Exploring Global and Local Information for Anomaly Detection with Normal Samples
CoRR(2023)
摘要
Anomaly detection aims to detect data that do not conform to regular patterns, and such data is also called outliers. The anomalies to be detected are often tiny in proportion, containing crucial information, and are suitable for application scenes like intrusion detection, fraud detection, fault diagnosis, e-commerce platforms, et al. However, in many realistic scenarios, only the samples following normal behavior are observed, while we can hardly obtain any anomaly information. To address such problem, we propose an anomaly detection method GALDetector which is combined of global and local information based on observed normal samples. The proposed method can be divided into a three-stage method. Firstly, the global similar normal scores and the local sparsity scores of unlabeled samples are computed separately. Secondly, potential anomaly samples are separated from the unlabeled samples corresponding to these two scores and corresponding weights are assigned to the selected samples. Finally, a weighted anomaly detector is trained by loads of samples, then the detector is utilized to identify else anomalies. To evaluate the effectiveness of the proposed method, we conducted experiments on three categories of real-world datasets from diverse domains, and experimental results show that our method achieves better performance when compared with other state-of-the-art methods.
更多查看译文
关键词
Local Information,Normal Samples,Global Information,Anomaly Detection,Global Score,Scores In Sample,Real-world Datasets,Normal Scores,Intrusion Detection,Categories In Dataset,Fraud Detection,Anomaly Detection Methods,Potential Anomalies,Evaluation Criteria,K-nearest Neighbor,Unsupervised Methods,Normal Pattern,Hyperplane,Root Node,Tree Nodes,Abnormal Samples,One-class Classification,Cluster Centers,Difference In Direction,Nearest Center,Semi-supervised Methods,Partial Identification,Label Information,Node Distance,Low-dimensional Space
AI 理解论文
溯源树
样例
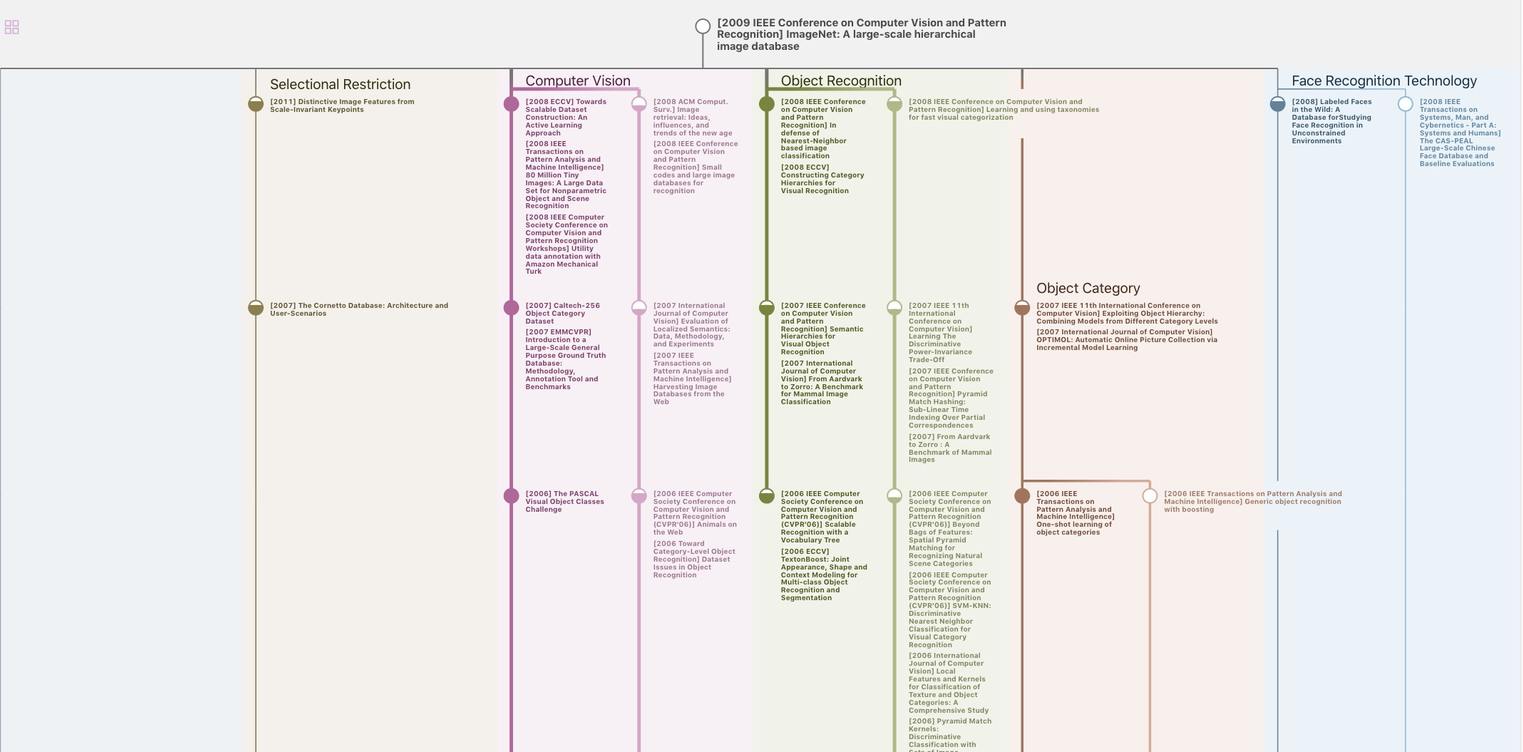
生成溯源树,研究论文发展脉络
Chat Paper
正在生成论文摘要