Opportunities and Risks of LLMs for Scalable Deliberation with Polis
CoRR(2023)
摘要
Polis is a platform that leverages machine intelligence to scale up deliberative processes. In this paper, we explore the opportunities and risks associated with applying Large Language Models (LLMs) towards challenges with facilitating, moderating and summarizing the results of Polis engagements. In particular, we demonstrate with pilot experiments using Anthropic's Claude that LLMs can indeed augment human intelligence to help more efficiently run Polis conversations. In particular, we find that summarization capabilities enable categorically new methods with immense promise to empower the public in collective meaning-making exercises. And notably, LLM context limitations have a significant impact on insight and quality of these results. However, these opportunities come with risks. We discuss some of these risks, as well as principles and techniques for characterizing and mitigating them, and the implications for other deliberative or political systems that may employ LLMs. Finally, we conclude with several open future research directions for augmenting tools like Polis with LLMs.
更多查看译文
关键词
scalable deliberation,llms,polis
AI 理解论文
溯源树
样例
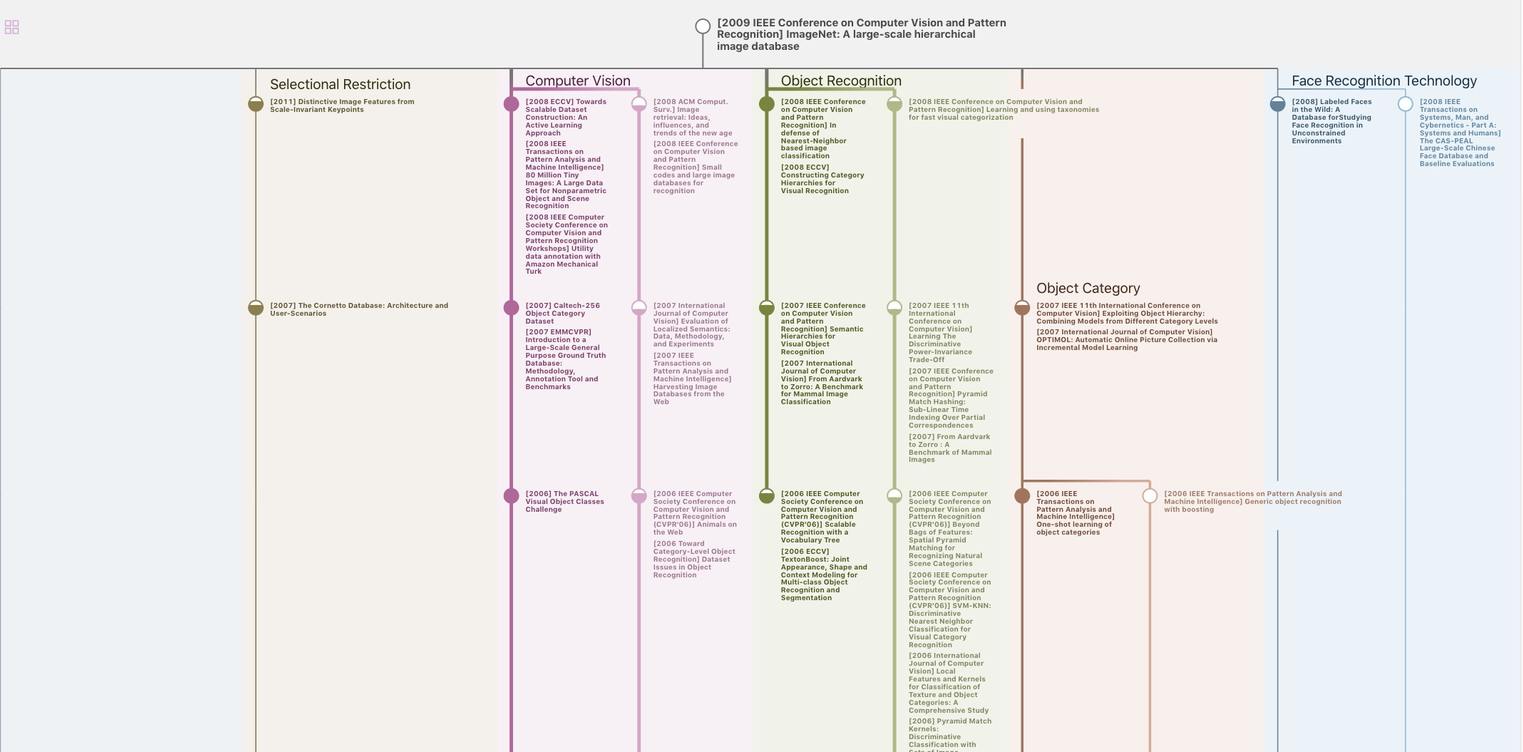
生成溯源树,研究论文发展脉络
Chat Paper
正在生成论文摘要