Quantifying disorder one atom at a time using an interpretable graph neural network paradigm
Nature Communications(2023)
摘要
Quantifying the level of atomic disorder within materials is critical to understanding how evolving local structural environments dictate performance and durability. Here, we leverage graph neural networks to define a physically interpretable metric for local disorder, called SODAS. This metric encodes the diversity of the local atomic configurations as a continuous spectrum between the solid and liquid phases, quantified against a distribution of thermal perturbations. We apply this methodology to four prototypical examples with varying levels of disorder: (1) grain boundaries, (2) solid-liquid interfaces, (3) polycrystalline microstructures, and (4) tensile failure/fracture. We also compare SODAS to several commonly used methods. Using elemental aluminum as a case study, we show how our paradigm can track the spatio-temporal evolution of interfaces, incorporating a mathematically defined description of the spatial boundary between order and disorder. We further show how to extract physics-preserved gradients from our continuous disorder fields, which may be used to understand and predict materials performance and failure. Overall, our framework provides a simple and generalizable pathway to quantify the relationship between complex local atomic structure and coarse-grained materials phenomena.
更多查看译文
关键词
interpretable graph
AI 理解论文
溯源树
样例
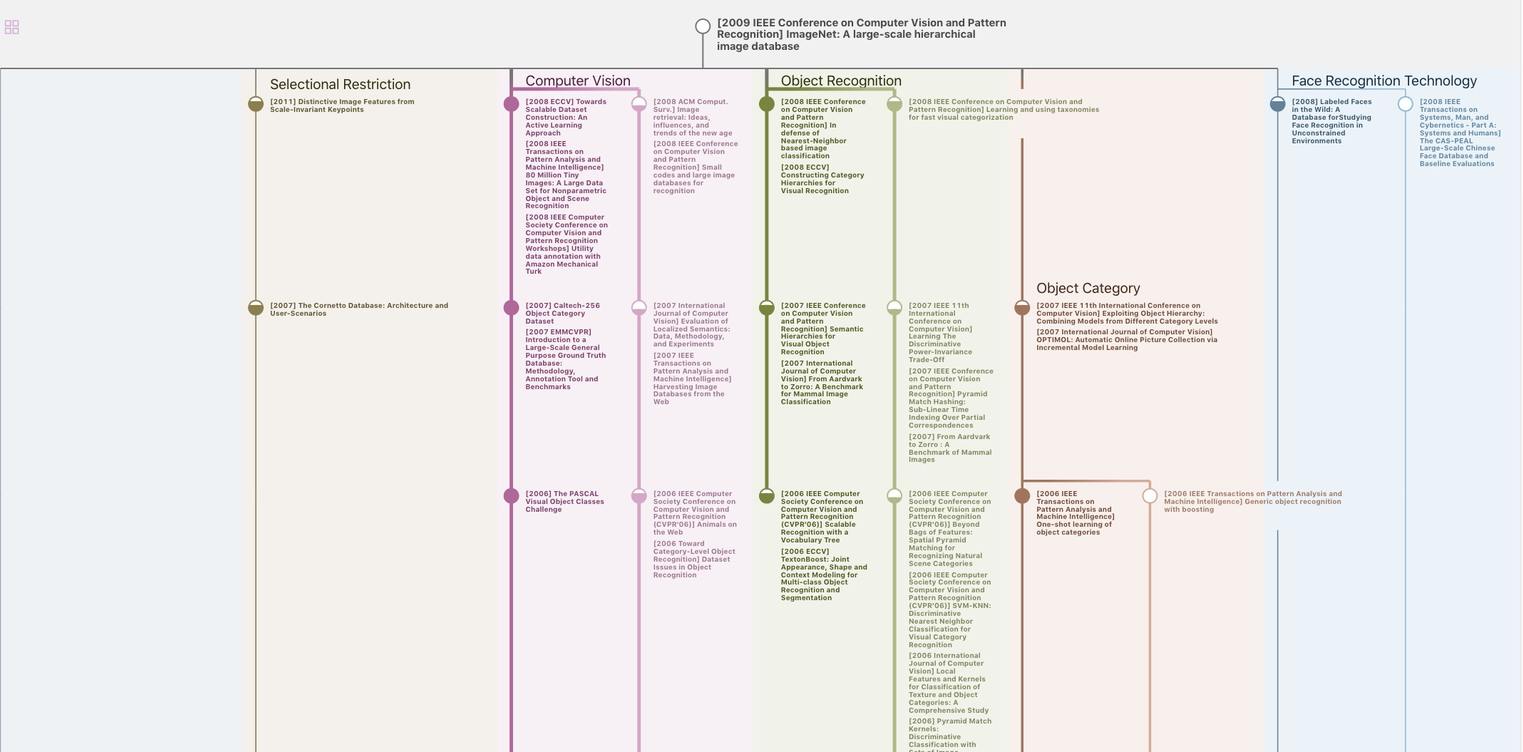
生成溯源树,研究论文发展脉络
Chat Paper
正在生成论文摘要