A decoupled physics-informed neural network for recovering a space-dependent force function in the wave equation from integral overdetermination data
Comput. Appl. Math.(2023)
摘要
In this paper, we study the inverse problem of approximating a space-dependent wave source in a one-dimensional wave equation. The problem is converted to a nonclassical second-order hyperbolic equation and is later reduced to the solution of a linear system of algebraic equations by employing the Ritz collocation method. The obtained Ritz approximation is used to train a physics-informed neural network (PINN). However, unlike the conventional training in PINN, the loss function of the proposed method is not additive and the loss terms are decoupled in the sense that the neural network is re-trained separately according to each loss term. Hence, we name the proposed method decoupled PINN or D-PINN for short. Since the function of the initial condition is nonlinear and continuously differentiable, it is used, up to a scalar, as the activation function as opposed to using an off-the-shelf activation function such as hyperbolic tangent. Experiments indicate that D-PINN is capable of approximating the solution more accurately than Ritz and PINN.
更多查看译文
关键词
Physics-informed neural networks,Inverse wave problem,Ritz approximation
AI 理解论文
溯源树
样例
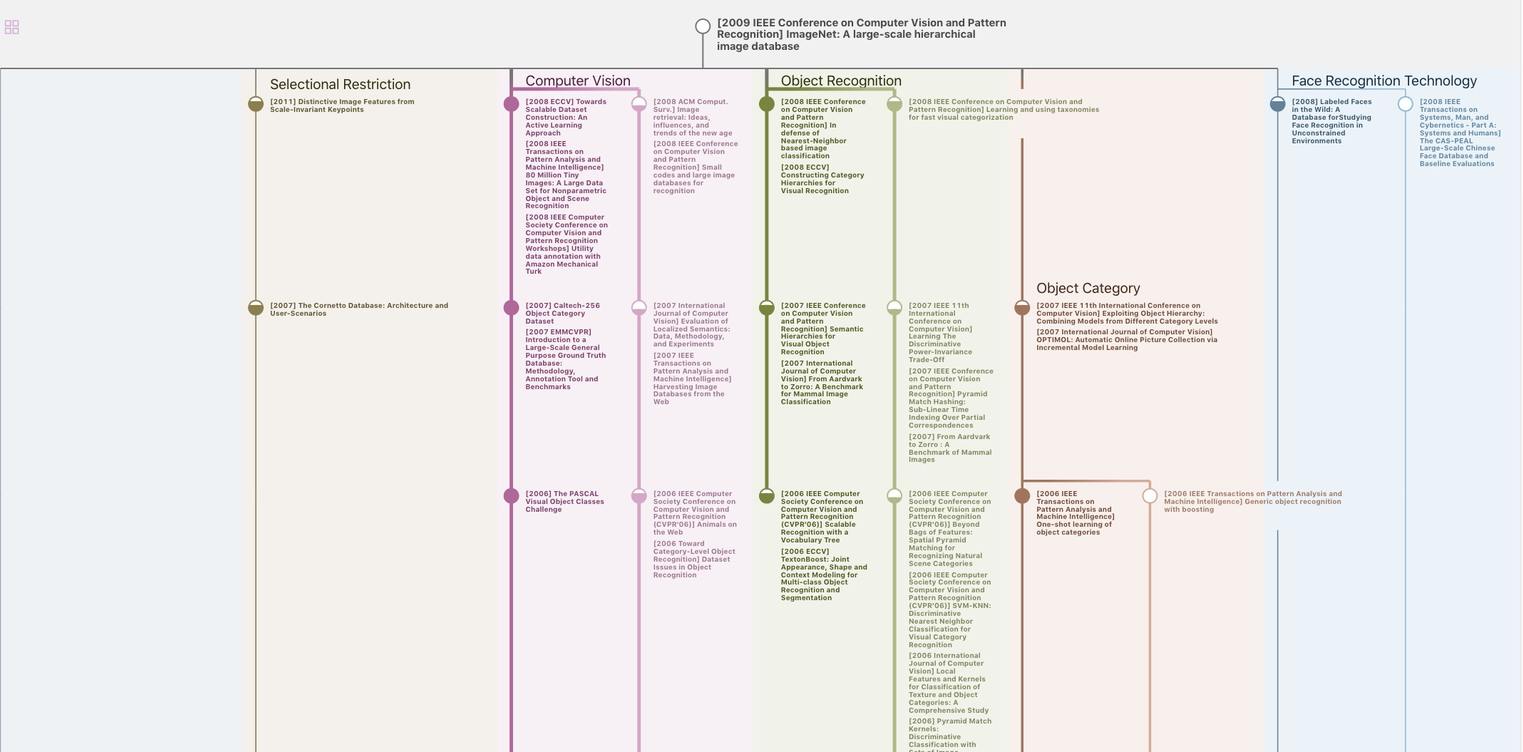
生成溯源树,研究论文发展脉络
Chat Paper
正在生成论文摘要