Reference Matters: Benchmarking Factual Error Correction for Dialogue Summarization with Fine-grained Evaluation Framework
PROCEEDINGS OF THE 61ST ANNUAL MEETING OF THE ASSOCIATION FOR COMPUTATIONAL LINGUISTICS (ACL 2023): LONG PAPERS, VOL 1(2023)
摘要
Factuality is important to dialogue summarization. Factual error correction (FEC) of model-generated summaries is one way to improve factuality. Current FEC evaluation that relies on factuality metrics is not reliable and detailed enough. To address this problem, we are the first to manually annotate a FEC dataset for dialogue summarization containing 4000 items and propose FERRANTI, a fine-grained evaluation framework based on reference correction that automatically evaluates the performance of FEC models on different error categories. Using this evaluation framework, we conduct sufficient experiments with FEC approaches under a variety of settings and find the best training modes and significant differences in the performance of the existing approaches on different factual error categories.
更多查看译文
AI 理解论文
溯源树
样例
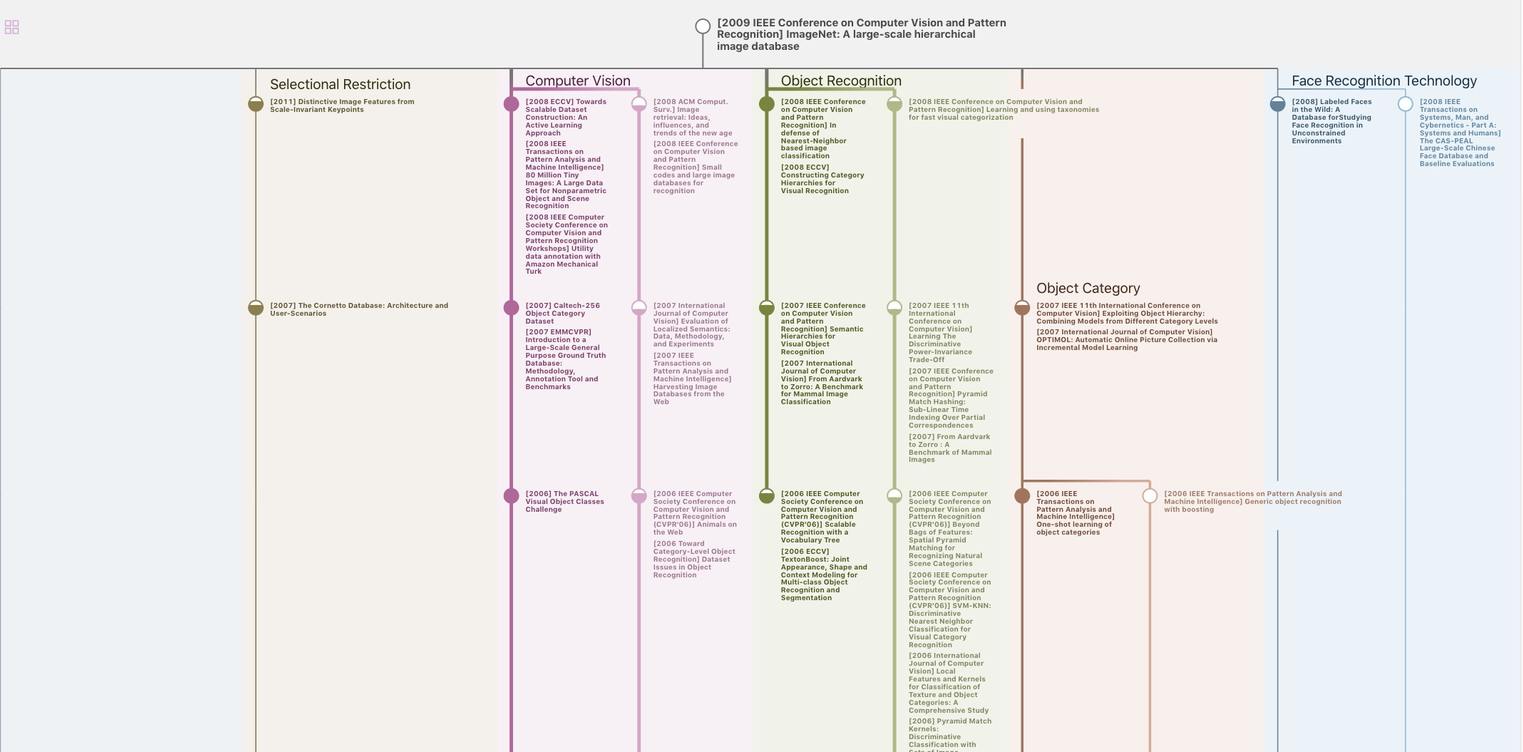
生成溯源树,研究论文发展脉络
Chat Paper
正在生成论文摘要