents and Multiagent Systems(2023)
摘要
In this work, we investigate Markov aggregation for agent-based models (ABMs). Specifically, if the ABM models agent movements on a graph, if its ruleset satisfies certain assumptions, and if the aim is to simulate aggregate statistics such as vertex populations, then the ABM can be replaced by a Markov chain on a comparably small state space. This equivalence between a function of the ABM and a smaller Markov chain allows to reduce the computational complexity of the agent-based simulation from being linear in the number of agents, to being constant in the number of agents and polynomial in the number of locations. We instantiate our theory for a recent ABM for forced migration (Flee). We show that, even though the rulesets of Flee violate some of our necessary assumptions, the aggregated Markov chain-based model, MarkovFlee, achieves comparable accuracy at substantially reduced computational cost. Thus, MarkovFlee can help NGOs and policy makers forecast forced migration in certain conflict scenarios in a cost-effective manner, contributing to fast and efficient delivery of humanitarian relief.
更多查看译文
AI 理解论文
溯源树
样例
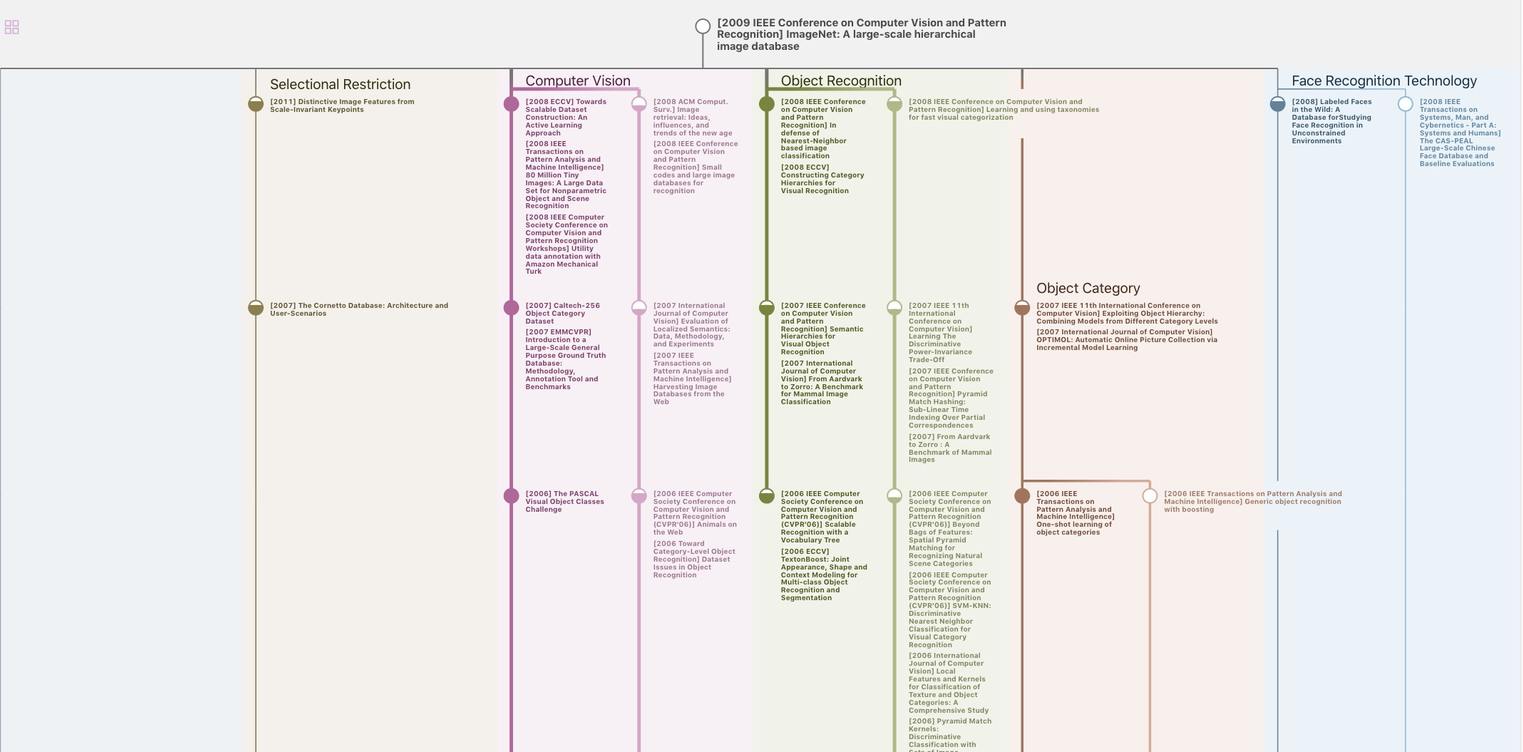
生成溯源树,研究论文发展脉络
Chat Paper
正在生成论文摘要