Lightweight Pixel Difference Networks for Efficient Visual Representation Learning
IEEE TRANSACTIONS ON PATTERN ANALYSIS AND MACHINE INTELLIGENCE(2023)
摘要
Recently, there have been tremendous efforts in developing lightweight Deep Neural Networks (DNNs) with satisfactory accuracy, which can enable the ubiquitous deployment of DNNs in edge devices. The core challenge of developing compact and efficient DNNs lies in how to balance the competing goals of achieving high accuracy and high efficiency. In this paper we propose two novel types of convolutions, dubbed Pixel Difference Convolution (PDC) and Binary PDC (Bi-PDC) which enjoy the following benefits: capturing higher-order local differential information, computationally efficient, and able to be integrated with existing DNNs. With PDC and Bi-PDC, we further present two lightweight deep networks named Pixel Difference Networks (PiDiNet) and Binary PiDiNet (Bi-PiDiNet) respectively to learn highly efficient yet more accurate representations for visual tasks including edge detection and object recognition. Extensive experiments on popular datasets (BSDS500, ImageNet, LFW, YTF, etc.) show that PiDiNet and Bi-PiDiNet achieve the best accuracy-efficiency trade-off. For edge detection, PiDiNet is the first network that can be trained without ImageNet, and can achieve the human-level performance on BSDS500 at 100 FPS and with <1 M parameters. For object recognition, among existing Binary DNNs, Bi-PiDiNet achieves the best accuracy and a nearly 2x reduction of computational cost on ResNet18.
更多查看译文
关键词
Binary neural networks,convolutional neural networks,edge detection,efficient representation learning,facial recognition,image classification
AI 理解论文
溯源树
样例
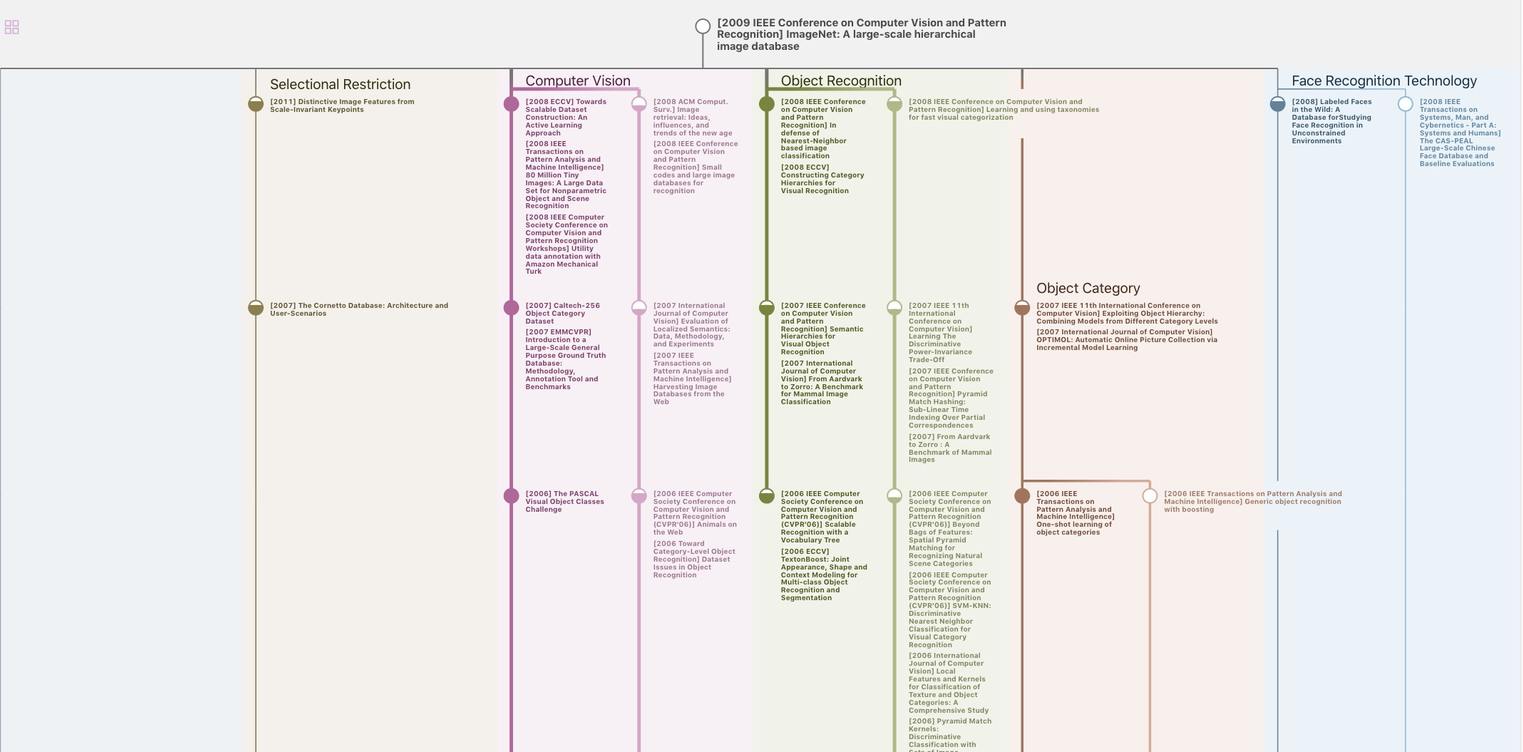
生成溯源树,研究论文发展脉络
Chat Paper
正在生成论文摘要