On the Forward Invariance of Neural ODEs
ICML 2023(2023)
摘要
We propose a new method to ensure neural ordinary differential equations (ODEs) satisfy output specifications by using invariance set propagation. Our approach uses a class of control barrier functions to transform output specifications into constraints on the parameters and inputs of the learning system. This setup allows us to achieve output specification guarantees simply by changing the constrained parameters/inputs both during training and inference. Moreover, we demonstrate that our invariance set propagation through data-controlled neural ODEs not only maintains generalization performance but also creates an additional degree of robustness by enabling causal manipulation of the system's parameters/inputs. We test our method on a series of representation learning tasks, including modeling physical dynamics and convexity portraits, as well as safe collision avoidance for autonomous vehicles.
更多查看译文
关键词
forward invariance,neural
AI 理解论文
溯源树
样例
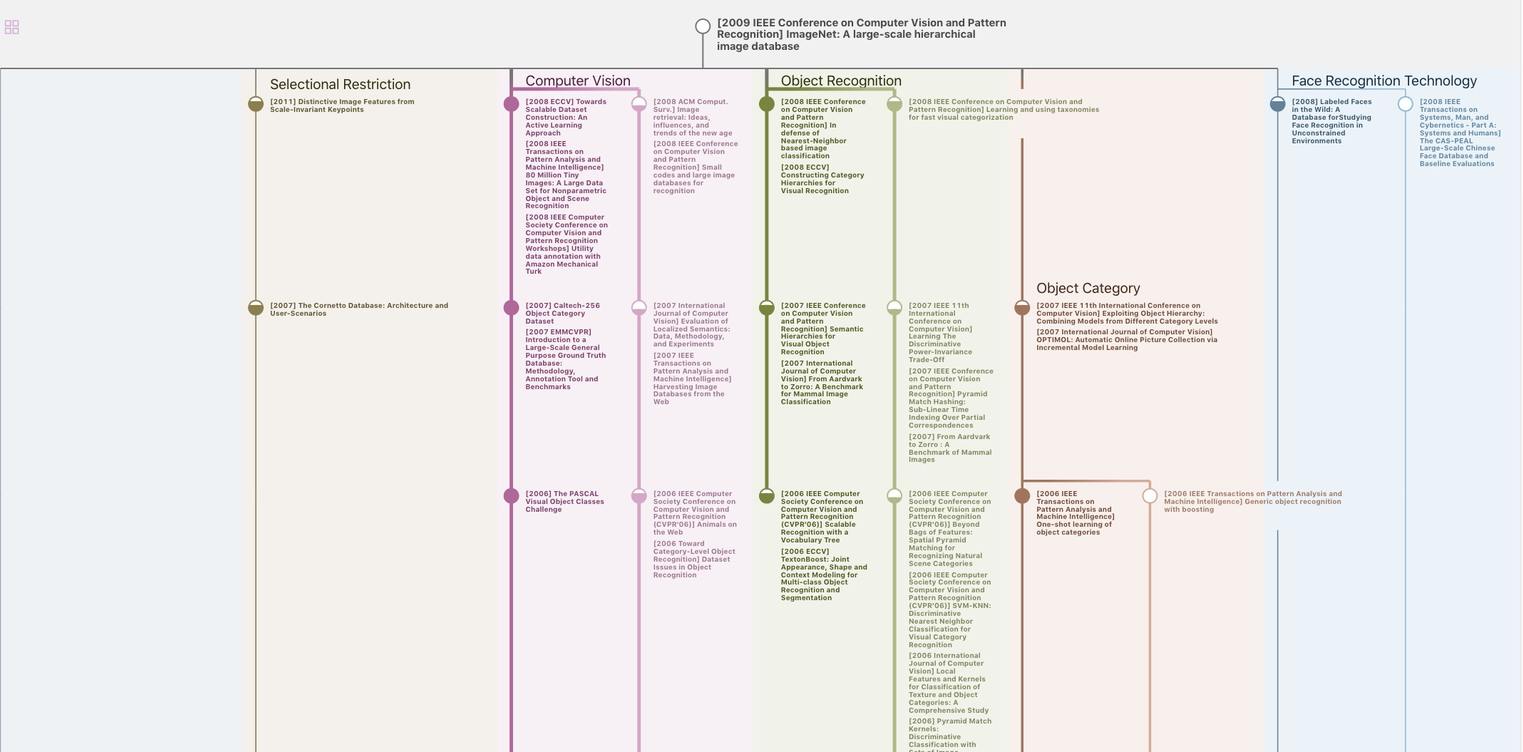
生成溯源树,研究论文发展脉络
Chat Paper
正在生成论文摘要