AFSPNet: an adaptive feature selection pyramid network for efficient object detection in remote sensing images
Journal of Applied Remote Sensing(2022)
摘要
In recent studies, remote sensing object detection methods based on deep learning have emerged as a primary concern in environmental monitoring, military investigation, and hazard response. However, many difficulties, such as complex backgrounds, dense target quantities, large-scale variations, and non-uniform distribution, lead to many parameters and complex network structures, thus limiting the accuracy of the detector and slowing the inference speed. To address these issues, we propose a lightweight and efficient object detector for remote sensing images. First, an asymmetric convolution with the visual attention mechanism is reconstructed to decrease the complexity and strengthen the feature representation ability. Then, an adaptive feature selection structure is designed to extract discriminative feature information, which can adaptively model the shapes of objects by introducing deformable convolution to obtain a stronger geometric feature representation. To reduce information loss across different channels and spatial locations, a hybrid receptive field module is also proposed to increase the receptive field model by mixing dilated convolutional layers with different dilation rates. Finally, experimental results on the DIOR dataset show that our approach significantly improves detection accuracy and running speed. (c) 2022 Society of Photo-Optical Instrumentation Engineers (SPIE)
更多查看译文
关键词
remote sensing,efficient object detection,afspnet,feature selection
AI 理解论文
溯源树
样例
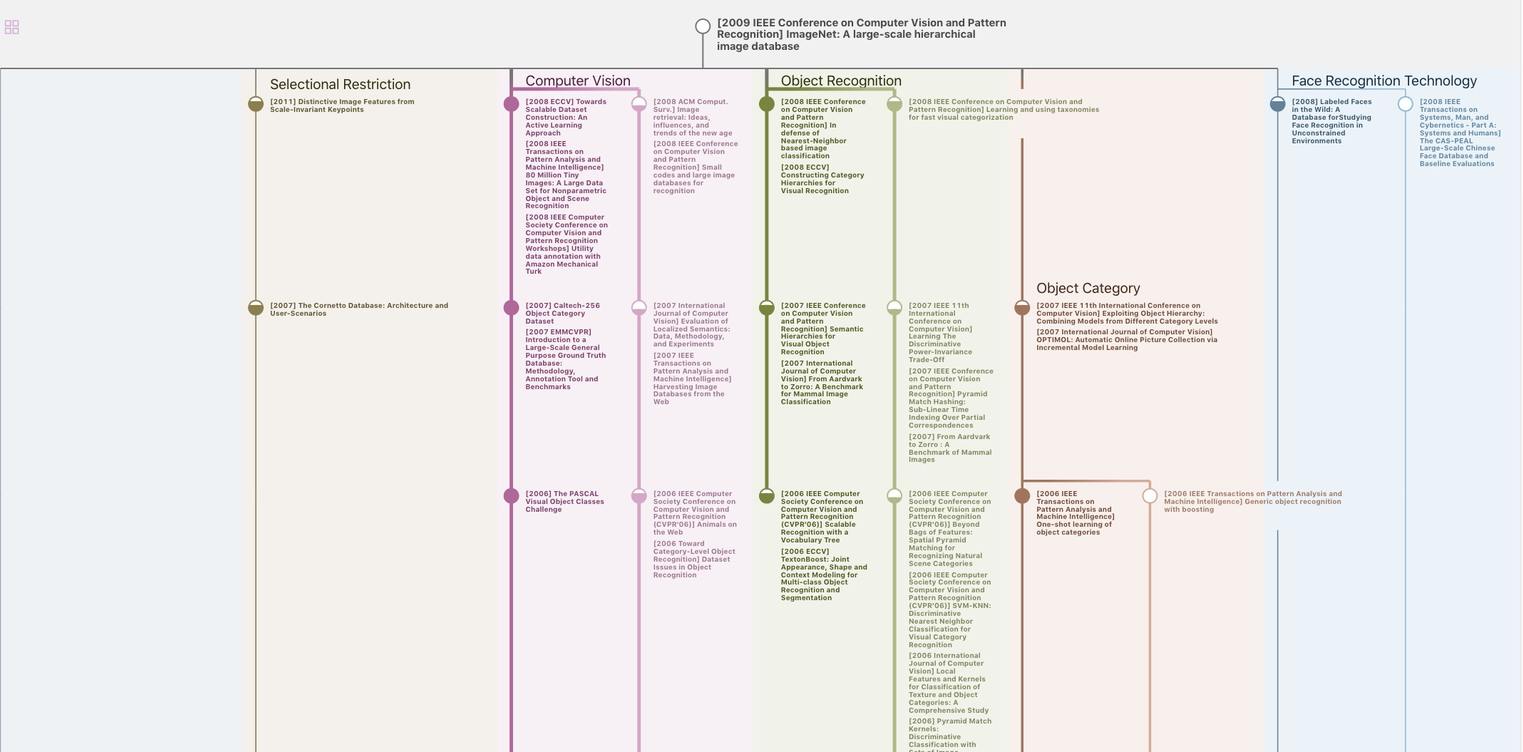
生成溯源树,研究论文发展脉络
Chat Paper
正在生成论文摘要