Multi-level semantic information guided image generation for few-shot steel surface defect classification
FRONTIERS IN PHYSICS(2023)
摘要
Surface defect classification is one of key points in the field of steel manufacturing. It remains challenging primarily due to the rare occurrence of defect samples and the similarity between different defects. In this paper, a multi-level semantic method based on residual adversarial learning with Wasserstein divergence is proposed to realize sample augmentation and automatic classification of various defects simultaneously. Firstly, the residual module is introduced into model structure of adversarial learning to optimize the network structure and effectively improve the quality of samples generated by model. By substituting original classification layer with multiple convolution layers in the network framework, the feature extraction capability of model is further strengthened, enhancing the classification performance of model. Secondly, in order to better capture different semantic information, we design a multi-level semantic extractor to extract rich and diverse semantic features from real-world images to efficiently guide sample generation. In addition, the Wasserstein divergence is introduced into the loss function to effectively solve the problem of unstable network training. Finally, high-quality defect samples can be generated through adversarial learning, effectively expanding the limited training samples for defect classification. The experimental results substantiate that our proposed method can not only generate high-quality defect samples, but also accurately achieve the classification of defect detection samples.
更多查看译文
关键词
few-shot steel surface defect classification, adversarial learning, residual module, multi-level semantic feature extractor, Wasserstein divergence
AI 理解论文
溯源树
样例
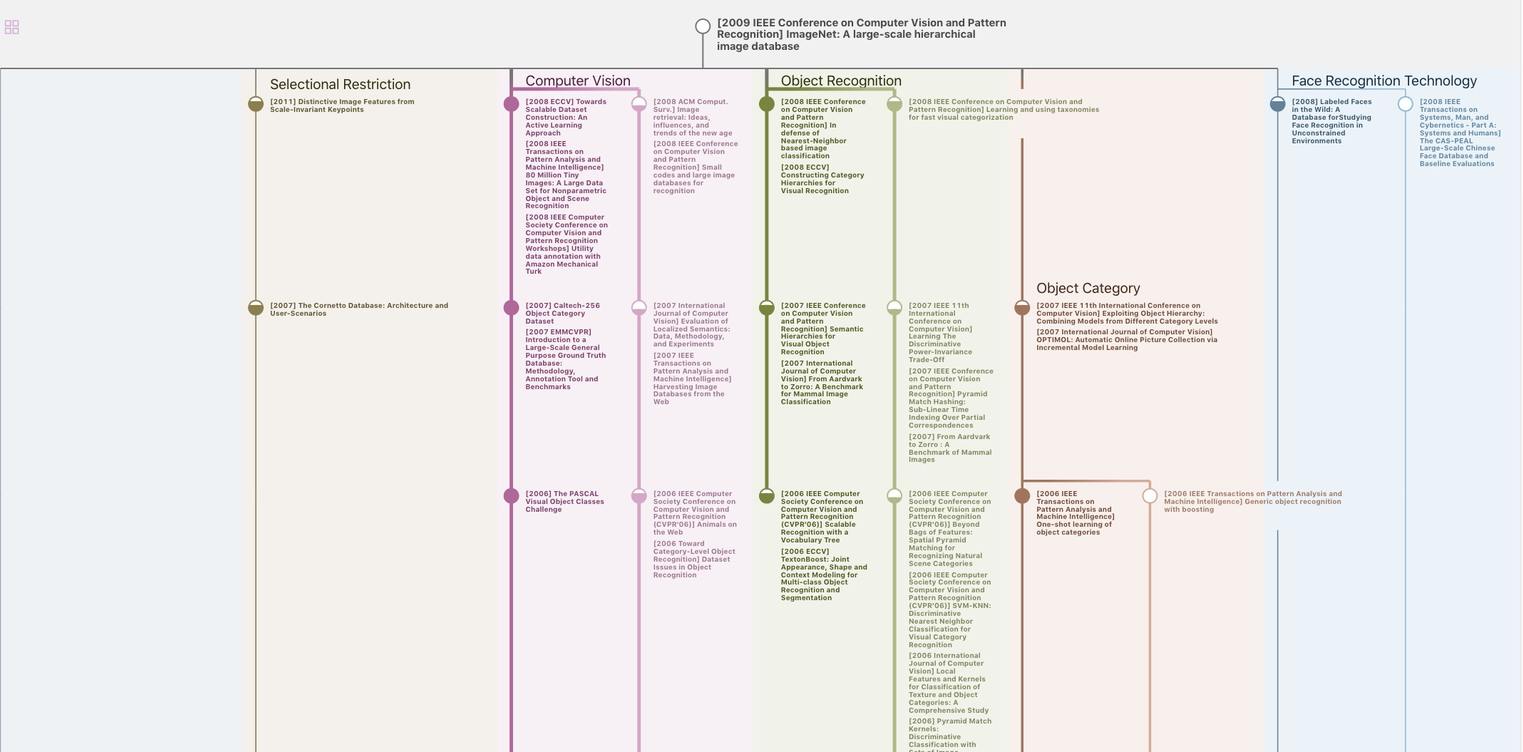
生成溯源树,研究论文发展脉络
Chat Paper
正在生成论文摘要