An accelerated randomized Bregman-Kaczmarz method for strongly convex linearly constraint optimization
2023 EUROPEAN CONTROL CONFERENCE, ECC(2023)
摘要
In this paper we propose a randomized accelerated method for the minimization of a strongly convex function under linear constraints. The method is of Kaczmarz-type, i.e. it only uses a single linear equation in each iteration. To obtain acceleration we build on the fact that the Kaczmarz method is dual to a coordinate descent method. We use a recently proposed acceleration method for the randomized coordinate descent and transfer it to the primal space. This method inherits many of the attractive features of the accelerated coordinate descent method, including its worst-case convergence rates. Theoretical analysis of the convergence of the proposed method is given. Numerical experiments show that the proposed method is more efficient and faster than the existing methods for solving the same problem.
更多查看译文
AI 理解论文
溯源树
样例
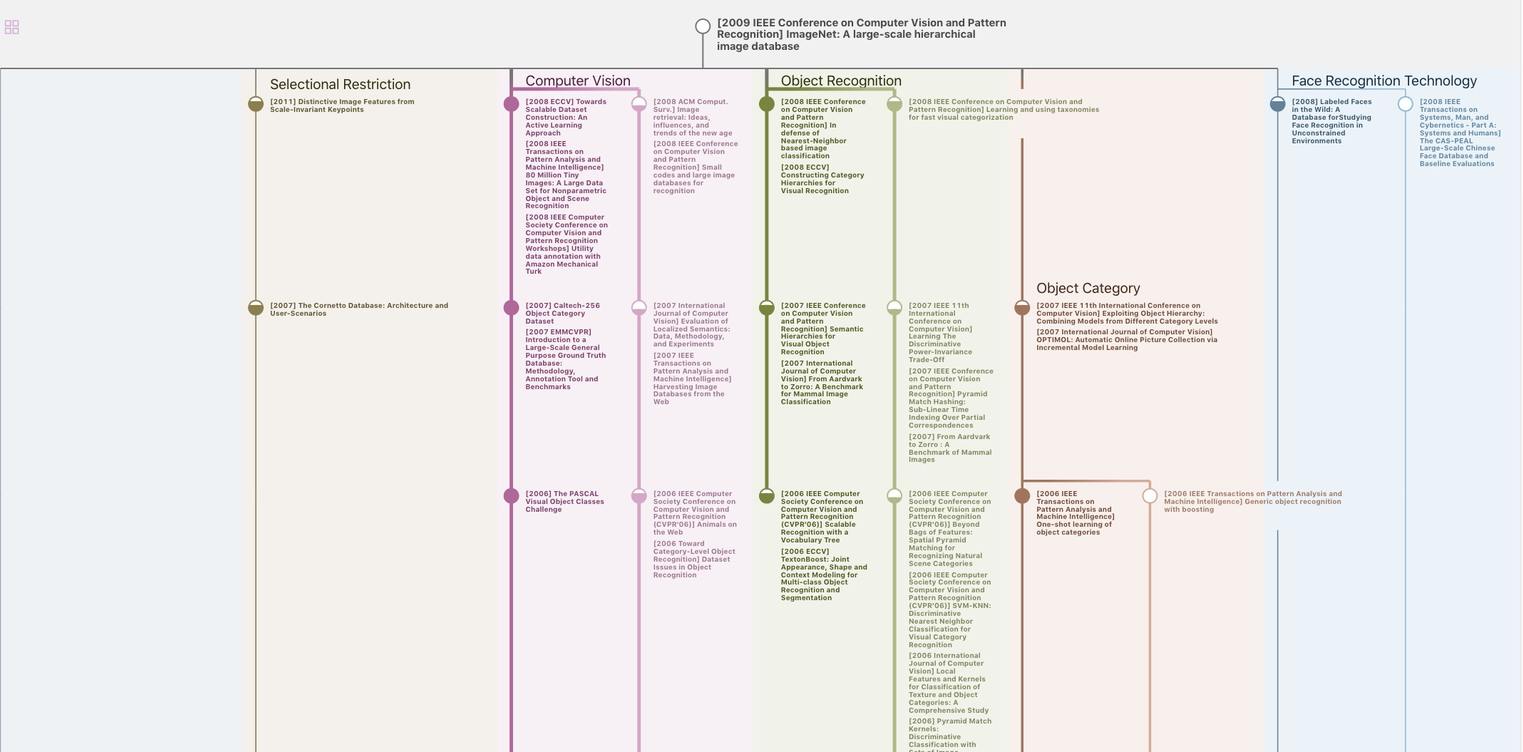
生成溯源树,研究论文发展脉络
Chat Paper
正在生成论文摘要