JobXMLC: EXtreme Multi-Label Classification of Job Skills with Graph Neural Networks
17TH CONFERENCE OF THE EUROPEAN CHAPTER OF THE ASSOCIATION FOR COMPUTATIONAL LINGUISTICS, EACL 2023(2023)
摘要
Writing a good job description is an important step in the online recruitment process to hire the best candidates. Most recruiters forget to include some relevant skills in the job description. These missing skills affect the performance of recruitment tasks such as job suggestions, job search, candidate recommendations, etc. Existing approaches are limited to contextual modelling, do not exploit inter-relational structures like job-job and job-skill relationships, and are not scalable. In this paper, we exploit these structural relationships using a graph-based approach. We propose a novel skill prediction framework called JobXMLC, which uses graph neural networks with skill attention to predict missing skills using job descriptions. JobXMLC enables joint learning over a job-skill graph consisting of 22.8K entities (jobs and skills) and 650K relationships. We experiment with real-world recruitment datasets to evaluate our proposed approach. We train JobXMLC on 20, 298 jobs and 2, 548 skills within 30 minutes on a single GPU machine. JobXMLC outperforms the state-of-the-art approaches by 6% on precision and 3% on recall. JobXMLC is 18X faster for training tasks and up to 634X faster in skill prediction on benchmark datasets enabling JobXMLC to scale up on larger datasets. We have made our code and dataset public at https://precog.iiit.ac.in/resources.html.
更多查看译文
AI 理解论文
溯源树
样例
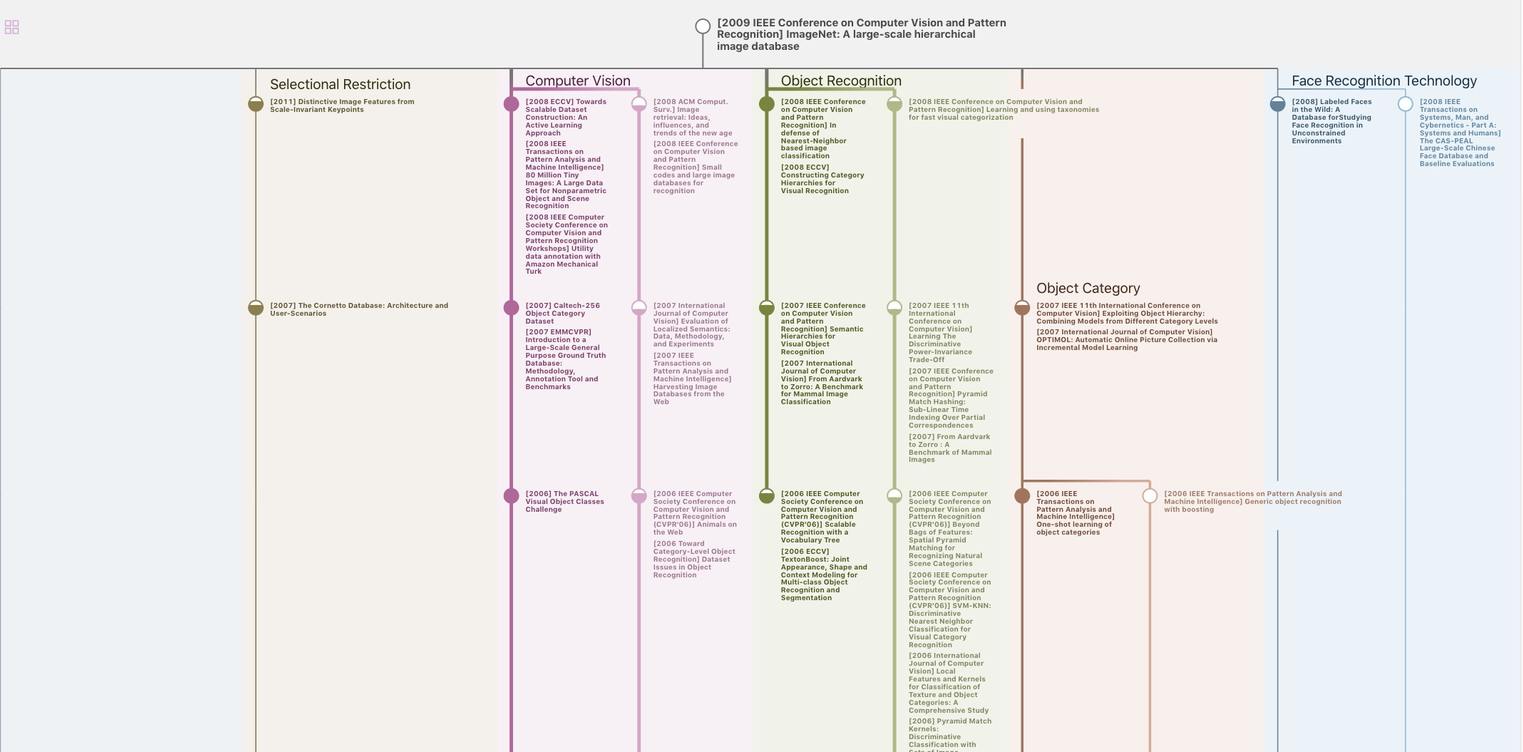
生成溯源树,研究论文发展脉络
Chat Paper
正在生成论文摘要