ROI-demand Traffic Prediction: A Pre-train, Query and Fine-tune Framework.
ICDE(2023)
摘要
Traffic prediction has drawn increasing attention due to its essential role in smart city applications. To achieve precise predictions, a large number of approaches have been proposed to model spatial dependencies and temporal dynamics. Despite their superior performance, most existing studies focus datasets that are usually in large geographic scales, e.g., citywide, while ignoring the results on specific regions. However, in many scenarios, for example, route planning on time-dependent road networks, only small regions are of interest. We name the task of answering forecasting requests from any query region of interest (ROI) as ROI-demand traffic prediction (RTP). In this paper, we make a primary observation that existing methods fail to jointly achieve effectiveness and efficiency for RTP. To address this issue, a novel model-agnostic framework based on pre-Training, Querying and fine-Tuning, named TQT, is proposed, which first customizes input data given an ROI, and then makes fast adaptation from pre-trained traffic prediction backbone models by fine-tuning. We evaluate TQT on two real-world traffic datasets, performing both flow and speed prediction tasks. Extensive experiment results demonstrate the effectiveness and efficiency of the proposed method.
更多查看译文
关键词
traffic forecasting,time series forecasting,pre-train,fine-tune
AI 理解论文
溯源树
样例
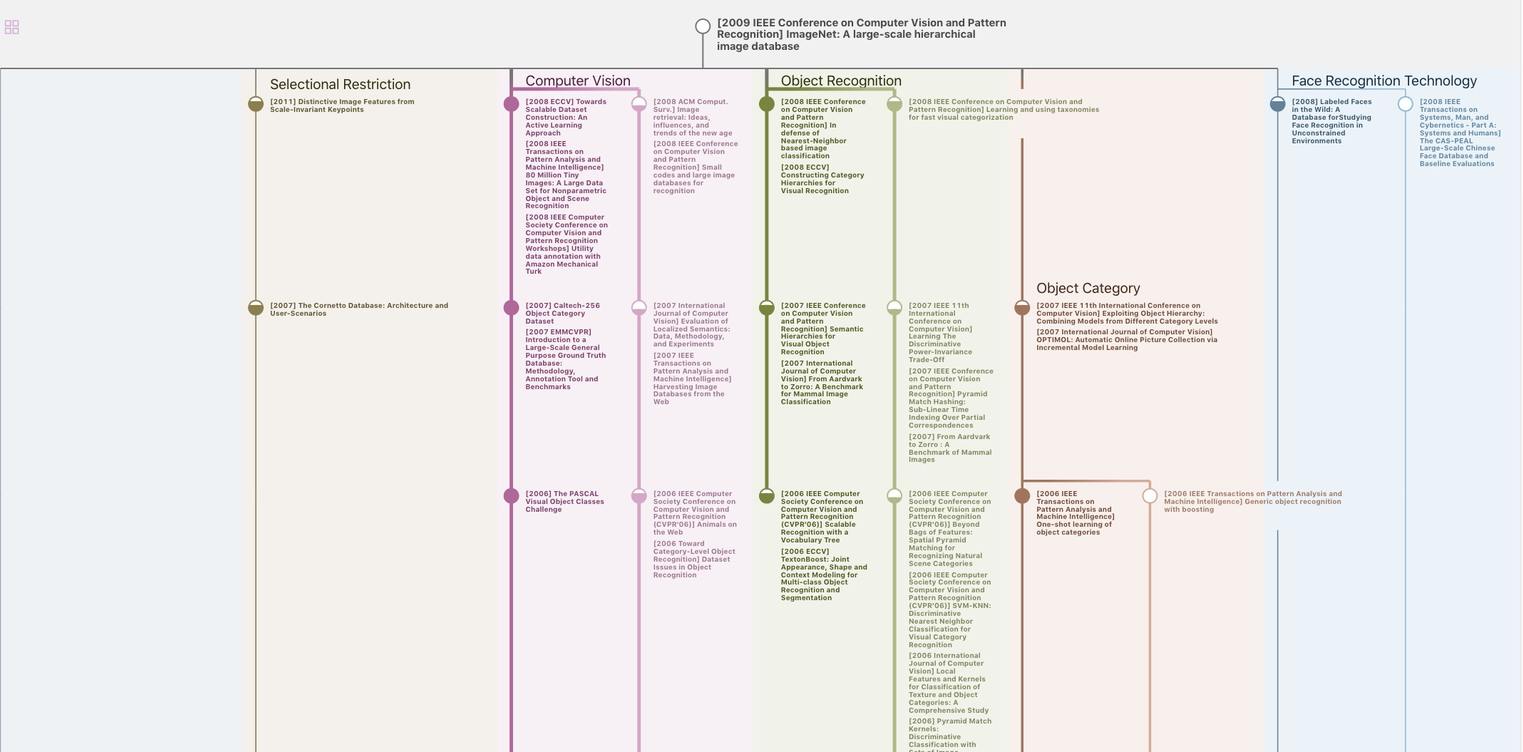
生成溯源树,研究论文发展脉络
Chat Paper
正在生成论文摘要