Leveraging Implicit Feedback from Deployment Data in Dialogue
arXiv (Cornell University)(2023)
摘要
We study improving social conversational agents by learning from natural dialogue between users and a deployed model, without extra annotations. To implicitly measure the quality of a machine-generated utterance, we leverage signals like user response length, sentiment and reaction of the future human utterances in the collected dialogue episodes. Our experiments use the publicly released deployment data from BlenderBot (Xu et al., 2023). Human evaluation indicates improvements in our new models over baseline responses; however, we find that some proxy signals can lead to more generations with undesirable properties as well. For example, optimizing for conversation length can lead to more controversial or unfriendly generations compared to the baseline, whereas optimizing for positive sentiment or reaction can decrease these behaviors.
更多查看译文
关键词
dialogue,deployment data,implicit feedback
AI 理解论文
溯源树
样例
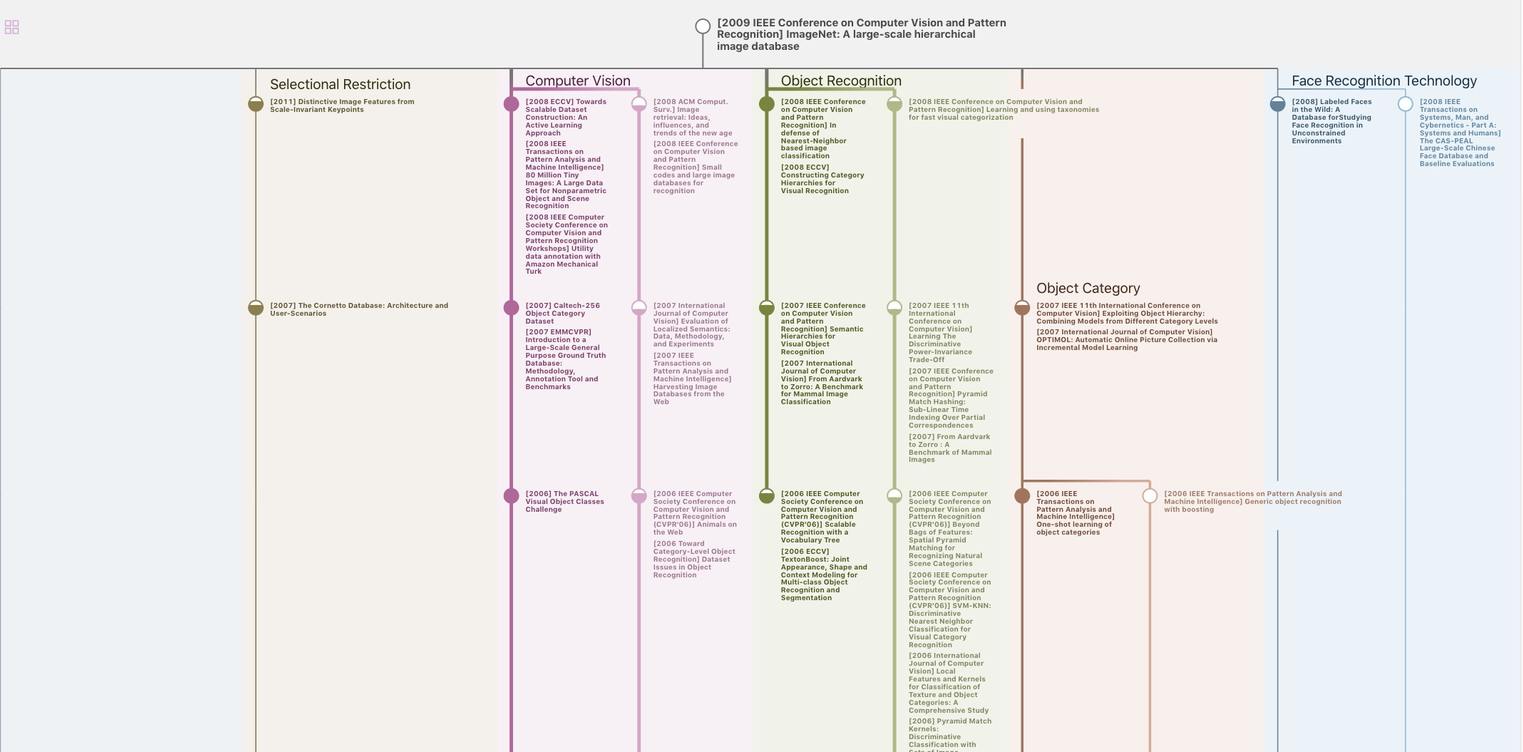
生成溯源树,研究论文发展脉络
Chat Paper
正在生成论文摘要