Compressed Private Aggregation for Scalable and Robust Federated Learning over Massive Networks
CoRR(2023)
摘要
Federated learning (FL) is an emerging paradigm that allows a central server to train machine learning models using remote users' data. Despite its growing popularity, FL faces challenges in preserving the privacy of local datasets, its sensitivity to poisoning attacks by malicious users, and its communication overhead. The latter is additionally considerably dominant in large-scale networks. These limitations are often individually mitigated by local differential privacy (LDP) mechanisms, robust aggregation, compression, and user selection techniques, which typically come at the cost of accuracy. In this work, we present compressed private aggregation (CPA), that allows massive deployments to simultaneously communicate at extremely low bit rates while achieving privacy, anonymity, and resilience to malicious users. CPA randomizes a codebook for compressing the data into a few bits using nested lattice quantizers, while ensuring anonymity and robustness, with a subsequent perturbation to hold LDP. The proposed CPA is proven to result in FL convergence in the same asymptotic rate as FL without privacy, compression, and robustness considerations, while satisfying both anonymity and LDP requirements. These analytical properties are empirically confirmed in a numerical study, where we demonstrate the performance gains of CPA compared with separate mechanisms for compression and privacy for training different image classification models, as well as its robustness in mitigating the harmful effects of malicious users.
更多查看译文
关键词
robust federated
AI 理解论文
溯源树
样例
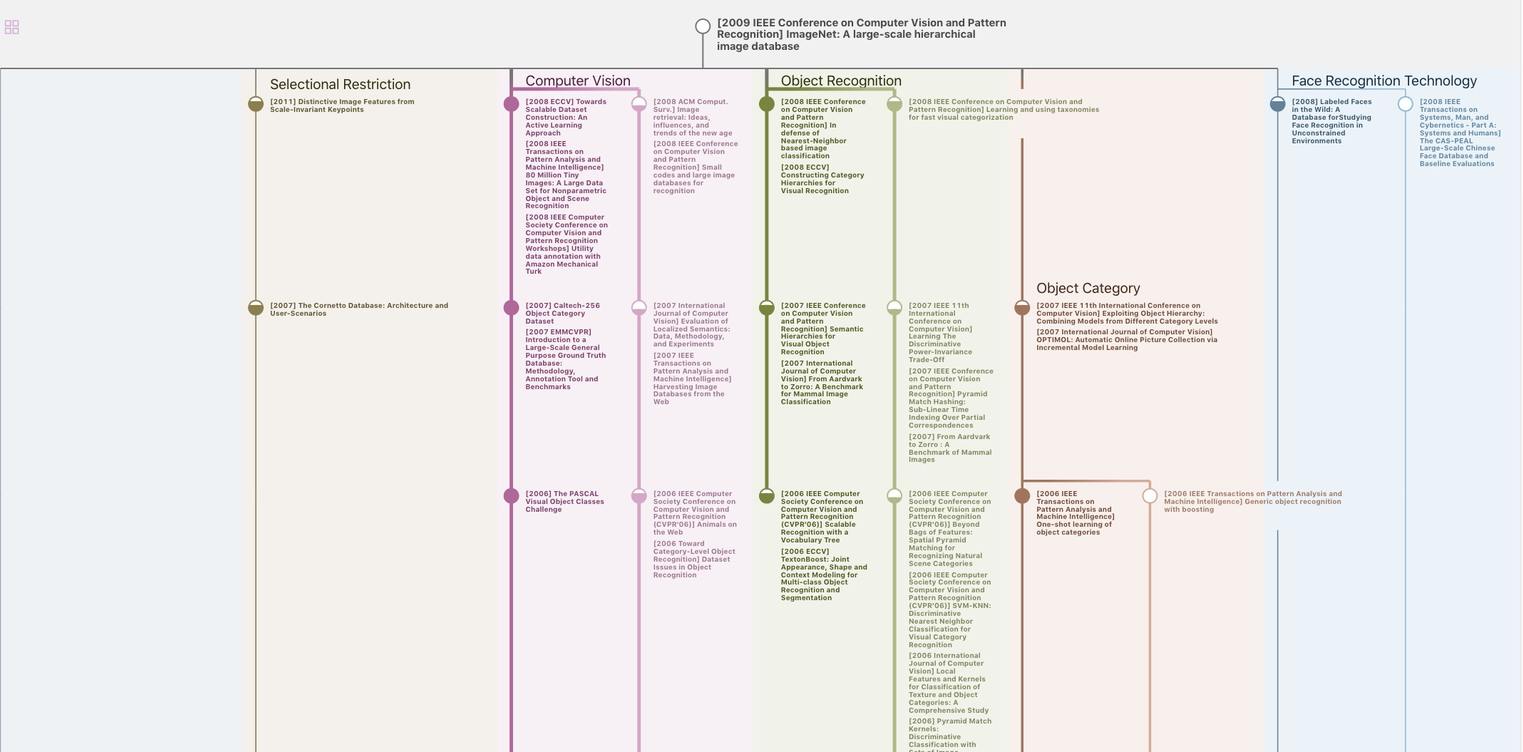
生成溯源树,研究论文发展脉络
Chat Paper
正在生成论文摘要