Weakly supervised point cloud segmentation via deep morphological semantic information embedding
CAAI TRANSACTIONS ON INTELLIGENCE TECHNOLOGY(2023)
摘要
Segmenting the semantic regions of point clouds is a crucial step for intelligent agents to understand 3D scenes. Weakly supervised point cloud segmentation is highly desirable because entirely labelling point clouds is highly time-consuming and costly. For the low-costing labelling of 3D point clouds, the scene-level label is one of the most effortless label strategies. However, due to the limitation of classifier discriminative capability and the orderless and structurless nature of the point cloud data, existing scene-level method is hard to transfer the semantic information, which usually leads to the under-activated or over-activated issues. To this end, a local semantic embedding network is introduced to learn local structural patterns and semantic propagation. Specifically, the proposed network contains graph convolution-based dilation and erosion embedding modules to implement 'inside-out' and 'outside-in' semantic information dissemination pathways. Therefore, the proposed weakly supervised learning framework could achieve the mutual propagation of semantic information in the foreground and background. Comprehensive experiments on the widely used ScanNet benchmark demonstrate the superior capacity of the proposed approach when compared to the current alternatives and baseline models.
更多查看译文
关键词
artificial intelligence, computer vision, deep learning
AI 理解论文
溯源树
样例
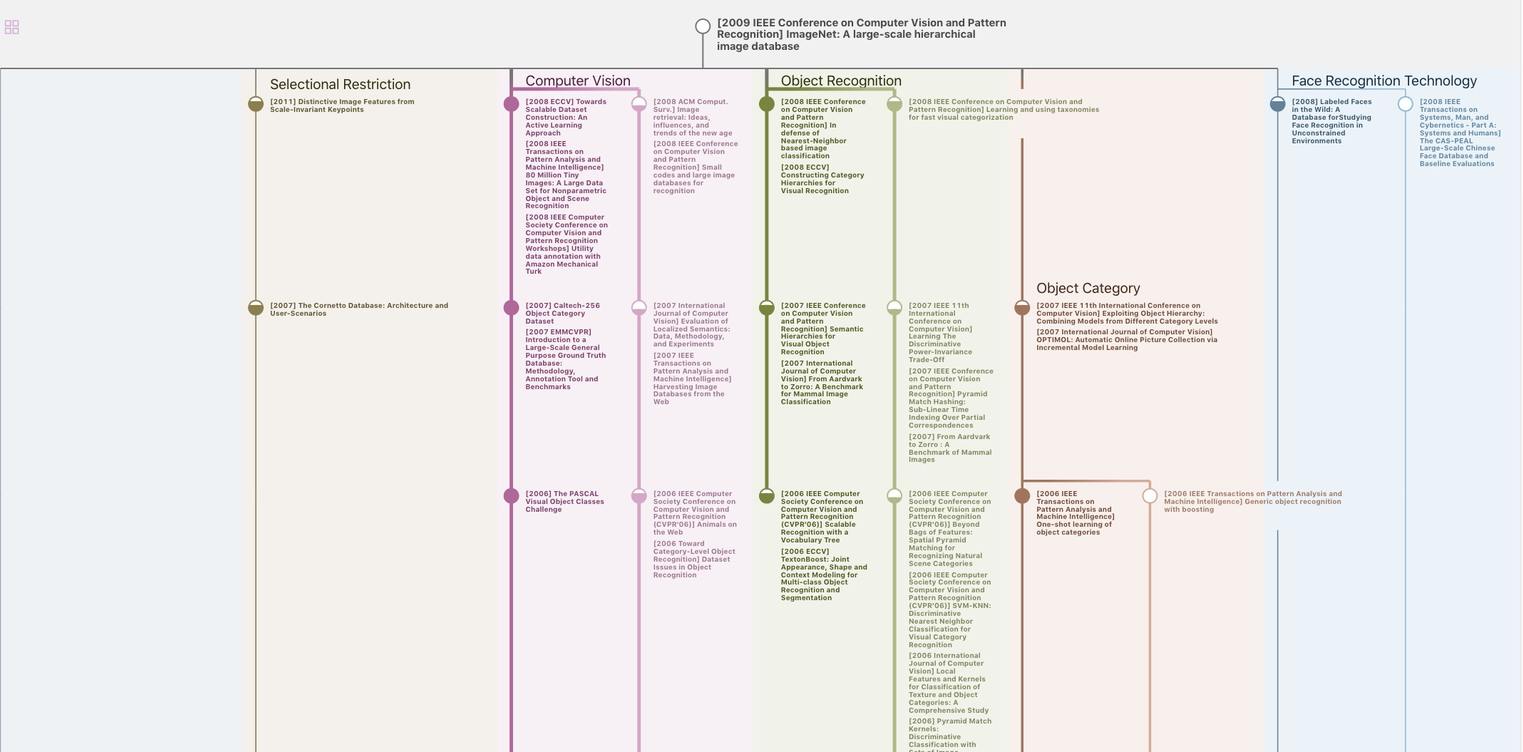
生成溯源树,研究论文发展脉络
Chat Paper
正在生成论文摘要