StARformer: Transformer with State-Action-Reward Representations for Robot Learning
IEEE Transactions on Pattern Analysis and Machine Intelligence(2022)
摘要
Reinforcement Learning (RL) can be considered as a sequence modeling task, where an agent employs a sequence of past state-action-reward experiences to predict a sequence of future actions. In this work, we propose State-Action-Reward Transformer (StARformer), a Transformer architecture for robot learning with image inputs, which explicitly models short-term state-action-reward representations (StAR-representations), essentially introducing a Markovian-like inductive bias to improve long-term modeling. StARformer first extracts StAR-representations using self-attending patches of image states, action, and reward tokens within a short temporal window. These StAR-representations are combined with pure image state representations, extracted as convolutional features, to perform self-attention over the whole sequence. Our experimental results show that StARformer outperforms the state-of-the-art Transformer-based method on image-based Atari and DeepMind Control Suite benchmarks, under both offline-RL and imitation learning settings. We find that models can benefit from our combination of patch-wise and convolutional image embeddings. StARformer is also more compliant with longer sequences of inputs than the baseline method. Finally, we demonstrate how StARformer can be successfully applied to a real-world robot imitation learning setting via a human-following task.
更多查看译文
关键词
robot learning,transformer,state-action-reward
AI 理解论文
溯源树
样例
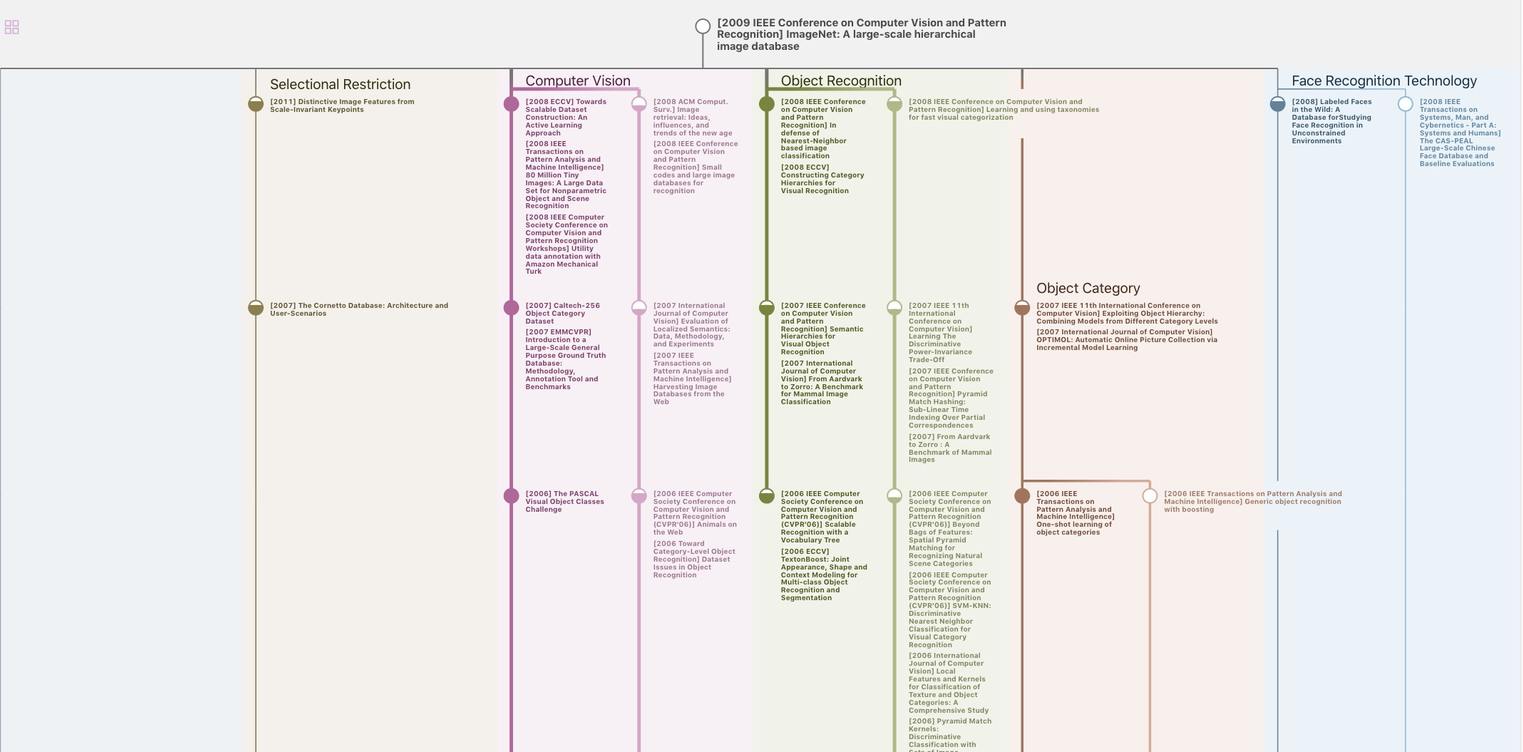
生成溯源树,研究论文发展脉络
Chat Paper
正在生成论文摘要