Bringing Chemistry to Scale: Loss Weight Adjustment for Multivariate Regression in Deep Learning of Thermochemical Processes
CoRR(2023)
摘要
Flamelet models are widely used in computational fluid dynamics to simulate thermochemical processes in turbulent combustion. These models typically employ memory-expensive lookup tables that are predetermined and represent the combustion process to be simulated. Artificial neural networks (ANNs) offer a deep learning approach that can store this tabular data using a small number of network weights, potentially reducing the memory demands of complex simulations by orders of magnitude. However, ANNs with standard training losses often struggle with underrepresented targets in multivariate regression tasks, e.g., when learning minor species mass fractions as part of lookup tables. This paper seeks to improve the accuracy of an ANN when learning multiple species mass fractions of a hydrogen (\ce{H2}) combustion lookup table. We assess a simple, yet effective loss weight adjustment that outperforms the standard mean-squared error optimization and enables accurate learning of all species mass fractions, even of minor species where the standard optimization completely fails. Furthermore, we find that the loss weight adjustment leads to more balanced gradients in the network training, which explains its effectiveness.
更多查看译文
关键词
deep learning,chemistry,regression,loss weight adjustment
AI 理解论文
溯源树
样例
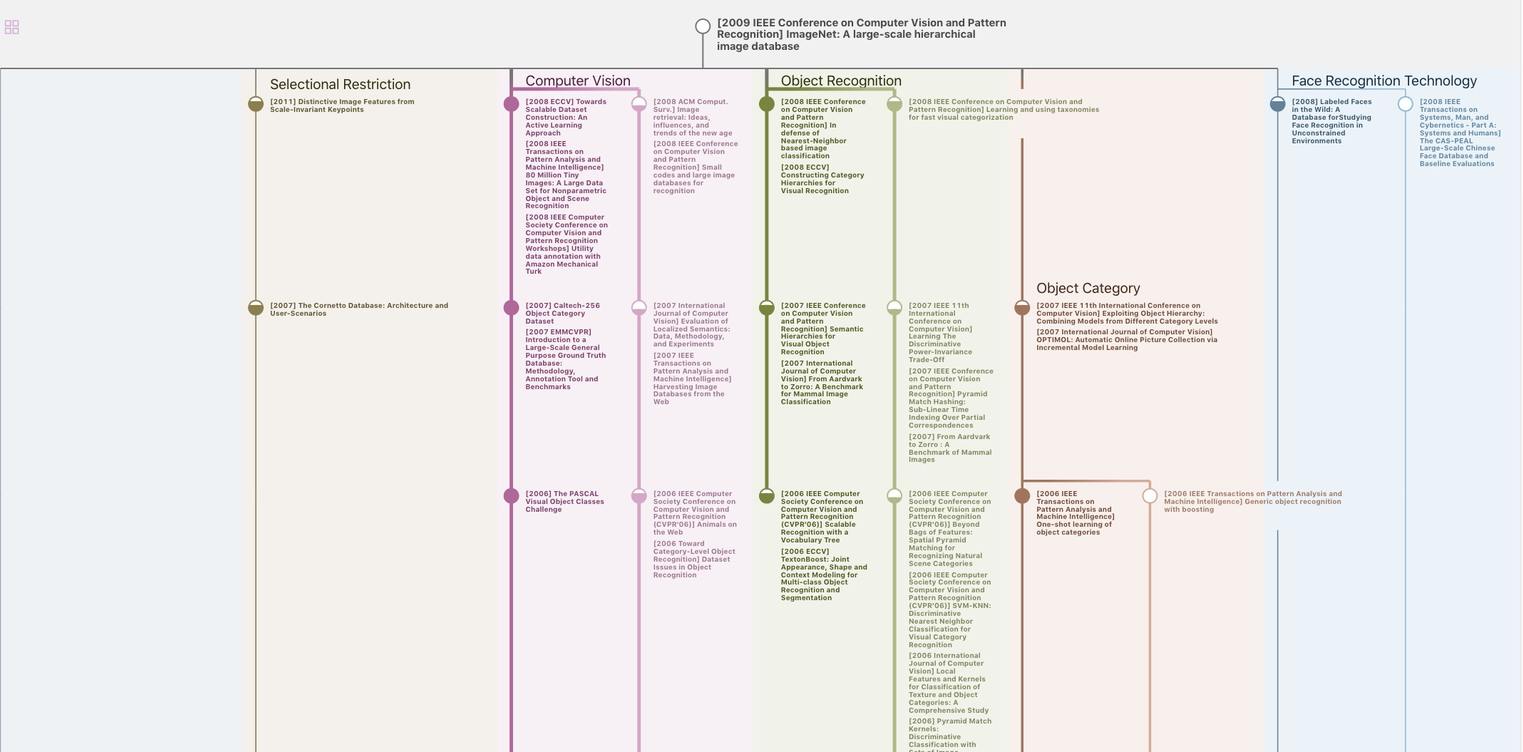
生成溯源树,研究论文发展脉络
Chat Paper
正在生成论文摘要