Neural Ideal Large Eddy Simulation: Modeling Turbulence with Neural Stochastic Differential Equations
NeurIPS(2023)
摘要
We introduce a data-driven learning framework that assimilates two powerful ideas: ideal large eddy simulation (LES) from turbulence closure modeling and neural stochastic differential equations (SDE) for stochastic modeling. The ideal LES models the LES flow by treating each full-order trajectory as a random realization of the underlying dynamics, as such, the effect of small-scales is marginalized to obtain the deterministic evolution of the LES state. However, ideal LES is analytically intractable. In our work, we use a latent neural SDE to model the evolution of the stochastic process and an encoder-decoder pair for transforming between the latent space and the desired ideal flow field. This stands in sharp contrast to other types of neural parameterization of closure models where each trajectory is treated as a deterministic realization of the dynamics. We show the effectiveness of our approach (niLES - neural ideal LES) on a challenging chaotic dynamical system: Kolmogorov flow at a Reynolds number of 20,000. Compared to competing methods, our method can handle non-uniform geometries using unstructured meshes seamlessly. In particular, niLES leads to trajectories with more accurate statistics and enhances stability, particularly for long-horizon rollouts.
更多查看译文
关键词
turbulence,stochastic differential equations,simulation
AI 理解论文
溯源树
样例
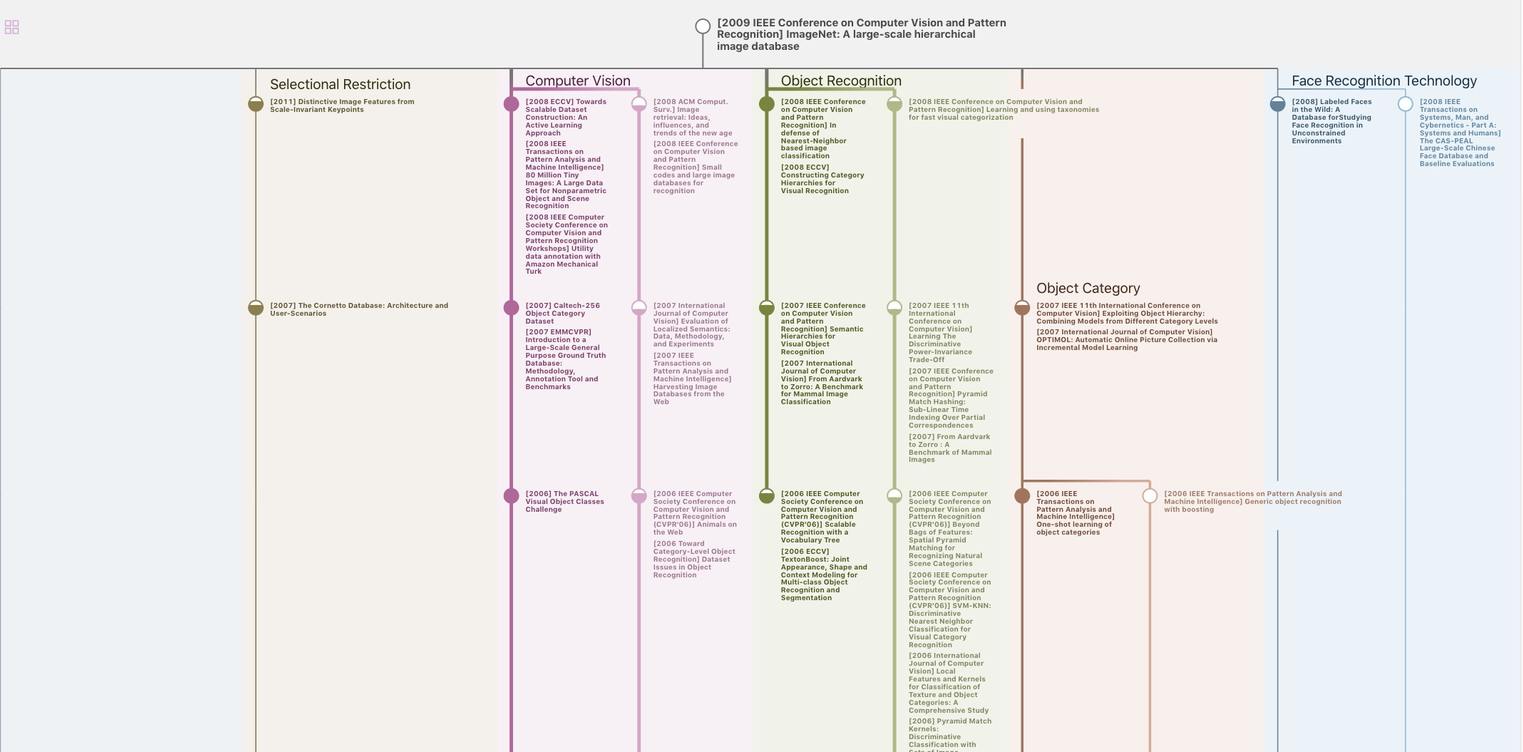
生成溯源树,研究论文发展脉络
Chat Paper
正在生成论文摘要