A Combined Convex Model for Travel Demand Forecasting with Hierarchical Extended Logit Model
arXiv (Cornell University)(2023)
摘要
The travel demand forecasting model plays a crucial role in evaluating large-scale infrastructure projects, such as the construction of new roads or transit lines. While combined modeling approaches have been explored as a solution to overcome the problem of input and output discrepancies in a sequential four-step modeling process, previous attempts at combined models have encountered challenges in real-world applications, primarily due to their limited behavioral richness or computational tractability. In this study, we propose a novel convex programming approach and present a key theorem demonstrating that the optimal solution is the same as the one from solving the hierarchical extended logit model. This model is specifically designed to capture correlations existing in travelers' choices, including similarities among transport modes and route overlaps. The convex property of our model ensures the existence and uniqueness of solutions and offers computational efficiency. The advantages of our proposed model are twofold. Firstly, it provides a single unifying rationale (i.e., utility maximization) that is valid across all steps. Secondly, its combined nature allows a systematic approach to handling observed data, enabling a more realistic representation of reality. By addressing the limited behavior richness of previous combined models, our convex programming-based approach shows promise in enhancing the accuracy and applicability of travel demand forecasting, thereby aiding in the planning and decision-making processes for infrastructure projects.
更多查看译文
关键词
travel demand forecasting,combined convex model
AI 理解论文
溯源树
样例
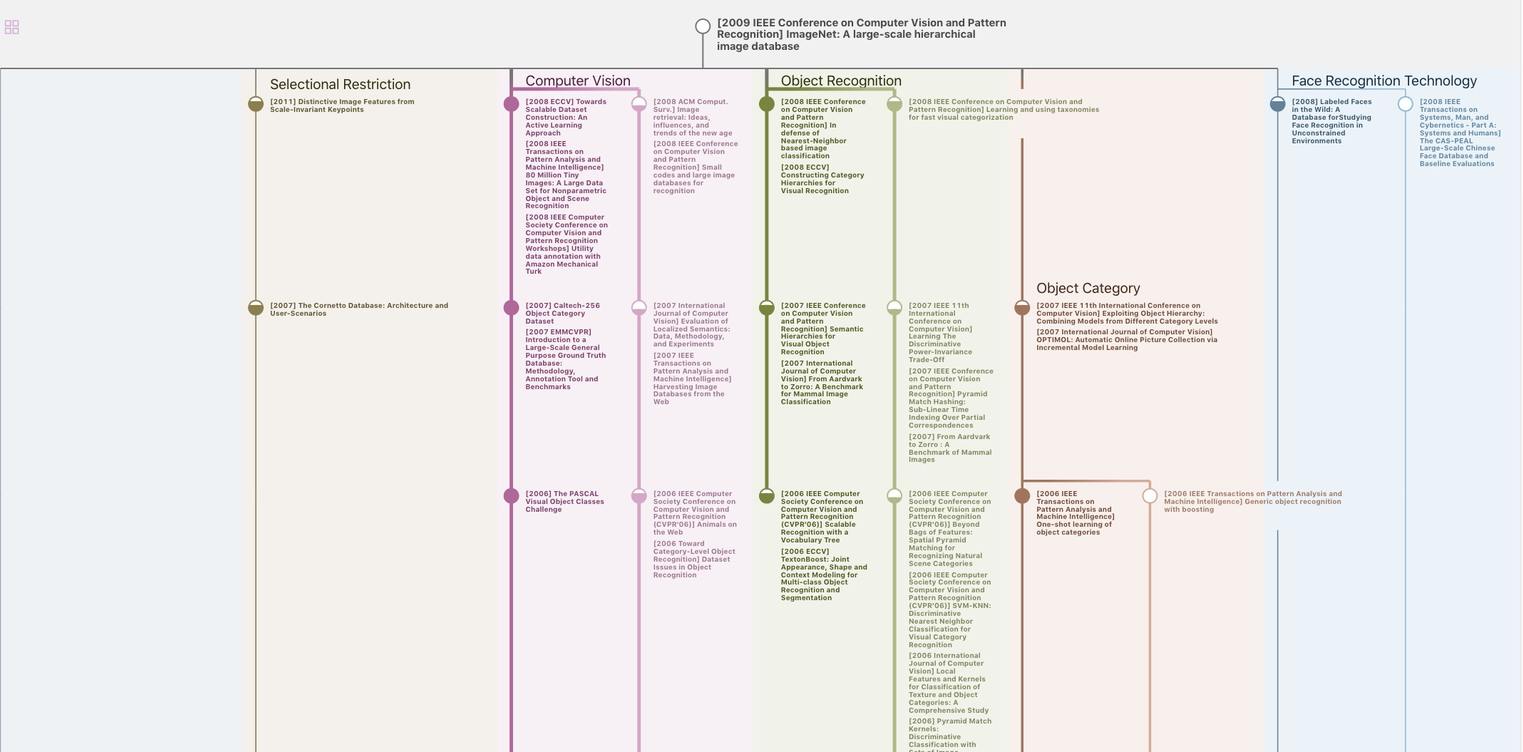
生成溯源树,研究论文发展脉络
Chat Paper
正在生成论文摘要