Bayesian Inverse Contextual Reasoning for Heterogeneous Semantics- Native Communication
IEEE TRANSACTIONS ON COMMUNICATIONS(2024)
摘要
This work deals with a heterogeneous semantics-native communication (SNC) problem. When agents do not share the same communication context, the effectiveness of contextual reasoning (CR) is compromised calling for agents to infer other agents' context before communication. This article proposes a novel framework for solving the inverse problem of CR in SNC using two Bayesian inference methods, namely: Bayesian inverse CR (iCR) and Bayesian inverse linearized CR (iLCR). The first proposed Bayesian iCR method utilizes Markov Chain Monte Carlo (MCMC) sampling to infer the agent's context while being computationally expensive. To address this issue, a Bayesian iLCR method is leveraged which obtains a linearized CR (LCR) model by training a linear neural network. Experimental results show that the Bayesian iLCR method requires less computation and achieves higher inference accuracy compared to Bayesian iCR. Additionally, heterogeneous SNC based on the context obtained through the Bayesian iLCR method shows better communication effectiveness than that of Bayesian iCR. Overall, this work provides valuable insights and methods to improve the effectiveness of SNC in situations where agents have different contexts.
更多查看译文
关键词
Bayes methods,Semantics,Computational modeling,Task analysis,Context modeling,Cognition,Training,Semantic communication,semantics-native communication,contextual reasoning,inverse contextual reasoning
AI 理解论文
溯源树
样例
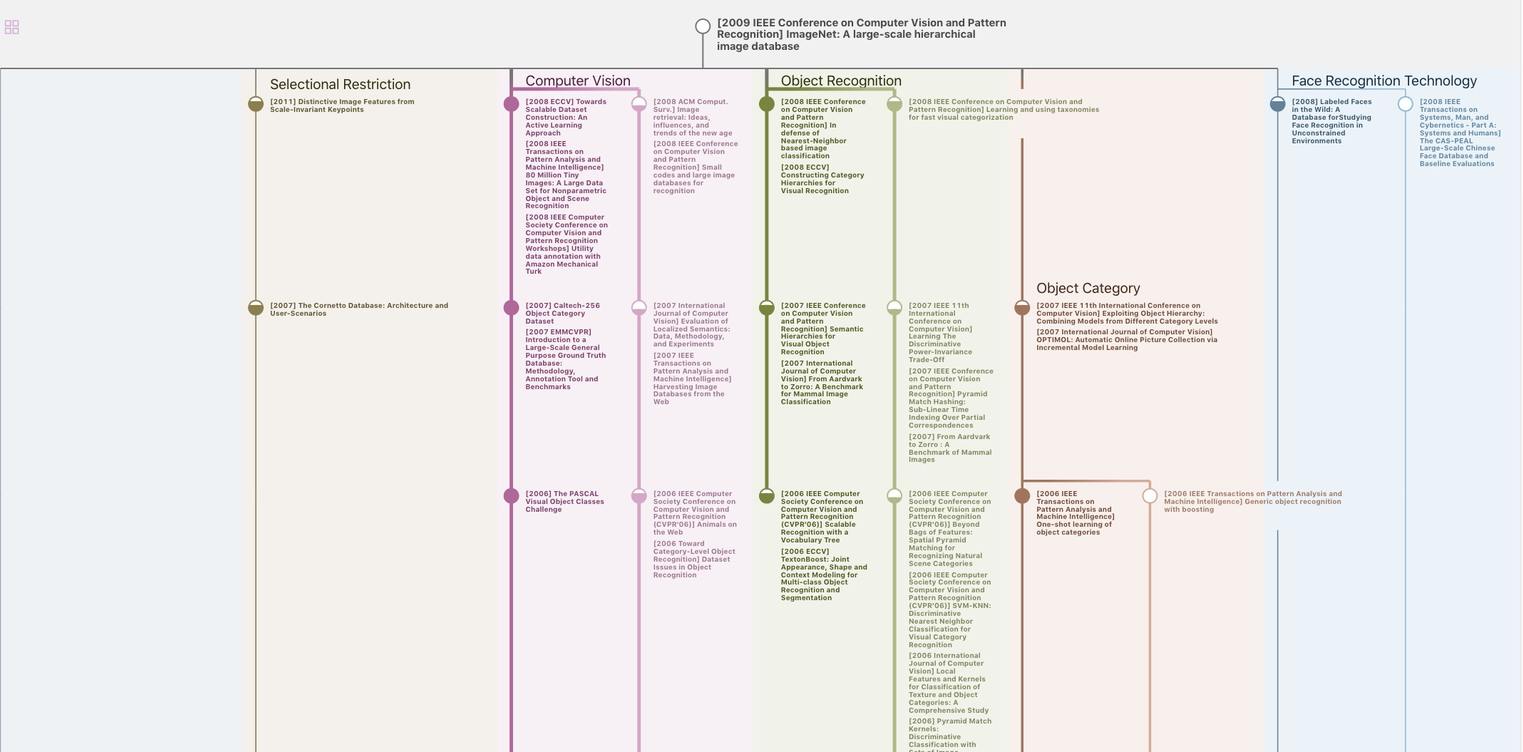
生成溯源树,研究论文发展脉络
Chat Paper
正在生成论文摘要