Unifying Token- and Span-level Supervisions for Few-shot Sequence Labeling
ACM TRANSACTIONS ON INFORMATION SYSTEMS(2024)
摘要
Few-shot sequence labeling aims to identify novel classes based on only a few labeled samples. Existing methods solve the data scarcity problem mainly by designing token-level or span-level labeling models based on metric learning. However, these methods are only trained at a single granularity (i.e., either token-level or span-level) and have some weaknesses of the corresponding granularity. In this article, we first unify token- and span-level supervisions and propose a Consistent Dual Adaptive Prototypical (CDAP) network for few-shot sequence labeling. CDAP contains the token- and span-level networks, jointly trained at different granularities. To align the outputs of two networks, we further propose a consistent loss to enable them to learn from each other. During the inference phase, we propose a consistent greedy inference algorithm that first adjusts the predicted probability and then greedily selects non-overlapping spans with maximum probability. Extensive experiments show that our model achieves new state-of-the-art results on three benchmark datasets. All the code and data of this work will be released at https://github.com/zifengcheng/CDAP.
更多查看译文
关键词
Few-shot sequence labeling,few-shot learning,sequence labeling
AI 理解论文
溯源树
样例
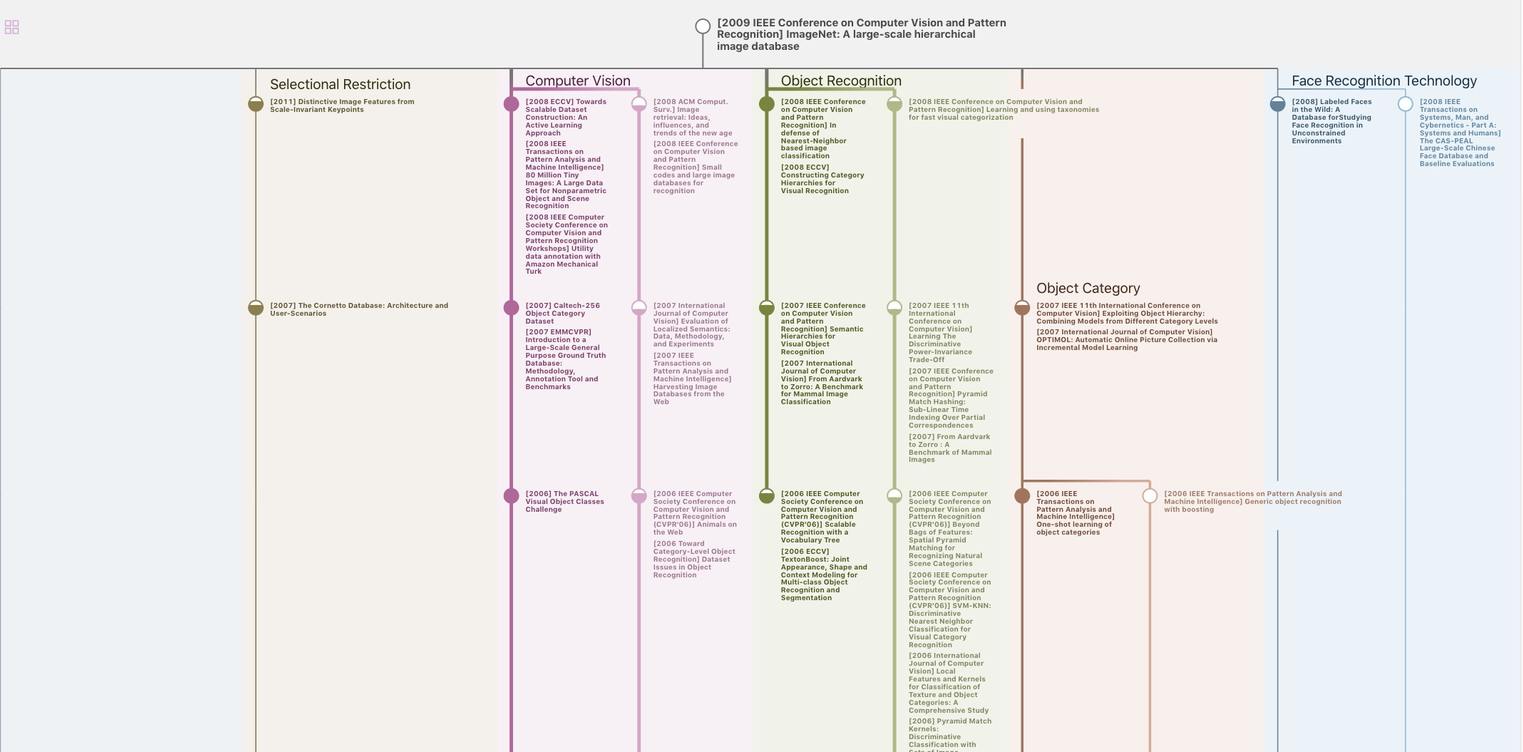
生成溯源树,研究论文发展脉络
Chat Paper
正在生成论文摘要